


YOLOv6's fast and accurate target detection framework has been open sourced
Authors: Chu Yi, Kai Heng, etc.
Recently, Meituan’s Visual Intelligence Department has developed YOLOv6, a target detection framework dedicated to industrial applications, which can focus on detection accuracy and reasoning efficiency at the same time. During the research and development process, the Visual Intelligence Department continued to explore and optimize, while drawing on some cutting-edge developments and scientific research results from academia and industry. Experimental results on COCO, the authoritative target detection data set, show that YOLOv6 surpasses other algorithms of the same size in terms of detection accuracy and speed. It also supports the deployment of a variety of different platforms, greatly simplifying the adaptation work during project deployment. This is open source, hoping to help more students.
1. Overview
YOLOv6 is a target detection framework developed by Meituan’s Visual Intelligence Department and is dedicated to industrial applications. This framework focuses on both detection accuracy and inference efficiency. Among the commonly used size models in the industry: YOLOv6-nano has an accuracy of up to 35.0% AP on COCO and an inference speed of on T4. 1242 FPS; YOLOv6-s can achieve an accuracy of 43.1% AP on COCO, and an inference speed of 520 FPS on T4. In terms of deployment, YOLOv6 supports the deployment of different platforms such as GPU (TensorRT), CPU (OPENVINO), ARM (MNN, TNN, NCNN), which greatly Simplify the adaptation work during project deployment. Currently, the project has been open sourced to Github, portal: YOLOv6. Friends who are in need are welcome to Star to collect it and access it at any time.
A new framework whose accuracy and speed far exceed YOLOv5 and YOLOX
Object detection, as a basic technology in the field of computer vision, has been widely used in the industry , among which the YOLO series algorithms have gradually become the preferred framework for most industrial applications due to their good comprehensive performance. So far, the industry has derived many YOLO detection frameworks, among which YOLOv5[1], YOLOX[2] and PP-YOLOE[3] are the most representative. performance, but in actual use, we found that the above framework still has a lot of room for improvement in terms of speed and accuracy. Based on this, we developed a new target detection framework-YOLOv6 by researching and drawing on existing advanced technologies in the industry. The framework supports the full chain of industrial application requirements such as model training, inference and multi-platform deployment, and has made a number of improvements and optimizations at the algorithm level such as network structure and training strategies. On the COCO data set, YOLOv6 has both accuracy and speed. Surpassing other algorithms of the same size, the relevant results are shown in Figure 1 below:
##Figure 1-1 YOLOv6 model performance of each size and other models Comparison
Figure 1-2 Performance comparison between YOLOv6 and other models at different resolutionsFigure 1-1 shows the performance comparison of each detection algorithm under different size networks. The points on the curve respectively represent the performance of the detection algorithm under different size networks (s /tiny/nano) model performance, as can be seen from the figure, YOLOv6 surpasses other YOLO series algorithms of the same size in terms of accuracy and speed. Figure 1-2 shows the performance comparison of each detection network model when the input resolution changes. The points on the curve from left to right represent when the image resolution increases sequentially (384/448/512/576 /640) The performance of this model, as can be seen from the figure, YOLOv6 still maintains a large performance advantage under different resolutions.
2. Introduction to key technologies of YOLOv6YOLOv6 has made many improvements mainly in Backbone, Neck, Head and training strategies:
- We have designed a more efficient Backbone and Neck in a unified manner: Inspired by the design ideas of hardware-aware neural networks, we designed a reparameterizable and more efficient design based on RepVGG style[4] EfficientRep Backbone and Rep-PAN Neck.
- The more concise and effective Efficient Decoupled Head is optimized and designed to further reduce the additional delay overhead caused by general decoupled heads while maintaining accuracy.
- In terms of training strategy, we adopt the Anchor-free anchor-free paradigm, supplemented by SimOTA[2] label allocation strategy and SIoU[9] Bounding box regression loss to further improve detection accuracy.
2.1 Hardware-friendly backbone network design
The Backbone and Neck used by YOLOv5/YOLOX are both built based on CSPNet[5] , using a multi-branch approach and residual structure. For hardware such as GPUs, this structure will increase latency to a certain extent and reduce memory bandwidth utilization. Figure 2 below is an introduction to the Roofline Model[8] in the field of computer architecture, showing the relationship between computing power and memory bandwidth in hardware.
Figure 2 Roofline Model introduction diagram
So, we based on the idea of hardware-aware neural network design , Backbone and Neck have been redesigned and optimized. This idea is based on the characteristics of the hardware and the characteristics of the inference framework/compilation framework. It takes the hardware and compilation-friendly structure as the design principle. When building the network, it comprehensively considers the hardware computing power, memory bandwidth, compilation optimization characteristics, network representation capabilities, etc., and then Get fast and good network structure. For the above two redesigned detection components, we call them EfficientRep Backbone and Rep-PAN Neck respectively in YOLOv6. Their main contributions are:
