


Using CSV as an IO tool for read and write operations in Python data processing pandas
Preface
pandas
IO
API
It is a set of top-level reader
functions, such as pandas.read_csv()
, which will return a pandas
object.
The corresponding writer
function is an object method, such as DataFrame.to_csv()
.
Note: StringIO
will be used later, please make sure to import
# python3 from io import StringIO # python2 from StringIO import StringIO
1 CSV and text files
The main function to read text files isread_csv()
1 Parameter analysis
read_csv()
Accepts the following common parameters:
1.1 Basics
filepath_or_buffer
: The variable
can be a file path, file
URL
or anything with theread()
function Object
sep
: str
, default ,
, for read_table
it is \t
File delimiter, if set to
None
, theC
engine cannot automatically detect the delimiter, andThe Python
engine can automatically detect delimiters through the built-in sniffer tool.In addition, if the set character length is greater than
1
and is not'\s '
, then the string will be parsed as a regular expression, and forces the use of thePython
parsing engine.For example
'\\r\\t'
, but regular expressions tend to ignore reference data in the text.
delimiter
: str
, default is None
# Alternative parameters for ##sep
, the functions are the same
header:
int or
list, defaults to
'infer'
- The line number used as the column name, the default behavior is to infer the column name:
- If the
names
parameter is not specified the behavior is similar to
header=0, i.e. starting from the first line read .
- If
names
is set, the behavior is the same as
header=None.
- You can also set a list for
header
to represent multi-level column names. For example,
[0,1,3], unspecified lines (here
2) will be skipped. If
skip_blank_lines=True, they will be skipped. Pass blank lines and commented lines. Therefore
header=0does not represent the first line of the file
names:
array-like, the default is
None
- The list of column names that need to be set. If the file does not contain a header row,
header=None
should be passed explicitly, and Duplicate values are not allowed in this list.
index_col:
int,
str,
sequence of int/str,
False, defaults to
None
- The column used as the index of
DataFrame
, which can be in the form of a string name or column index given. If a list is specified, use
MultiIndex - Note:
index_col=False
can be used to force
pandasnot to One column is used as an index. For example, when your file is a bad file with a delimiter at the end of each line.
usecols: List or function, default is
None
- Read only the specified List. If a list, all elements must be positional (i.e., integer indices into the file's columns) or strings that match the column names supplied with the
names
parameter or inferred from the document header row. correspond.
- The order in the list will be ignored, that is,
usecols=[0, 1]
is equivalent to
