


Exclusive interview with ByteDance Wang Mingxuan: Machine translation and manual translation are essentially two tracks | T Frontline
The advancement of technology often means that the evolution of the industry has found a new direction. The translation industry is no exception. As the process of globalization continues to accelerate, people cannot do without cross-language communication when conducting foreign-related activities. The emergence of machine translation has greatly expanded the application scenarios of translation. Although it is far from perfect, it has taken a solid step towards mankind's challenge to the Tower of Babel. 51CTO specially invited Wang Mingxuan, head of machine translation at ByteDance AI Lab, to talk about the development of machine translation over the years.
From rule-based, to statistical model-based, to neural network-based
The development of machine translation is closely related to the development of computer technology, information theory, linguistics and other disciplines. After entering the 21st century, with the improvement of hardware capabilities and optimization of algorithms, machine translation technology has ushered in an unprecedented leap forward, and has successfully stepped out of the ivory tower and embarked on the road to inclusiveness.
51CTO: Throughout history, what important development nodes has machine translation experienced?
Wang Mingxuan: Machine translation is essentially a very old problem. The history of machine translation can be traced back to the "universal" proposed by philosophers such as Descartes and Leibniz in the 17th century. language". After the official birth of computers in 1946, people expected computers to be able to translate one language into another. American scientist Warren Weaver formally defined the concept and ideas of machine translation in "Translation Memo". During this period, in the context of the Cold War, the United States and the Soviet Union also invested a lot of money in machine translation-related research based on the need to collect information.
At the beginning, everyone was relatively optimistic, thinking that this matter would be settled soon. The first version of the translation system was very simple, mainly based on dictionaries, such as translating "sun" into "sun". However, this word-to-word translation quickly encountered a bottleneck because there were many polysemy phenomena, such as "Bank" can be either "bank" or "river bank". In specific contexts, you will face many word selection dilemmas. Combining the semantic rules formulated by linguists can resolve some ambiguities, but in the later stages of development, the more rules there are, the more conflicts there will be, and the system will become more and more complex, but the problem still cannot be solved.
In 1966, the United States published the report "Language and Machine", which comprehensively denied the feasibility of machine translation and recommended that financial support for machine translation projects be stopped. Affected by this, machine translation has fallen into a low ebb.
Until the 1990s, IBM proposed a translation model based on word alignment, marking the birth of modern statistical machine translation methods. The principle of machine translation based on statistics is very simple. For example, if you want to determine whether bank should be translated into "bank" or "river bank" in the context, then conduct a large amount of relevant corpus statistics, and you will find that there is something related to "money" in the context, then It is more likely to be translated as "bank". If the context mentions "river", it is more likely to correspond to "river bank". In this way, does not use dictionaries and grammatical rules, but judges the semantics in specific scenarios based on probability. This is an epoch-making change, and the quality of machine translation has been greatly improved. Soon, machine translation began to be implemented in many practical scenarios.
The period from 1993 to 2014 basically belonged to the era of statistics. However, although it was based on statistics, it still required manual work to define many features and templates, and then further design details. Therefore, it was not very flexible, and the power of the model was not very powerful.
Then in the neural network era, neural network translation mainly includes encoders and decoders from a model perspective. The encoder represents the source language into a high-dimensional vector after a series of neural network transformations, and the decoder is responsible for re-decoding this high-dimensional vector into the target language. With the introduction of Seq2Seq in 2014, neural network translation gradually began to do better than statistical machine translation.
By 2017, Google proposed Transformer, which has a larger model, more flexible structure, and higher degree of parallelization, which further improved the quality of translation. In the same year, AlphaGo’s victory also made everyone more confident in artificial intelligence. It was also after 2017 that the industrialization of machine translation ushered in an explosive period. Until now, the overall framework has not changed much, but there have been many innovations in small details.
Challenging the "Tower of Babel"
From dictionary matching, to rule translation combined with the knowledge of linguistic experts, to corpus-based statistical machine translation, and the current mainstream neural network machine translation, Compared with before, the quality of machine translation has improved dramatically, but it still faces many challenges.