- The introduction of RepVGG[4] style structure.
- Backbone and Neck have been redesigned based on hardware-aware thinking.
RepVGG[4] Style structure is a multi-branch topology during training, which can be equivalently fused into a single 3x3 during actual deployment A reparameterizable structure of convolution (The fusion process is shown in Figure 3 below). Through the fused 3x3 convolution structure, the computing power of computationally intensive hardware (such as GPU) can be effectively utilized, and the help of the highly optimized NVIDIA cuDNN and Intel MKL compilation frameworks on GPU/CPU can also be obtained. .
Experiments show that through the above strategy, YOLOv6 reduces the hardware delay and significantly improves the accuracy of the algorithm, making the detection network faster and stronger. Taking the nano-size model as an example, compared with the network structure used by YOLOv5-nano, this method improves the speed by 21% and increases the accuracy by 3.6% AP.
Figure 3 Fusion process of Rep operator[4]
EfficientRep Backbone: In terms of Backbone design, we designed an efficient Backbone based on the above Rep operator. Compared with the CSP-Backbone used by YOLOv5, this Backbone can efficiently utilize the computing power of hardware (such as GPU) and also has strong representation capabilities.
Figure 4 below is the specific design structure diagram of EfficientRep Backbone. We replaced the ordinary Conv layer with stride=2 in Backbone with the RepConv layer with stride=2. At the same time, the original CSP-Block is redesigned into RepBlock, where the first RepConv of RepBlock will transform and align the channel dimension. Additionally, we optimize the original SPPF into a more efficient SimSPPF.
Figure 4 EfficientRep Backbone structure diagram
Rep-PAN: In terms of Neck design, in order to make its reasoning on hardware more efficient and achieve a better balance between accuracy and speed, we designed a more effective feature fusion network structure for YOLOv6 based on the hardware-aware neural network design idea.
Rep-PAN is based on the PAN[6] topology, using RepBlock to replace the CSP-Block used in YOLOv5, and at the same time adjusting the operators in the overall Neck, with the purpose of While achieving efficient inference on the hardware, it maintains good multi-scale feature fusion capabilities (Rep-PAN structure diagram is shown in Figure 5 below).
Figure 5 Rep-PAN structure diagram
2.2 More concise and efficient Decoupled Head
In YOLOv6, we adopt the decoupled detection head (Decoupled Head) structure and streamline its design. The detection head of the original YOLOv5 is implemented by merging and sharing the classification and regression branches, while the detection head of YOLOX decouples the classification and regression branches, and adds two additional 3x3 convolutional layers. Although The detection accuracy is improved, but the network delay is increased to a certain extent.
Therefore, we streamlined the design of the decoupling head, taking into account the balance between the representation capabilities of the relevant operators and the computational overhead of the hardware, and redesigned it using the Hybrid Channels strategy A more efficient decoupling head structure is developed, which reduces the delay while maintaining accuracy, and alleviates the additional delay overhead caused by the 3x3 convolution in the decoupling head. By conducting ablation experiments on a nano-size model and comparing the decoupling head structure with the same number of channels, the accuracy is increased by 0.2% AP and the speed is increased by 6.8%.
Figure 6 Efficient Decoupled Head structure diagram
2.3 More effective training strategy
In order to further improve detection accuracy, we draw on advanced research progress from other detection frameworks in academia and industry: Anchor-free anchor-free paradigm, SimOTA label allocation strategy and SIoU bounding box regression loss.
Anchor-free anchor-free paradigm
YOLOv6 adopts a more concise Anchor-free detection method. Since Anchor-based detectors need to perform cluster analysis before training to determine the optimal Anchor set, this will increase the complexity of the detector to a certain extent; at the same time, in some edge-end applications, a large number of detection results need to be transported between hardware steps will also bring additional delays. The Anchor-free anchor-free paradigm has been widely used in recent years due to its strong generalization ability and simpler decoding logic. After experimental research on Anchor-free, we found that compared to the additional delay caused by the complexity of the Anchor-based detector, the Anchor-free detector has a 51% improvement in speed.
SimOTA label allocation strategy
In order to obtain more high-quality positive samples, YOLOv6 introduced SimOTA [4]The algorithm dynamically allocates positive samples to further improve detection accuracy. The label allocation strategy of YOLOv5 is based on Shape matching, and increases the number of positive samples through the cross-grid matching strategy, thereby allowing the network to converge quickly. However, this method is a static allocation method and will not be adjusted along with the network training process.