[1, 0] - If it is a callable function, it will be calculated based on the column name and the name of the callable function calculated as
True
In [1]: import pandas as pd In [2]: from io import StringIO In [3]: data = "col1,col2,col3\na,b,1\na,b,2\nc,d,3" In [4]: pd.read_csv(StringIO(data)) Out[4]: col1 col2 col3 0 a b 1 1 a b 2 2 c d 3 In [5]: pd.read_csv(StringIO(data), usecols=lambda x: x.upper() in ["COL1", "COL3"]) Out[5]: col1 col3 0 a 1 1 a 2 2 c 3
squeeze:
boolean, default is
False
- If the parsed data contains only one column, then return a
Series
: str
, defaults to None
- 'X'
- means
X0
,
X1...
mangle_dupe_cols
boolean, default is
True
重复的列将被指定为
'X'
,'X.1'
…'X.N'
,而不是'X'
... 。如果在列中有重复的名称,传递False
将导致数据被覆盖
1.3 常规解析配置
dtype
: 类型名或类型字典(column -> type
), 默认为 None
数据或列的数据类型。例如。
{'a':np.float64,'b':np.int32}
engine
: {'c', 'python'}
要使用的解析器引擎。
C
引擎更快,而Python
引擎目前功能更完整
converters
: dict
, 默认为 None
用于在某些列中对值进行转换的函数字典。键可以是整数,也可以是列名
true_values
: list
, 默认为 None
数据值解析为
True
false_values
: list
, 默认为 None
数据值解析为
False
skipinitialspace
: boolean
, 默认为 False
跳过分隔符之后的空格
skiprows
: 整数或整数列表, 默认为 None
在文件开头要跳过的行号(索引为
0
)或要跳过的行数如果可调用函数,则对索引应用函数,如果返回
True
,则应跳过该行,否则返回False
In [6]: data = "col1,col2,col3\na,b,1\na,b,2\nc,d,3" In [7]: pd.read_csv(StringIO(data)) Out[7]: col1 col2 col3 0 a b 1 1 a b 2 2 c d 3 In [8]: pd.read_csv(StringIO(data), skiprows=lambda x: x % 2 != 0) Out[8]: col1 col2 col3 0 a b 2
skipfooter
: int
, 默认为 0
需要跳过文件末尾的行数(不支持
C
引擎)
nrows
: int
, 默认为 None
要读取的文件行数,对于读取大文件很有用
memory_map
: boolean
, 默认为 False
如果为
filepath_or_buffer
参数指定了文件路径,则将文件对象直接映射到内存中,然后直接从那里访问数据。使用此选项可以提高性能,因为不再有任何I/O
开销
1.4 NA 和缺失数据处理
na_values
: scalar
, str
, list-like
, dict
, 默认为 None
需要转换为
NA
值的字符串
keep_default_na
: boolean
, 默认为 True
解析数据时是否包含默认的
NaN
值。根据是否传入na_values
,其行为如下keep_default_na=True
, 且指定了na_values
,na_values
将会与默认的NaN
一起被解析keep_default_na=True
, 且未指定na_values
, 只解析默认的NaN
keep_default_na=False
, 且指定了na_values
, 只解析na_values
指定的NaN
keep_default_na=False
, 且未指定na_values
, 字符串不会被解析为NaN
注意:如果 na_filter=False
,那么 keep_default_na
和 na_values
参数将被忽略
na_filter
: boolean
, 默认为 True
检测缺失值标记(空字符串和
na_values
的值)。在没有任何NA
的数据中,设置na_filter=False
可以提高读取大文件的性能
skip_blank_lines
: boolean
, 默认为 True
如果为
True
,则跳过空行,而不是解释为NaN
值
1.5 日期时间处理
parse_dates
: 布尔值、列表或嵌套列表、字典, 默认为 False
.
如果为
True
-> 尝试解析索引如果为
[1, 2, 3]
-> 尝试将1, 2, 3
列解析为分隔的日期如果为
[[1, 3]]
-> 将1, 3
列解析为单个日期列如果为
{'foo': [1, 3]}
-> 将1, 3
列作为日期并设置列名为foo
infer_datetime_format
: 布尔值, 默认为 False
如果设置为
True
且设置了parse_dates
,则尝试推断datetime
格式以加快处理速度
date_parser
: 函数, 默认为 None
用于将字符串序列转换为日期时间实例数组的函数。默认使用
dateutil.parser.parser
进行转换,pandas
将尝试以三种不同的方式调用date_parser
传递一个或多个数组(
parse_dates
定义的列)作为参数;将
parse_dates
定义的列中的字符串值连接到单个数组中,并将其传递;使用一个或多个字符串(对应于
parse_dates
定义的列)作为参数,对每一行调用date_parser
一次。
dayfirst
: 布尔值, 默认为 False
DD/MM
格式的日期
cache_dates
: 布尔值, 默认为 True
如果为
True
,则使用唯一的、经过转换的日期缓存来应用datetime
转换。在解析重复的日期字符串,特别是带有时区偏移量的日期字符串时,可能会显著提高速度。
1.6 迭代
iterator
: boolean
, 默认为 False
返回
TextFileReader
对象以进行迭代或使用get_chunk()
来获取块