51CTO: What are the main challenges currently facing machine translation?
Wang Mingxuan: There are actually many challenges.
First, How to do machine translation of scarce languages. This is a problem that machine translation has faced since its inception. The smaller the language, the smaller the amount of data, and the scarcity of corpus will be a long-term challenge.
Second, How to do multi-modal machine translation. In recent years, we often need to do voice translation and video translation. In fact, this kind of translation requires AI to do some pre-processing before translation. If AI processing is wrong, translation errors may occur. For another example, in the scenario of simultaneous interpretation, it is usually done while speaking, and complete contextual information cannot be obtained. This is a common problem in multimodal translation.
Third, The most essential problem is that the current machine translation is still based on data-driven and has not gone deeper in understanding. Model learning still relies on the contribution of language rather than true understanding of semantics. This greatly limits the upper limit of machine translation.
51CTO: As a machine translation brand under Bytedance, how does Huoshan Translation deal with the problem of sparse corpus?
Wang Mingxuan: There are two more direct methods.
The first is to expand the corpus and strive to make the scarce corpus "no longer scarce". This idea is to use some models to obtain corpus from the Internet as much as possible. For example, in Icelandic, we can collect a large amount of Icelandic monolingual corpus. On the Internet, we can collect English texts that are similar to the monolingual corpus. We look for such corpus that may be aligned to form bilingual pairs. Of course, we sometimes use manual annotation, but more often we rely on intelligent methods to add it ourselves.
The second is to use the commonality of language. We all live on the same planet. Although we use different languages, we are actually describing the same world. Therefore, languages have many commonalities at a high level. We will use some transfer learning or pre-training methods to solve this type of problem, such as letting the English model help the French model, or letting the German model help the French model. Mainly these two ideas.
51CTO: In order to reduce noise interference in multi-modal machine translation, what strategies has been adopted by Huoshan Translation?
Wang Mingxuan: To deal with noise interference, first of all, we conducted joint modeling of multiple modes. We will use voice signals and text signals together to do downstream tasks, so that error transmission will be reduced a lot. Currently, building multi-modal unified semantics is also a very hot topic in academia, so we will also absorb a lot of things from other fields.
Secondly, we will also do a lot of robustness training in the text area, trying to ensure that the model can still ensure correct output even if there are incorrect inputs, or not amplify this error, which is equivalent to Automatic error correction and machine translation are integrated into one model. Because people actually have this kind of automatic error correction ability. For example, human translators will automatically correct themselves when they hear wrong information, so we will also consider this information in the model.
51CTO: Simultaneous interpretation has very high requirements on delay. However, if there is no contextual context or complete semantics, it is difficult to guarantee accuracy. How does machine translation balance the contradiction between the two?
Wang Mingxuan: This is very challenging in the industrial world, because it is not only about the trade off of delay and accuracy, but actually requires more optimization.
For example, in some conference scenarios, translated subtitles need to be displayed on the big screen. The speed at which the audience accepts the subtitles is also one of the key issues, including the length of each subtitle display and the frequency of subtitle pop-up. How to read more comfortably. There are many details that require us to repeatedly communicate with the product manager and conduct in-depth user surveys to see overall satisfaction. Therefore, This is not just a matter of accuracy. The actual user experience must be taken into consideration before adjusting the model.
In addition, Latency may be one of the indicators of user satisfaction, but the shorter the delay, the better. Usually it is better to have a suitable gap. Because if the delay is very short, the subtitles will pop up very quickly, and the user's acceptance effect will not be very good. In this regard, we will also learn from many mature practices in the industry, such as dynamically controlling the interval of subtitle translation. Overall, this is a very engineering and product-oriented problem.
Future Trends
Machine translation is still not perfect, but practitioners are working hard to make it higher quality, more usable, and more applicable. Let’s take a look at its development trends, especially when machine translation “collides” with professional translators, what chemical reactions will occur in the translation service scene.
51CTO: With the development of technology, will machine translation derive more interesting application scenarios?
Wang Mingxuan: The volcano translation AR glasses we launched before were a similar attempt. The AR translation glasses released at the finale of this year's Google I/O conference are also a very interesting application. After wearing them, users can see the interlocutor's translation in real time, similar to the subtitle effect.
