


Self-regulation is now the control standard for artificial intelligence
Are you worried that artificial intelligence is developing too fast and may have negative consequences? Do you wish there was a national law regulating it? Today, there are no new laws to restrict the use of AI, and often self-regulation becomes the best option for companies adopting AI – at least for now.
Although "artificial intelligence" has replaced "big data" as the hottest buzzword in the technology world for many years, in late November 2022 ChatGPT The launch of AI started an AI gold rush that surprised many AI observers, including us. In just a few months, a slew of powerful generative AI models have captured the world’s attention, thanks to their remarkable ability to mimic human language and understanding.
The extraordinary rise of generative models in mainstream culture, fueled by the emergence of ChatGPT, raises many questions about where this is all headed. The astonishing power of AI to produce compelling poetry and whimsical art is giving way to concerns about its negative consequences, ranging from consumer harm and job losses, all the way to false imprisonment and even the destruction of humanity.
This has some people very worried. Last month, a coalition of AI researchers sought a six-month moratorium on the development of new generative models larger than GPT-4 (Further reading: Open letter urges moratorium on AI research), GPT-4, the massive model OpenAI launched last month Language Model (LLM).
An open letter signed by Turing Award winner Yoshua Bengio and OpenAI co-founder Elon Musk and others stated: "Advanced artificial intelligence may represent profound changes in the history of life on Earth and should Plan and manage with appropriate care and resources.” “Unfortunately, this level of planning and management has not been achieved.” The call is rising. Polls show Americans don’t think artificial intelligence can be trusted and want it regulated, especially on impactful things like self-driving cars and access to government benefits.
Yet despite several new local laws targeting AI — such as one in New York City that focuses on the use of AI in hiring — enforcement efforts have been delayed As of this month—Congress has no new federal regulations specifically targeting AI that’s approaching the finish line (although AI has made its way into the legal realm of highly regulated industries like financial services and health care).
Spurred by artificial intelligence, what should a company do? It’s no surprise that companies want to share the benefits of artificial intelligence. After all, the urge to become “data-driven” is seen as a necessity for survival in the digital age. However, companies also want to avoid the negative consequences, real or perceived, that can result from inappropriate use of AI.
Artificial intelligence is wild in "
Westworld". Andrew Burt, founder of artificial intelligence law firm BNH.AI, once said, "No one knows how to manage risk. Everyone does it differently." That being said, companies can use Several frameworks to help manage AI risks. Burt recommends using the Artificial Intelligence Risk Management Framework (
#RMF: Risk Management Framework), which comes from the National Institute of Standards and Technology (NIST) and was finalized earlier this year. RMF helps companies think about how their artificial intelligence works and the potential negative consequences it may have. It uses a “map, measure, manage and govern” approach to understand and ultimately mitigate the risks of using artificial intelligence across a variety of service offerings.
Another AI risk management framework comes from Cathy O'Neil, CEO of O'Neil Risk Advisory & Algorithmic Auditing (ORCAA). ORCAA proposed a framework called "
Explainable Fairness". Explainable fairness gives organizations a way to not only test their algorithms for bias, but also study what happens when differences in outcomes are detected. For example, if a bank is determining eligibility for a student loan, what factors can legally be used to approve or deny the loan or charge higher or lower interest?
Clearly, banks must use data to answer these questions. But what data can they use—that is, what factors reflect a loan applicant? Which factors should be legally allowed to be used and which factors should not be used? Answering these questions is neither easy nor simple, O'Neil said.
#"That's what this framework is all about, is that these legal factors have to be legal," O'Neil said during a discussion at the Nvidia GPU Technology Conference (GTC) last month. ization."
Even without new AI laws, companies should start asking themselves how to be fair and compliant, said Triveni Gandhi, head of Dataiku AI, a provider of data analytics and AI software. Implement AI ethically to comply with existing laws.
“People have to start thinking, okay, how do we take existing laws and apply them to the AI use cases that exist today?” “There are some regulations, but there are also a lot People are thinking about the ethical and value-oriented ways in which we want to build artificial intelligence. These are actually the questions companies are starting to ask themselves, even if there are no overarching prescriptions."
EU classifies potential harms of artificial intelligence into 'criticality pyramid'
The EU is already moving forward with its own regulations, the Artificial Intelligence Act , the bill could take effect later this year.
The Artificial Intelligence Bill will create a common regulatory and legal framework for the use of artificial intelligence that affects EU residents, including how it is developed and what companies can use it for purpose, and the legal consequences of failure to comply with the requirements. The law could require companies to get approval before adopting AI in certain use cases and ban certain other uses of AI deemed too risky.
The above is the detailed content of Self-regulation is now the control standard for artificial intelligence. For more information, please follow other related articles on the PHP Chinese website!