1.7 引用、压缩和文件格式
compression
: {'infer', 'gzip', 'bz2', 'zip', 'xz', None, dict}
, 默认为 'infer'
用于对磁盘数据进行即时解压缩。如果为
"infer"
,则如果filepath_or_buffer
是文件路径且以".gz"
,".bz2"
,".zip"
或".xz"
结尾,则分别使用gzip
,bz2
,zip
或xz
解压,否则不进行解压缩。如果使用
"zip"
,则ZIP
文件必须仅包含一个要读取的数据文件。设置为None
表示不解压也可以使用字典的方式,键为
method
的值从{'zip', 'gzip', 'bz2'}
中选择。例如
compression={'method': 'gzip', 'compresslevel': 1, 'mtime': 1}
thousandsstr
, 默认为 None
数值在千位的分隔符
decimal
: str
, 默认为 '.'
小数点
float_precision
: string
, 默认为 None
指定
C
引擎应该使用哪个转换器来处理浮点值。普通转换器的选项为None
,高精度转换器的选项为high
,双向转换器的选项为round_trip
。
quotechar
: str
(长度为 1
)
用于表示被引用数据的开始和结束的字符。带引号的数据里的分隔符将被忽略
comment
: str
, 默认为 None
用于跳过该字符开头的行,例如,如果
comment='#'
,将会跳过#
开头的行
encoding
: str
, 默认为 None
设置编码格式
1.8 错误处理
error_bad_linesboolean
, 默认为 True
默认情况下,字段太多的行(例如,带有太多逗号的
csv
文件)会引发异常,并且不会返回任何DataFrame
。如果设置为
False
,则这些坏行将会被删除
warn_bad_linesboolean
, 默认为 True
如果
error_bad_lines=False
且warn_bad_lines=True
,每个坏行都会输出一个警告
2. 指定数据列的类型
您可以指示整个 DataFrame
或各列的数据类型
In [9]: import numpy as np In [10]: data = "a,b,c,d\n1,2,3,4\n5,6,7,8\n9,10,11" In [11]: print(data) a,b,c,d 1,2,3,4 5,6,7,8 9,10,11 In [12]: df = pd.read_csv(StringIO(data), dtype=object) In [13]: df Out[13]: a b c d 0 1 2 3 4 1 5 6 7 8 2 9 10 11 NaN In [14]: df["a"][0] Out[14]: '1' In [15]: df = pd.read_csv(StringIO(data), dtype={"b": object, "c": np.float64, "d": "Int64"}) In [16]: df.dtypes Out[16]: a int64 b object c float64 d Int64 dtype: object
你可以使用 read_csv()
的 converters
参数,统一某列的数据类型
In [17]: data = "col_1\n1\n2\n'A'\n4.22" In [18]: df = pd.read_csv(StringIO(data), converters={"col_1": str}) In [19]: df Out[19]: col_1 0 1 1 2 2 'A' 3 4.22 In [20]: df["col_1"].apply(type).value_counts() Out[20]: <class 'str'> 4 Name: col_1, dtype: int64
或者,您可以在读取数据后使用 to_numeric()
函数强制转换类型
In [21]: df2 = pd.read_csv(StringIO(data)) In [22]: df2["col_1"] = pd.to_numeric(df2["col_1"], errors="coerce") In [23]: df2 Out[23]: col_1 0 1.00 1 2.00 2 NaN 3 4.22 In [24]: df2["col_1"].apply(type).value_counts() Out[24]: <class 'float'> 4 Name: col_1, dtype: int64
它将所有有效的数值转换为浮点数,而将无效的解析为 NaN
最后,如何处理包含混合类型的列取决于你的具体需要。在上面的例子中,如果您只想要将异常的数据转换为 NaN
,那么 to_numeric()
可能是您的最佳选择。
然而,如果您想要强制转换所有数据,而无论类型如何,那么使用 read_csv()
的 converters
参数会更好
注意
在某些情况下,读取包含混合类型列的异常数据将导致数据集不一致。
如果您依赖 pandas
来推断列的类型,解析引擎将继续推断数据块的类型,而不是一次推断整个数据集。
In [25]: col_1 = list(range(500000)) + ["a", "b"] + list(range(500000)) In [26]: df = pd.DataFrame({"col_1": col_1}) In [27]: df.to_csv("foo.csv") In [28]: mixed_df = pd.read_csv("foo.csv") In [29]: mixed_df["col_1"].apply(type).value_counts() Out[29]: <class 'int'> 737858 <class 'str'> 262144 Name: col_1, dtype: int64 In [30]: mixed_df["col_1"].dtype Out[30]: dtype('O')
这就导致 mixed_df
对于列的某些块包含 int
类型,而对于其他块则包含 str
,这是由于读取的数据是混合类型。
The above is the detailed content of Using CSV as an IO tool for read and write operations in Python data processing pandas. For more information, please follow other related articles on the PHP Chinese website!