This actually reflects a relatively simple ideal: We hope that everyone can live in a world with barrier-free communication. For example: When traveling abroad, you can understand text prompts in any language by wearing glasses. The street signs you see are in German, but the ones displayed on the glasses are in Chinese. During daily communication, when someone talks to you, the conversation information is automatically turned into text you understand and displayed under the glasses. These are all scenarios where information can be obtained more effectively.
51CTO: In the long run, how will machine translation develop?
Wang Mingxuan: In terms of application, I think machine translation may be more closely integrated with multi-modal applications, such as video content and audio content. The demand for translation will increase. In addition, machine translation may be more associated with overseas business and cultural overseas expansion. Because many domestic companies are actively expanding their overseas business, I think this field will be of great help to the development of machine translation.
In terms of technology, the trends I can see that are already happening are: First, the training of big data and large models. There are more and more people engaged in this field, the models are getting larger and larger, and the amount of data is also increasing. Many people believe that this change may bring about a qualitative change in the capabilities of machine translation. The second is the combination of translation and modality. Not only in terms of translation, many people in the industry are trying to build a unified semantic representation of different modalities. In the past few years, the boundaries between different modalities were relatively clear, and there was relatively little communication. Today, models are increasingly consistent. In the future, there may be a model that can do both text translation, voice translation, and even video translation.
51CTO: In the future, is it possible for machine translation to completely replace human translation in specific scenarios?Wang Mingxuan:
According to the current practice, it certainly cannot replace labor. However, I think machine translation and human translation may not belong to the same track. The characteristics of machine translation are that it is very fast and can be scaled up, so it is suitable for processing massive amounts of information that needs to be processed in a timely manner. For example, if there are 10 million videos that need to be translated from English to French, it is impossible to do it purely manually, but machines can do it. This allows the machine to play a very important role in its track, which is beneficial in the long run, because it broadens the entire market and makes the cross-language market larger.
But for very precise translation scenarios, machine translation may not be able to handle it. As someone mentioned, can machine translation translate "A Dream of Red Mansions"? In my opinion, this does not fall within the scope of machine translation tasks. Translation of novels or poems, this type of translation must rely on experts. There are also high-standard conference simultaneous interpretations, which definitely require professional translators, not machines. But in some meetings that are not very important, the cost advantage of machine translation will be revealed.
Machine translation and professional translators belong to different tracks, and the distinction is still very clear. However, to some extent, the two also have a relationship of mutual help.This is reflected in: On the one hand, the corpus required for machine translation is produced by professional translators. Professional translators continue to produce a large amount of corpus during their work, and these corpus can continue to help machine translation improve its capabilities. On the other hand, machine translation can also help reduce the burden on people and handle less demanding tasks. Nowadays, many translators are doing post-translation editing. Many translation companies let machines do the translation first, and the translators do the editing later. This can greatly improve efficiency. Guest introduction
Wang Mingxuan is the head of ByteDance AI-Lab machine translation team. His research directions are mainly machine translation and natural language processing. In the field of machine translation, he has published more than 40 papers at top conferences such as ACL and EMNLP, and has won first place in international translation evaluation competitions such as WMT many times. At the same time, he also serves as the sponsoring chair of EMNLP2022 and the chair of conference areas such as NeurIPS 2022, NLPCC 2022, and AACL2022.
Column Introduction
"T Frontline" is one of the in-depth interview columns specially opened by the 51CTO Content Center for technical figures. By inviting business people in the technology industry Leaders, senior architects, senior technical experts, etc. provide in-depth interpretation and insight into current technology hot spots, technology practices and technology trends, and promote the dissemination and development of cutting-edge technology.
The above is the detailed content of Exclusive interview with ByteDance Wang Mingxuan: Machine translation and manual translation are essentially two tracks | T Frontline. For more information, please follow other related articles on the PHP Chinese website!