Hot AI Tools

Undresser.AI Undress
AI-powered app for creating realistic nude photos

AI Clothes Remover
Online AI tool for removing clothes from photos.

Undress AI Tool
Undress images for free

Clothoff.io
AI clothes remover

AI Hentai Generator
Generate AI Hentai for free.

Hot Article

Hot Tools

Notepad++7.3.1
Easy-to-use and free code editor

SublimeText3 Chinese version
Chinese version, very easy to use

Zend Studio 13.0.1
Powerful PHP integrated development environment

Dreamweaver CS6
Visual web development tools

SublimeText3 Mac version
God-level code editing software (SublimeText3)

Hot Topics


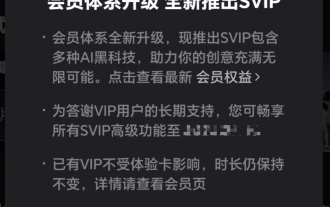
This site reported on June 27 that Jianying is a video editing software developed by FaceMeng Technology, a subsidiary of ByteDance. It relies on the Douyin platform and basically produces short video content for users of the platform. It is compatible with iOS, Android, and Windows. , MacOS and other operating systems. Jianying officially announced the upgrade of its membership system and launched a new SVIP, which includes a variety of AI black technologies, such as intelligent translation, intelligent highlighting, intelligent packaging, digital human synthesis, etc. In terms of price, the monthly fee for clipping SVIP is 79 yuan, the annual fee is 599 yuan (note on this site: equivalent to 49.9 yuan per month), the continuous monthly subscription is 59 yuan per month, and the continuous annual subscription is 499 yuan per year (equivalent to 41.6 yuan per month) . In addition, the cut official also stated that in order to improve the user experience, those who have subscribed to the original VIP
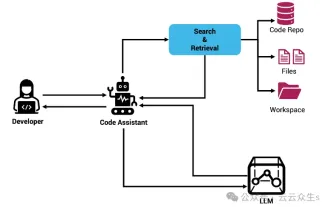
Improve developer productivity, efficiency, and accuracy by incorporating retrieval-enhanced generation and semantic memory into AI coding assistants. Translated from EnhancingAICodingAssistantswithContextUsingRAGandSEM-RAG, author JanakiramMSV. While basic AI programming assistants are naturally helpful, they often fail to provide the most relevant and correct code suggestions because they rely on a general understanding of the software language and the most common patterns of writing software. The code generated by these coding assistants is suitable for solving the problems they are responsible for solving, but often does not conform to the coding standards, conventions and styles of the individual teams. This often results in suggestions that need to be modified or refined in order for the code to be accepted into the application
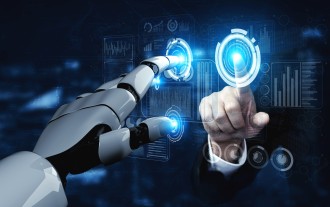
Large Language Models (LLMs) are trained on huge text databases, where they acquire large amounts of real-world knowledge. This knowledge is embedded into their parameters and can then be used when needed. The knowledge of these models is "reified" at the end of training. At the end of pre-training, the model actually stops learning. Align or fine-tune the model to learn how to leverage this knowledge and respond more naturally to user questions. But sometimes model knowledge is not enough, and although the model can access external content through RAG, it is considered beneficial to adapt the model to new domains through fine-tuning. This fine-tuning is performed using input from human annotators or other LLM creations, where the model encounters additional real-world knowledge and integrates it