Hot AI Tools

Undresser.AI Undress
AI-powered app for creating realistic nude photos

AI Clothes Remover
Online AI tool for removing clothes from photos.

Undress AI Tool
Undress images for free

Clothoff.io
AI clothes remover

Video Face Swap
Swap faces in any video effortlessly with our completely free AI face swap tool!

Hot Article

Hot Tools

Notepad++7.3.1
Easy-to-use and free code editor

SublimeText3 Chinese version
Chinese version, very easy to use

Zend Studio 13.0.1
Powerful PHP integrated development environment

Dreamweaver CS6
Visual web development tools

SublimeText3 Mac version
God-level code editing software (SublimeText3)

Hot Topics


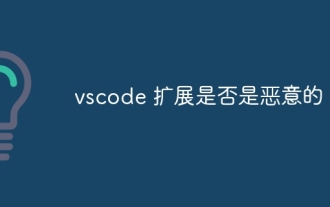
VS Code extensions pose malicious risks, such as hiding malicious code, exploiting vulnerabilities, and masturbating as legitimate extensions. Methods to identify malicious extensions include: checking publishers, reading comments, checking code, and installing with caution. Security measures also include: security awareness, good habits, regular updates and antivirus software.
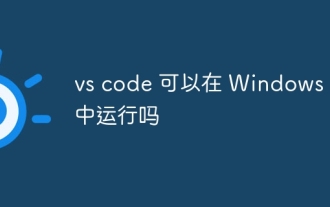
VS Code can run on Windows 8, but the experience may not be great. First make sure the system has been updated to the latest patch, then download the VS Code installation package that matches the system architecture and install it as prompted. After installation, be aware that some extensions may be incompatible with Windows 8 and need to look for alternative extensions or use newer Windows systems in a virtual machine. Install the necessary extensions to check whether they work properly. Although VS Code is feasible on Windows 8, it is recommended to upgrade to a newer Windows system for a better development experience and security.
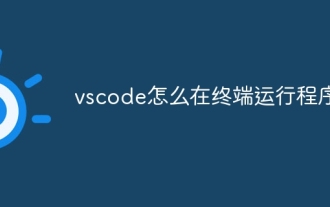
In VS Code, you can run the program in the terminal through the following steps: Prepare the code and open the integrated terminal to ensure that the code directory is consistent with the terminal working directory. Select the run command according to the programming language (such as Python's python your_file_name.py) to check whether it runs successfully and resolve errors. Use the debugger to improve debugging efficiency.
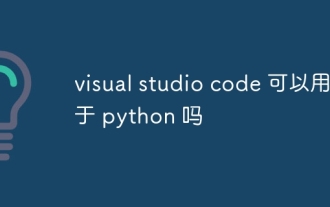
VS Code can be used to write Python and provides many features that make it an ideal tool for developing Python applications. It allows users to: install Python extensions to get functions such as code completion, syntax highlighting, and debugging. Use the debugger to track code step by step, find and fix errors. Integrate Git for version control. Use code formatting tools to maintain code consistency. Use the Linting tool to spot potential problems ahead of time.
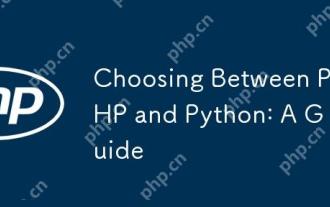
PHP is suitable for web development and rapid prototyping, and Python is suitable for data science and machine learning. 1.PHP is used for dynamic web development, with simple syntax and suitable for rapid development. 2. Python has concise syntax, is suitable for multiple fields, and has a strong library ecosystem.
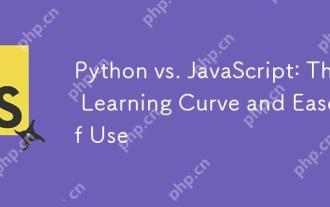
Python is more suitable for beginners, with a smooth learning curve and concise syntax; JavaScript is suitable for front-end development, with a steep learning curve and flexible syntax. 1. Python syntax is intuitive and suitable for data science and back-end development. 2. JavaScript is flexible and widely used in front-end and server-side programming.
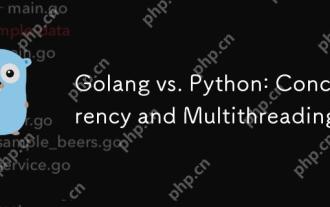
Golang is more suitable for high concurrency tasks, while Python has more advantages in flexibility. 1.Golang efficiently handles concurrency through goroutine and channel. 2. Python relies on threading and asyncio, which is affected by GIL, but provides multiple concurrency methods. The choice should be based on specific needs.
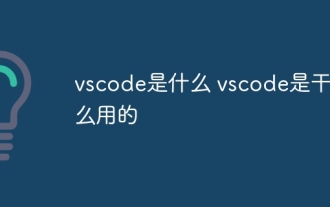
VS Code is the full name Visual Studio Code, which is a free and open source cross-platform code editor and development environment developed by Microsoft. It supports a wide range of programming languages and provides syntax highlighting, code automatic completion, code snippets and smart prompts to improve development efficiency. Through a rich extension ecosystem, users can add extensions to specific needs and languages, such as debuggers, code formatting tools, and Git integrations. VS Code also includes an intuitive debugger that helps quickly find and resolve bugs in your code.
