


GPT-4's 32k input box is still not enough? Unlimiformer stretches the context length to infinite length
Transformer is the most powerful seq2seq architecture today. Pretrained transformers typically have context windows of 512 (e.g. BERT) or 1024 (e.g. BART) tokens, which is long enough for many current text summarization datasets (XSum, CNN/DM).
But 16384 is not an upper limit on the length of context required to generate: tasks involving long narratives, such as book summaries (Krys-´cinski et al., 2021) or narrative question and answer (Kociskýet al. ., 2018), typically inputting more than 100,000 tokens. The challenge set generated from Wikipedia articles (Liu* et al., 2018) contains inputs of more than 500,000 tokens. Open-domain tasks in generative question answering can synthesize information from larger inputs, such as answering questions about the aggregated properties of articles by all living authors on Wikipedia. Figure 1 plots the sizes of several popular summarization and Q&A datasets against common context window lengths; the longest input is more than 34 times longer than Longformer’s context window.
In the case of these very long inputs, the vanilla transformer cannot scale because the native attention mechanism has quadratic complexity. Long input transformers, while more efficient than standard transformers, still require significant computational resources that increase as the context window size increases. Furthermore, increasing the context window requires retraining the model from scratch with the new context window size, which is computationally and environmentally expensive.
In the article "Unlimiformer: Long-Range Transformers with Unlimited Length Input", researchers from Carnegie Mellon University introduced Unlimiformer. This is a retrieval-based approach that augments a pre-trained language model to accept infinite-length input at test time.
Paper link: https://arxiv.org/pdf/2305.01625v1.pdf
Unlimiformer can be injected into any existing encoder-decoder transformer, capable of handling input of unlimited length. Given a long input sequence, Unlimiformer can build a data store on the hidden states of all input tokens. The decoder's standard cross-attention mechanism is then able to query the data store and focus on the top k input tokens. The data store can be stored in GPU or CPU memory and can be queried sub-linearly.
Unlimiformer can be applied directly to a trained model and can improve existing checkpoints without any further training. The performance of Unlimiformer will be further improved after fine-tuning. This paper demonstrates that Unlimiformer can be applied to multiple base models, such as BART (Lewis et al., 2020a) or PRIMERA (Xiao et al., 2022), without adding weights and retraining. In various long-range seq2seq data sets, Unlimiformer is not only stronger than long-range Transformers such as Longformer (Beltagy et al., 2020b), SLED (Ivgi et al., 2022) and Memorizing transformers (Wu et al., 2021) on these data sets The performance is better, and this article also found that Unlimiform can be applied on top of the Longformer encoder model to make further improvements.
Unlimiformer technical principle
Since the size of the encoder context window is fixed, the maximum input length of the Transformer is limited. However, during decoding, different information may be relevant; furthermore, different attention heads may focus on different types of information (Clark et al., 2019). Therefore, a fixed context window may waste effort on tokens to which attention is less focused.
At each decoding step, each attention head in Unlimiformer selects a separate context window from all inputs. This is achieved by injecting the Unlimiformer lookup into the decoder: before entering the cross-attention module, the model performs a k-nearest neighbor (kNN) search in the external data store, selecting a set of each attention head in each decoder layer. token to participate.
coding
In order to encode input sequences longer than the context window length of the model, this article encodes the input overlapping blocks according to the method of Ivgi et al. (2022) (Ivgi et al., 2022), only The middle half of the output for each chunk is retained to ensure sufficient context before and after the encoding process. Finally, this article uses libraries such as Faiss (Johnson et al., 2019) to index encoded inputs in data stores (Johnson et al., 2019).
Retrieve enhanced cross-attention mechanism
In the standard cross-attention mechanism, the decoder of the transformer Focusing on the final hidden state of the encoder, the encoder usually truncates the input and encodes only the first k tokens in the input sequence.
This article does not only focus on the first k tokens of the input. For each cross attention head, it retrieves the first k hidden states of the longer input series and only focuses on the first k tokens. k. This allows the keyword to be retrieved from the entire input sequence rather than truncating the keyword. Our approach is also cheaper in terms of computation and GPU memory than processing all input tokens, while typically retaining over 99% of attention performance.
Figure 2 shows this article’s changes to the seq2seq transformer architecture. The complete input is block-encoded using the encoder and stored in a data store; the encoded latent state data store is then queried when decoding. The kNN search is non-parametric and can be injected into any pretrained seq2seq transformer, as detailed below.
Long document summary
Table 3 shows the results in the long text (4k and 16k token input) summary dataset.
Among the training methods in Table 4, Unlimiformer can achieve the best in various indicators.
Book Summary
Table 5 Display Results on book abstracts. It can be seen that based on BARTbase and PRIMERA, applying Unlimiformer can achieve certain improvement results.
The above is the detailed content of GPT-4's 32k input box is still not enough? Unlimiformer stretches the context length to infinite length. For more information, please follow other related articles on the PHP Chinese website!

Hot AI Tools

Undresser.AI Undress
AI-powered app for creating realistic nude photos

AI Clothes Remover
Online AI tool for removing clothes from photos.

Undress AI Tool
Undress images for free

Clothoff.io
AI clothes remover

AI Hentai Generator
Generate AI Hentai for free.