In recent years, many methods based on dynamic label assignment have emerged. Such methods will allocate positive samples based on the network output during the training process, thereby producing more high-quality Positive samples, in turn, promote forward optimization of the network. For example, OTA[7] models sample matching as an optimal transmission problem and obtains the best sample matching strategy under global information to improve accuracy. However, OTA uses the Sinkhorn-Knopp algorithm, resulting in training The time is lengthened, and the SimOTA[4] algorithm uses the Top-K approximation strategy to obtain the best match of the sample, which greatly speeds up the training. Therefore, YOLOv6 adopts the SimOTA dynamic allocation strategy and combines it with the anchor-free paradigm to increase the average detection accuracy by 1.3% AP on the nano-size model.
SIoU bounding box regression loss
In order to further improve the regression accuracy, YOLOv6 adopts SIoU[9 ] Bounding box regression loss function to supervise the learning of the network. The training of target detection networks generally requires the definition of at least two loss functions: classification loss and bounding box regression loss, and the definition of the loss function often has a greater impact on detection accuracy and training speed.
In recent years, commonly used bounding box regression losses include IoU, GIoU, CIoU, DIoU loss, etc. These loss functions consider factors such as the degree of overlap between the prediction frame and the target frame, center point distance, aspect ratio, etc. To measure the gap between the two, thereby guiding the network to minimize the loss to improve regression accuracy, but these methods do not take into account the matching of the direction between the prediction box and the target box. The SIoU loss function redefines distance loss by introducing the vector angle between required regressions, effectively reducing the degree of freedom of regression, accelerating network convergence, and further improving regression accuracy. By using SIoU loss for experiments on YOLOv6s, compared with CIoU loss, the average detection accuracy is increased by 0.3% AP.
3. Experimental results
After the above optimization strategies and improvements, YOLOv6 has achieved excellent performance in multiple models of different sizes. Table 1 below shows the ablation experimental results of YOLOv6-nano. From the experimental results, we can see that our self-designed detection network has brought great gains in both accuracy and speed.
Table 1 YOLOv6-nano ablation experimental resultsTable 2 below shows the experimental results of YOLOv6 compared with other currently mainstream YOLO series algorithms. You can see from the table:
Table 2 Comparison of the performance of YOLOv6 models of various sizes with other models
- YOLOv6-nano achieved an accuracy of 35.0% AP on COCO val. At the same time, using TRT FP16 batchsize=32 for inference on T4, it can reach a performance of 1242FPS. Compared with YOLOv5-nano, the accuracy is increased by 7% AP. , the speed is increased by 85%.
- YOLOv6-tiny achieved an accuracy of 41.3% AP on COCO val. At the same time, using TRT FP16 batchsize=32 for inference on T4, it can achieve a performance of 602FPS, compared to YOLOv5 -s increases accuracy by 3.9% AP and speed by 29.4%.
- YOLOv6-s achieved an accuracy of 43.1% AP on COCO val. At the same time, using TRT FP16 batchsize=32 for inference on T4, it can achieve a performance of 520FPS, compared to YOLOX -s accuracy increases by 2.6% AP, and speed increases by 38.6%; compared to PP-YOLOE-s, which increases accuracy by 0.4% AP, using TRT FP16 on T4 for single-batch inference, the speed increases by 71.3%.
4. Summary and Outlook
This article introduces the optimization and practical experience of Meituan Visual Intelligence Department in the target detection framework , we have thought and optimized the training strategy, backbone network, multi-scale feature fusion, detection head, etc. for the YOLO series framework, and designed a new detection framework-YOLOv6. The original intention came from solving the actual problems encountered when implementing industrial applications. question.
While building the YOLOv6 framework, we explored and optimized some new methods, such as self-developed EfficientRep Backbone, Rep-Neck and Efficient Decoupled Head based on hardware-aware neural network design ideas. , and also draws on some cutting-edge developments and results in academia and industry, such as Anchor-free, SimOTA and SIoU regression loss. Experimental results on the COCO data set show that YOLOv6 is among the best in terms of detection accuracy and speed.
In the future, we will continue to build and improve the YOLOv6 ecosystem. The main work includes the following aspects:
- ##Improving the full range of YOLOv6 models , and continue to improve detection performance.
- Design hardware-friendly models on a variety of hardware platforms.
- Supports full-chain adaptation such as ARM platform deployment and quantitative distillation.
- Laterally expand and introduce related technologies, such as semi-supervised, self-supervised learning, etc.
- Explore the generalization performance of YOLOv6 in more unknown business scenarios.
The above is the detailed content of YOLOv6's fast and accurate target detection framework has been open sourced. For more information, please follow other related articles on the PHP Chinese website!

