Baidu Wenxinyiyan ranks last among domestic models? I was confused
Xi Xiaoyao Technology Talk Original
Author | Selling Mengjiang In recent days, our public account community has been forwarding a screenshot called SuperClue review. iFlytek even promoted it on its official account:
# Since the iFlytek Spark model has just been released, I haven’t played it very much. Is it really the most powerful one made in China? The author dares not draw any conclusions.
But in the screenshot of this evaluation, Baidu Wenxinyiyan, the most popular domestic model at the moment, can't even beat a small academic open source model ChatGLM-6B. Not only is this seriously inconsistent with the author’s own experience, but in our professional NLP technical community, everyone also expressed confusion:
Out of curiosity, the author went to the github of this superclue list to see how this evaluation conclusion was reached: https://www.php.cn/link/97c8dd44858d3568fdf9537c4b8743b2
First of all, the author noticed that there are already some issues under this repo:
It seems that this outrageous feeling is not only The author has it, and sure enough, the masses’ eyes are still sharp. . .
The author further took a look at the evaluation method of this list:
Good guy, it turns out that the so-called generative large model tests are all about letting The model does multiple choice questions. . .
Obviously, this multiple-choice evaluation method is aimed at the discriminative AI model in the BERT era. At that time, the AI model generally did not have the ability to generate, but only had the ability to discriminate (such as being able to determine what a piece of text belongs to) Category, which of the options is the correct answer to the question, judging whether the semantics of two pieces of text are consistent, etc.).
The evaluation of generative models is quite different from the evaluation of discriminative models.
For example, for special generation tasks such as machine translation, evaluation indicators such as BLEU are generally used to detect the "vocabulary and phrase coverage" between the responses generated by the model and the reference responses. However, there are very few generative tasks with reference responses such as machine translation, and the vast majority of generative evaluations require manual evaluation.
For example, generation tasks such as chat-style dialogue generation, text style transfer, chapter generation, title generation, text summary, etc. require each model to be evaluated to freely generate responses, and then manually compare the responses generated by these different models. Quality, or human judgment as to whether task requirements are met.
The current round of AI competition is a competition for model generation capabilities, not a competition for model discrimination capabilities. The most powerful thing to evaluate is real user reputation, not cold academic lists anymore. What's more, it's a list that doesn't test model generation capabilities at all.
Looking back on the past few years-
In 2019, when OpenAI released GPT-2, we were piling up tricks to brush up the rankings;
In 2020, OpenAI released During GPT-3, we were piling up tricks to refresh the list;
2021-2022, when instruction tuning and RLHF work such as FLAN, T0, InstructGPT and so on broke out, we still had many teams insisting on piling up tricks to refresh the list. List...
I hope we will not repeat the same mistakes in this wave of generative model arms race.
So how should the generative AI model be tested?
I'm sorry, as I said before, it is very, very difficult to achieve unbiased testing, even more difficult than developing a generative model yourself. What are the difficulties? A few specific questions:
- How to divide the evaluation dimensions? By understanding, memory, reasoning, expression? By area of expertise? Or combine traditional NLP generative evaluation tasks?
- How to train evaluators? For test questions with extremely high professional thresholds such as coding, debugging, mathematical derivation, and financial, legal, and medical Q&A, how do you recruit people to test?
- How to define the evaluation criteria for highly subjective test questions (such as generating Xiaohongshu-style copywriting)?
- Can asking a few general writing questions represent a model’s text generation/writing ability?
- Examine the text generation sub-capabilities of the model. Are chapter generation, question and answer generation, translation, summary, and style transfer covered? Are the proportions of each task even? Are the judging criteria clear? Statistically significant?
- In the above question and answer generation sub-task, are all vertical categories such as science, medical care, automobiles, mother and baby, finance, engineering, politics, military, entertainment, etc. covered? Is the proportion even?
- How to evaluate conversational ability? How to design the inspection tasks for the consistency, diversity, topic depth, and personification of dialogue?
- For the same ability test, are simple questions, medium difficulty questions and complex long-term questions covered? How to define? What proportions do they account for?
These are just a few basic problems to be solved. In the process of actual benchmark design, we have to face a large number of problems that are much more difficult than the above problems.
Therefore, as an AI practitioner, the author calls on everyone to view the rankings of various AI models rationally. There isn't even an unbiased test benchmark, so what's the use of this ranking?
Again, whether a generative model is good or not depends on real users.
No matter how high a model ranks on a list, if it cannot solve the problem you care about, it will be just an average model to you. In other words, if a model ranked at the bottom is very strong in the scenario you are concerned about, then it is a treasure model for you.
Here, the author discloses a hard case (difficult example) test set enriched and written by our team. This test set focuses on the model's ability to solve difficult problems/instructions.
This difficult test set focuses on the model's language understanding, understanding and following complex instructions, text generation, complex content generation, multiple rounds of dialogue, contradiction detection, common sense reasoning, mathematical reasoning, counterfactual reasoning, and hazard information Identification, legal and ethical awareness, Chinese literature knowledge, cross-language ability and coding ability, etc.
Again, this is a case set made by the author’s team to test the generative model’s ability to solve difficult examples. The evaluation results can only represent “which model feels better to the author’s team?” , is far from representing an unbiased test conclusion. If you want an unbiased test conclusion, please answer the evaluation questions mentioned above first, and then define an authoritative test benchmark.
Friends who want to evaluate and verify by themselves can reply to the [AI Evaluation] password in the background of this public account "Xi Xiaoyao Technology" to download the test file
The following are the evaluation results of the three most controversial models on the superclue list: iFlytek Spark, Wenxin Yiyan and ChatGPT:
- ChatGPT (GPT-3.5-turbo): 11/24=45.83%
- Wen Xinyi Words (2023.5.10 version): 13/24=54.16%
- iFlytek Spark (2023.5.10 version): 7/24=29.16%
For simple questions, there is actually not much difference between the domestic model and ChatGPT. For difficult problems, each model has its own strengths. Judging from the author's team's comprehensive experience, Wen Xinyiyan is enough to beat open source models such as ChatGLM-6B for academic testing. Some capabilities are inferior to ChatGPT, and some capabilities surpass ChatGPT.
The same is true for domestic models produced by other major manufacturers such as Alibaba Tongyi Qianwen and iFlytek Spark.
Still saying that, now there is not even an unbiased test benchmark, so what’s the use of ranking the models?
Rather than arguing about various biased rankings, it is better to make a test set that you care about like the author's team did.
A model that can solve your problem is a good model.
The above is the detailed content of Baidu Wenxinyiyan ranks last among domestic models? I was confused. For more information, please follow other related articles on the PHP Chinese website!