To learn more about AIGC, please visit: 51CTOAI.x Community https://www.51cto.com/aigc/Translator|Jingyan Reviewer|Chonglou is different from the traditional question bank that can be seen everywhere on the Internet. These questions It requires thinking outside the box. Large Language Models (LLMs) are increasingly important in the fields of data science, generative artificial intelligence (GenAI), and artificial intelligence. These complex algorithms enhance human skills and drive efficiency and innovation in many industries, becoming the key for companies to remain competitive. LLM has a wide range of applications. It can be used in fields such as natural language processing, text generation, speech recognition and recommendation systems. By learning from large amounts of data, LLM is able to generate text
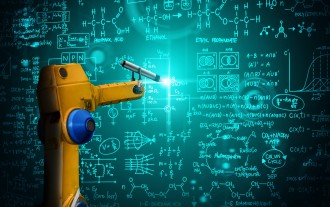
Machine learning is an important branch of artificial intelligence that gives computers the ability to learn from data and improve their capabilities without being explicitly programmed. Machine learning has a wide range of applications in various fields, from image recognition and natural language processing to recommendation systems and fraud detection, and it is changing the way we live. There are many different methods and theories in the field of machine learning, among which the five most influential methods are called the "Five Schools of Machine Learning". The five major schools are the symbolic school, the connectionist school, the evolutionary school, the Bayesian school and the analogy school. 1. Symbolism, also known as symbolism, emphasizes the use of symbols for logical reasoning and expression of knowledge. This school of thought believes that learning is a process of reverse deduction, through existing
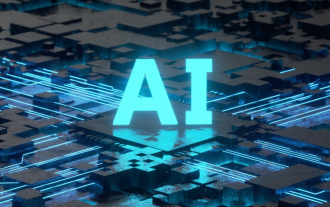
Editor |ScienceAI Question Answering (QA) data set plays a vital role in promoting natural language processing (NLP) research. High-quality QA data sets can not only be used to fine-tune models, but also effectively evaluate the capabilities of large language models (LLM), especially the ability to understand and reason about scientific knowledge. Although there are currently many scientific QA data sets covering medicine, chemistry, biology and other fields, these data sets still have some shortcomings. First, the data form is relatively simple, most of which are multiple-choice questions. They are easy to evaluate, but limit the model's answer selection range and cannot fully test the model's ability to answer scientific questions. In contrast, open-ended Q&A
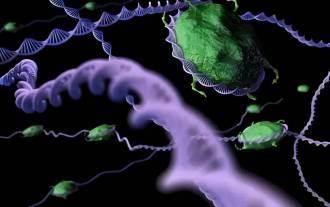
Editor | KX In the field of drug research and development, accurately and effectively predicting the binding affinity of proteins and ligands is crucial for drug screening and optimization. However, current studies do not take into account the important role of molecular surface information in protein-ligand interactions. Based on this, researchers from Xiamen University proposed a novel multi-modal feature extraction (MFE) framework, which for the first time combines information on protein surface, 3D structure and sequence, and uses a cross-attention mechanism to compare different modalities. feature alignment. Experimental results demonstrate that this method achieves state-of-the-art performance in predicting protein-ligand binding affinities. Furthermore, ablation studies demonstrate the effectiveness and necessity of protein surface information and multimodal feature alignment within this framework. Related research begins with "S
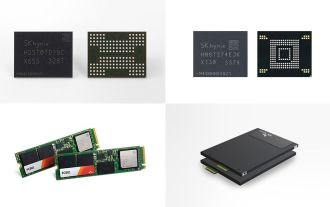
According to news from this site on August 1, SK Hynix released a blog post today (August 1), announcing that it will attend the Global Semiconductor Memory Summit FMS2024 to be held in Santa Clara, California, USA from August 6 to 8, showcasing many new technologies. generation product. Introduction to the Future Memory and Storage Summit (FutureMemoryandStorage), formerly the Flash Memory Summit (FlashMemorySummit) mainly for NAND suppliers, in the context of increasing attention to artificial intelligence technology, this year was renamed the Future Memory and Storage Summit (FutureMemoryandStorage) to invite DRAM and storage vendors and many more players. New product SK hynix launched last year