Hot AI Tools

Undresser.AI Undress
AI-powered app for creating realistic nude photos

AI Clothes Remover
Online AI tool for removing clothes from photos.

Undress AI Tool
Undress images for free

Clothoff.io
AI clothes remover

Video Face Swap
Swap faces in any video effortlessly with our completely free AI face swap tool!

Hot Article

Hot Tools

Notepad++7.3.1
Easy-to-use and free code editor

SublimeText3 Chinese version
Chinese version, very easy to use

Zend Studio 13.0.1
Powerful PHP integrated development environment

Dreamweaver CS6
Visual web development tools

SublimeText3 Mac version
God-level code editing software (SublimeText3)

Hot Topics




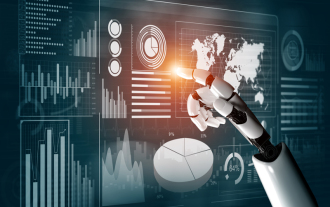
Text annotation is the work of corresponding labels or tags to specific content in text. Its main purpose is to provide additional information to the text for deeper analysis and processing, especially in the field of artificial intelligence. Text annotation is crucial for supervised machine learning tasks in artificial intelligence applications. It is used to train AI models to help more accurately understand natural language text information and improve the performance of tasks such as text classification, sentiment analysis, and language translation. Through text annotation, we can teach AI models to recognize entities in text, understand context, and make accurate predictions when new similar data appears. This article mainly recommends some better open source text annotation tools. 1.LabelStudiohttps://github.com/Hu
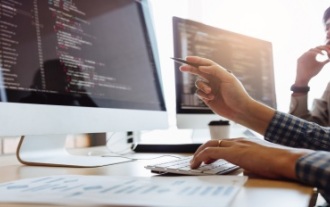
Image annotation is the process of associating labels or descriptive information with images to give deeper meaning and explanation to the image content. This process is critical to machine learning, which helps train vision models to more accurately identify individual elements in images. By adding annotations to images, the computer can understand the semantics and context behind the images, thereby improving the ability to understand and analyze the image content. Image annotation has a wide range of applications, covering many fields, such as computer vision, natural language processing, and graph vision models. It has a wide range of applications, such as assisting vehicles in identifying obstacles on the road, and helping in the detection and diagnosis of diseases through medical image recognition. . This article mainly recommends some better open source and free image annotation tools. 1.Makesens
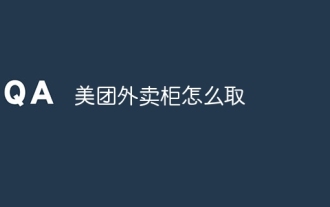
1. When the delivery clerk puts the meal into the cabinet, he will notify the customer to pick up the meal through text message, phone call or Meituan message. 2. Customers can scan the QR code on the food cabinet through WeChat or Meituan APP to enter the smart food cabinet applet. 3. Enter the pickup code or use the "one-click cabinet opening" function to easily open the cabinet door and take out the takeaway.
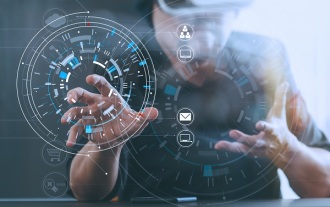
Face detection and recognition technology is already a relatively mature and widely used technology. Currently, the most widely used Internet application language is JS. Implementing face detection and recognition on the Web front-end has advantages and disadvantages compared to back-end face recognition. Advantages include reducing network interaction and real-time recognition, which greatly shortens user waiting time and improves user experience; disadvantages include: being limited by model size, the accuracy is also limited. How to use js to implement face detection on the web? In order to implement face recognition on the Web, you need to be familiar with related programming languages and technologies, such as JavaScript, HTML, CSS, WebRTC, etc. At the same time, you also need to master relevant computer vision and artificial intelligence technologies. It is worth noting that due to the design of the Web side