Hot AI Tools

Undresser.AI Undress
AI-powered app for creating realistic nude photos

AI Clothes Remover
Online AI tool for removing clothes from photos.

Undress AI Tool
Undress images for free

Clothoff.io
AI clothes remover

AI Hentai Generator
Generate AI Hentai for free.

Hot Article

Hot Tools

Notepad++7.3.1
Easy-to-use and free code editor

SublimeText3 Chinese version
Chinese version, very easy to use

Zend Studio 13.0.1
Powerful PHP integrated development environment

Dreamweaver CS6
Visual web development tools

SublimeText3 Mac version
God-level code editing software (SublimeText3)

Hot Topics


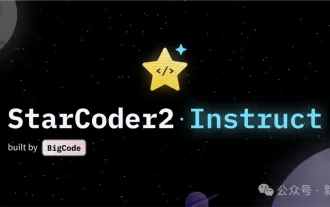
At the forefront of software technology, UIUC Zhang Lingming's group, together with researchers from the BigCode organization, recently announced the StarCoder2-15B-Instruct large code model. This innovative achievement achieved a significant breakthrough in code generation tasks, successfully surpassing CodeLlama-70B-Instruct and reaching the top of the code generation performance list. The unique feature of StarCoder2-15B-Instruct is its pure self-alignment strategy. The entire training process is open, transparent, and completely autonomous and controllable. The model generates thousands of instructions via StarCoder2-15B in response to fine-tuning the StarCoder-15B base model without relying on expensive manual annotation.
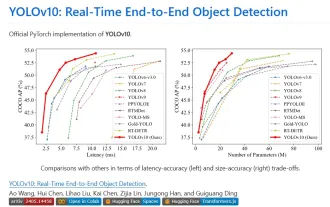
1. Introduction Over the past few years, YOLOs have become the dominant paradigm in the field of real-time object detection due to its effective balance between computational cost and detection performance. Researchers have explored YOLO's architectural design, optimization goals, data expansion strategies, etc., and have made significant progress. At the same time, relying on non-maximum suppression (NMS) for post-processing hinders end-to-end deployment of YOLO and adversely affects inference latency. In YOLOs, the design of various components lacks comprehensive and thorough inspection, resulting in significant computational redundancy and limiting the capabilities of the model. It offers suboptimal efficiency, and relatively large potential for performance improvement. In this work, the goal is to further improve the performance efficiency boundary of YOLO from both post-processing and model architecture. to this end
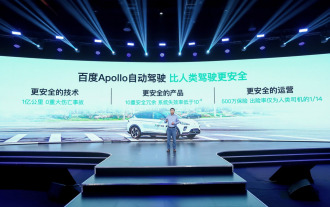
On May 15, Baidu Apollo held Apollo Day 2024 in Wuhan Baidu Luobo Automobile Robot Zhixing Valley, comprehensively demonstrating Baidu's major progress in autonomous driving over the past ten years, bringing technological leaps based on large models and a new definition of passenger safety. With the world's largest autonomous vehicle operation network, Baidu has made autonomous driving safer than human driving. Thanks to this, safer, more comfortable, green and low-carbon travel methods are turning from ideal to reality. Wang Yunpeng, vice president of Baidu Group and president of the Intelligent Driving Business Group, said on the spot: "Our original intention to build autonomous vehicles is to satisfy people's growing yearning for better travel. People's satisfaction is our driving force. Because safety, So beautiful, we are happy to see
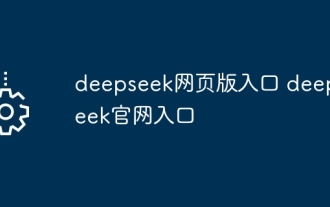
DeepSeek is a powerful intelligent search and analysis tool that provides two access methods: web version and official website. The web version is convenient and efficient, and can be used without installation; the official website provides comprehensive product information, download resources and support services. Whether individuals or corporate users, they can easily obtain and analyze massive data through DeepSeek to improve work efficiency, assist decision-making and promote innovation.