Hot Article

Hot Tools

Notepad++7.3.1
Easy-to-use and free code editor

SublimeText3 Chinese version
Chinese version, very easy to use

Zend Studio 13.0.1
Powerful PHP integrated development environment

Dreamweaver CS6
Visual web development tools

SublimeText3 Mac version
God-level code editing software (SublimeText3)

Hot Topics


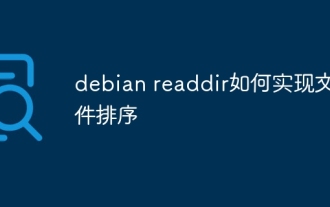
In Debian systems, the readdir function is used to read directory contents, but the order in which it returns is not predefined. To sort files in a directory, you need to read all files first, and then sort them using the qsort function. The following code demonstrates how to sort directory files using readdir and qsort in Debian system: #include#include#include#include#include//Custom comparison function, used for qsortintcompare(constvoid*a,constvoid*b){returnstrcmp(*(
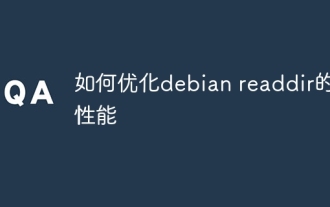
In Debian systems, readdir system calls are used to read directory contents. If its performance is not good, try the following optimization strategy: Simplify the number of directory files: Split large directories into multiple small directories as much as possible, reducing the number of items processed per readdir call. Enable directory content caching: build a cache mechanism, update the cache regularly or when directory content changes, and reduce frequent calls to readdir. Memory caches (such as Memcached or Redis) or local caches (such as files or databases) can be considered. Adopt efficient data structure: If you implement directory traversal by yourself, select more efficient data structures (such as hash tables instead of linear search) to store and access directory information
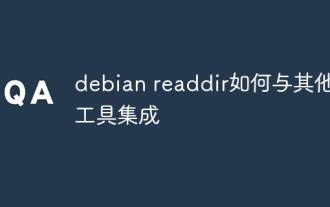
The readdir function in the Debian system is a system call used to read directory contents and is often used in C programming. This article will explain how to integrate readdir with other tools to enhance its functionality. Method 1: Combining C language program and pipeline First, write a C program to call the readdir function and output the result: #include#include#include#includeintmain(intargc,char*argv[]){DIR*dir;structdirent*entry;if(argc!=2){
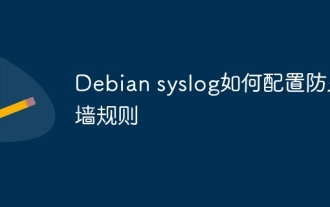
This article describes how to configure firewall rules using iptables or ufw in Debian systems and use Syslog to record firewall activities. Method 1: Use iptablesiptables is a powerful command line firewall tool in Debian system. View existing rules: Use the following command to view the current iptables rules: sudoiptables-L-n-v allows specific IP access: For example, allow IP address 192.168.1.100 to access port 80: sudoiptables-AINPUT-ptcp--dport80-s192.16
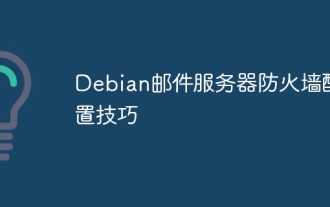
Configuring a Debian mail server's firewall is an important step in ensuring server security. The following are several commonly used firewall configuration methods, including the use of iptables and firewalld. Use iptables to configure firewall to install iptables (if not already installed): sudoapt-getupdatesudoapt-getinstalliptablesView current iptables rules: sudoiptables-L configuration
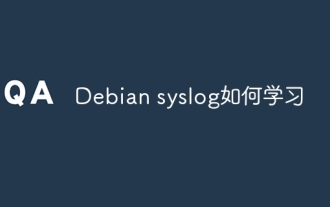
This guide will guide you to learn how to use Syslog in Debian systems. Syslog is a key service in Linux systems for logging system and application log messages. It helps administrators monitor and analyze system activity to quickly identify and resolve problems. 1. Basic knowledge of Syslog The core functions of Syslog include: centrally collecting and managing log messages; supporting multiple log output formats and target locations (such as files or networks); providing real-time log viewing and filtering functions. 2. Install and configure Syslog (using Rsyslog) The Debian system uses Rsyslog by default. You can install it with the following command: sudoaptupdatesud
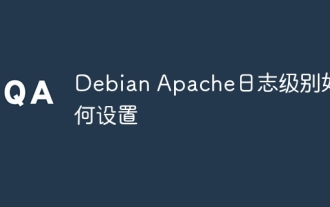
This article describes how to adjust the logging level of the ApacheWeb server in the Debian system. By modifying the configuration file, you can control the verbose level of log information recorded by Apache. Method 1: Modify the main configuration file to locate the configuration file: The configuration file of Apache2.x is usually located in the /etc/apache2/ directory. The file name may be apache2.conf or httpd.conf, depending on your installation method. Edit configuration file: Open configuration file with root permissions using a text editor (such as nano): sudonano/etc/apache2/apache2.conf
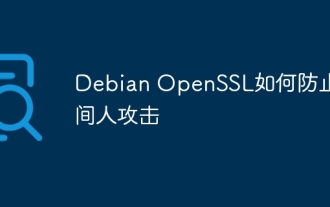
In Debian systems, OpenSSL is an important library for encryption, decryption and certificate management. To prevent a man-in-the-middle attack (MITM), the following measures can be taken: Use HTTPS: Ensure that all network requests use the HTTPS protocol instead of HTTP. HTTPS uses TLS (Transport Layer Security Protocol) to encrypt communication data to ensure that the data is not stolen or tampered during transmission. Verify server certificate: Manually verify the server certificate on the client to ensure it is trustworthy. The server can be manually verified through the delegate method of URLSession