Hot AI Tools

Undresser.AI Undress
AI-powered app for creating realistic nude photos

AI Clothes Remover
Online AI tool for removing clothes from photos.

Undress AI Tool
Undress images for free

Clothoff.io
AI clothes remover

Video Face Swap
Swap faces in any video effortlessly with our completely free AI face swap tool!

Hot Article

Hot Tools

Notepad++7.3.1
Easy-to-use and free code editor

SublimeText3 Chinese version
Chinese version, very easy to use

Zend Studio 13.0.1
Powerful PHP integrated development environment

Dreamweaver CS6
Visual web development tools

SublimeText3 Mac version
God-level code editing software (SublimeText3)

Hot Topics


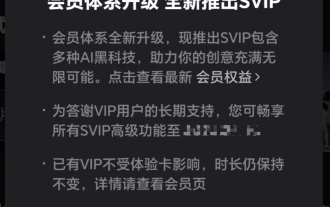
This site reported on June 27 that Jianying is a video editing software developed by FaceMeng Technology, a subsidiary of ByteDance. It relies on the Douyin platform and basically produces short video content for users of the platform. It is compatible with iOS, Android, and Windows. , MacOS and other operating systems. Jianying officially announced the upgrade of its membership system and launched a new SVIP, which includes a variety of AI black technologies, such as intelligent translation, intelligent highlighting, intelligent packaging, digital human synthesis, etc. In terms of price, the monthly fee for clipping SVIP is 79 yuan, the annual fee is 599 yuan (note on this site: equivalent to 49.9 yuan per month), the continuous monthly subscription is 59 yuan per month, and the continuous annual subscription is 499 yuan per year (equivalent to 41.6 yuan per month) . In addition, the cut official also stated that in order to improve the user experience, those who have subscribed to the original VIP
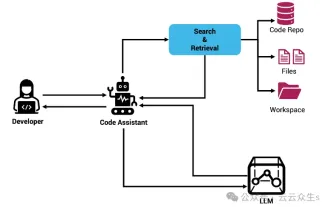
Improve developer productivity, efficiency, and accuracy by incorporating retrieval-enhanced generation and semantic memory into AI coding assistants. Translated from EnhancingAICodingAssistantswithContextUsingRAGandSEM-RAG, author JanakiramMSV. While basic AI programming assistants are naturally helpful, they often fail to provide the most relevant and correct code suggestions because they rely on a general understanding of the software language and the most common patterns of writing software. The code generated by these coding assistants is suitable for solving the problems they are responsible for solving, but often does not conform to the coding standards, conventions and styles of the individual teams. This often results in suggestions that need to be modified or refined in order for the code to be accepted into the application
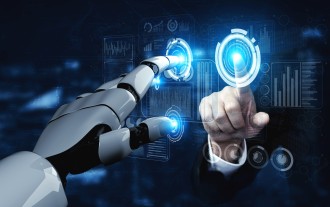
Large Language Models (LLMs) are trained on huge text databases, where they acquire large amounts of real-world knowledge. This knowledge is embedded into their parameters and can then be used when needed. The knowledge of these models is "reified" at the end of training. At the end of pre-training, the model actually stops learning. Align or fine-tune the model to learn how to leverage this knowledge and respond more naturally to user questions. But sometimes model knowledge is not enough, and although the model can access external content through RAG, it is considered beneficial to adapt the model to new domains through fine-tuning. This fine-tuning is performed using input from human annotators or other LLM creations, where the model encounters additional real-world knowledge and integrates it