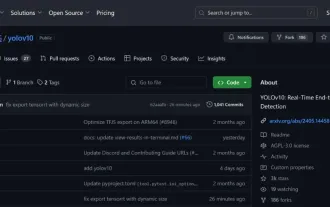
The benchmark YOLO series of target detection systems has once again received a major upgrade. Since the release of YOLOv9 in February this year, the baton of the YOLO (YouOnlyLookOnce) series has been passed to the hands of researchers at Tsinghua University. Last weekend, the news of the launch of YOLOv10 attracted the attention of the AI community. It is considered a breakthrough framework in the field of computer vision and is known for its real-time end-to-end object detection capabilities, continuing the legacy of the YOLO series by providing a powerful solution that combines efficiency and accuracy. Paper address: https://arxiv.org/pdf/2405.14458 Project address: https://github.com/THU-MIG/yo
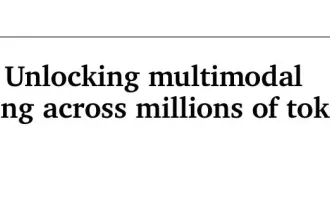
In February this year, Google launched the multi-modal large model Gemini 1.5, which greatly improved performance and speed through engineering and infrastructure optimization, MoE architecture and other strategies. With longer context, stronger reasoning capabilities, and better handling of cross-modal content. This Friday, Google DeepMind officially released the technical report of Gemini 1.5, which covers the Flash version and other recent upgrades. The document is 153 pages long. Technical report link: https://storage.googleapis.com/deepmind-media/gemini/gemini_v1_5_report.pdf In this report, Google introduces Gemini1
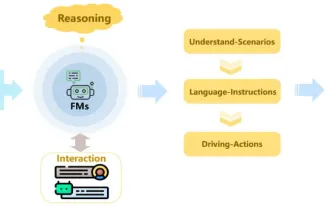
Written above & the author’s personal understanding: Recently, with the development and breakthroughs of deep learning technology, large-scale foundation models (Foundation Models) have achieved significant results in the fields of natural language processing and computer vision. The application of basic models in autonomous driving also has great development prospects, which can improve the understanding and reasoning of scenarios. Through pre-training on rich language and visual data, the basic model can understand and interpret various elements in autonomous driving scenarios and perform reasoning, providing language and action commands for driving decision-making and planning. The base model can be data augmented with an understanding of the driving scenario to provide those rare feasible features in long-tail distributions that are unlikely to be encountered during routine driving and data collection.
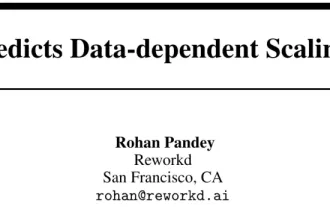
Generally speaking, the more calculations it takes to train a neural network, the better its performance. When scaling up a calculation, a decision must be made: increase the number of model parameters or increase the size of the data set—two factors that must be weighed within a fixed computational budget. The advantage of increasing the number of model parameters is that it can improve the complexity and expression ability of the model, thereby better fitting the training data. However, too many parameters can lead to overfitting, making the model perform poorly on unseen data. On the other hand, expanding the data set size can improve the generalization ability of the model and reduce overfitting problems. Let us tell you: As long as you allocate parameters and data appropriately, you can maximize performance within a fixed computing budget. Many previous studies have explored Scalingl of neural language models.