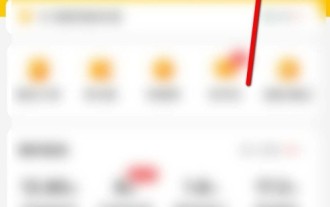
1. First, we enter the Meituan software, find Settings on the My Menu page, and click to enter Settings. 2. Then we find the payment settings on the settings page and click to enter the payment settings. 3. Enter the payment center, find the payment password settings, and click to enter the payment password settings. 4. In the payment password setting page, find the payment password retrieval and click to enter the page option. 5. Enter the payment password information you want to retrieve, click Verify, and you can retrieve the payment password after passing it.
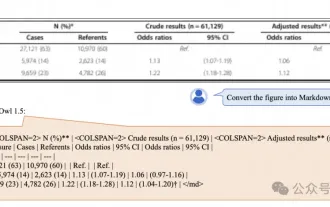
New SOTA for multimodal document understanding capabilities! Alibaba's mPLUG team released the latest open source work mPLUG-DocOwl1.5, which proposed a series of solutions to address the four major challenges of high-resolution image text recognition, general document structure understanding, instruction following, and introduction of external knowledge. Without further ado, let’s look at the effects first. One-click recognition and conversion of charts with complex structures into Markdown format: Charts of different styles are available: More detailed text recognition and positioning can also be easily handled: Detailed explanations of document understanding can also be given: You know, "Document Understanding" is currently An important scenario for the implementation of large language models. There are many products on the market to assist document reading. Some of them mainly use OCR systems for text recognition and cooperate with LLM for text processing.
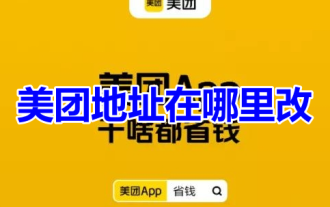
1. Where can I change my Meituan address? Meituan address modification tutorial! Method (1) 1. Enter Meituan My Page and click Settings. 2. Select personal information. 3. Click the shipping address again. 4. Finally, select the address you want to modify, click the pen icon on the right side of the address, and modify it. Method (2) 1. On the homepage of the Meituan app, click Takeout, then click More Functions after entering. 2. In the More interface, click Manage Address. 3. In the My Shipping Address interface, select Edit. 4. Modify them one by one according to your needs, and finally click to save the address.
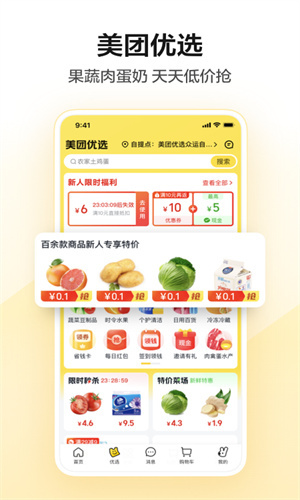
I believe that many players and users should be very familiar with the above functions, so when we use the kind of orders, we can give you a better understanding of some orders, so we When you choose to buy, you can directly generate some orders. However, when you want to cancel some orders, you can directly come over to learn about the methods in many aspects, so that everyone can better understand them. Understand it, it will be convenient for you to carry out various operations in the future. Today, the editor will give you a good explanation of the content and methods. Friends who have any ideas, you must not miss it. Come and try it with the editor now. Try it, I believe you will be very interested, don’t miss it.