To learn more about AIGC, please visit: 51CTOAI.x Community https://www.51cto.com/aigc/Translator|Jingyan Reviewer|Chonglou is different from the traditional question bank that can be seen everywhere on the Internet. These questions It requires thinking outside the box. Large Language Models (LLMs) are increasingly important in the fields of data science, generative artificial intelligence (GenAI), and artificial intelligence. These complex algorithms enhance human skills and drive efficiency and innovation in many industries, becoming the key for companies to remain competitive. LLM has a wide range of applications. It can be used in fields such as natural language processing, text generation, speech recognition and recommendation systems. By learning from large amounts of data, LLM is able to generate text
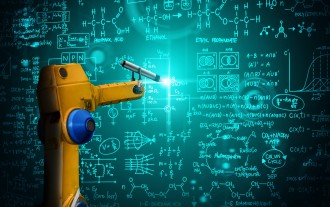
Machine learning is an important branch of artificial intelligence that gives computers the ability to learn from data and improve their capabilities without being explicitly programmed. Machine learning has a wide range of applications in various fields, from image recognition and natural language processing to recommendation systems and fraud detection, and it is changing the way we live. There are many different methods and theories in the field of machine learning, among which the five most influential methods are called the "Five Schools of Machine Learning". The five major schools are the symbolic school, the connectionist school, the evolutionary school, the Bayesian school and the analogy school. 1. Symbolism, also known as symbolism, emphasizes the use of symbols for logical reasoning and expression of knowledge. This school of thought believes that learning is a process of reverse deduction, through existing
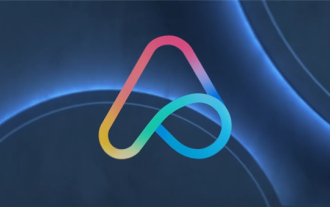
According to news on June 13, according to Byte's "Volcano Engine" public account, Xiaomi's artificial intelligence assistant "Xiao Ai" has reached a cooperation with Volcano Engine. The two parties will achieve a more intelligent AI interactive experience based on the beanbao large model. It is reported that the large-scale beanbao model created by ByteDance can efficiently process up to 120 billion text tokens and generate 30 million pieces of content every day. Xiaomi used the beanbao large model to improve the learning and reasoning capabilities of its own model and create a new "Xiao Ai Classmate", which not only more accurately grasps user needs, but also provides faster response speed and more comprehensive content services. For example, when a user asks about a complex scientific concept, &ldq
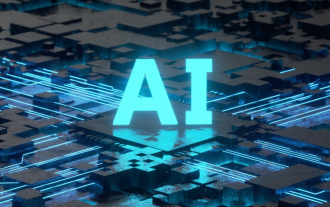
Editor |ScienceAI Question Answering (QA) data set plays a vital role in promoting natural language processing (NLP) research. High-quality QA data sets can not only be used to fine-tune models, but also effectively evaluate the capabilities of large language models (LLM), especially the ability to understand and reason about scientific knowledge. Although there are currently many scientific QA data sets covering medicine, chemistry, biology and other fields, these data sets still have some shortcomings. First, the data form is relatively simple, most of which are multiple-choice questions. They are easy to evaluate, but limit the model's answer selection range and cannot fully test the model's ability to answer scientific questions. In contrast, open-ended Q&A
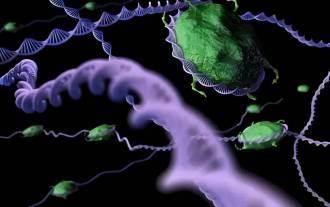
Editor | KX In the field of drug research and development, accurately and effectively predicting the binding affinity of proteins and ligands is crucial for drug screening and optimization. However, current studies do not take into account the important role of molecular surface information in protein-ligand interactions. Based on this, researchers from Xiamen University proposed a novel multi-modal feature extraction (MFE) framework, which for the first time combines information on protein surface, 3D structure and sequence, and uses a cross-attention mechanism to compare different modalities. feature alignment. Experimental results demonstrate that this method achieves state-of-the-art performance in predicting protein-ligand binding affinities. Furthermore, ablation studies demonstrate the effectiveness and necessity of protein surface information and multimodal feature alignment within this framework. Related research begins with "S
