


OpenAI uses GPT-4 to explain GPT-2's 300,000 neurons: This is what wisdom looks like
May 25, 2023 pm 12:04 PMAlthough ChatGPT seems to bring humans closer to recreating intelligence, so far we have never fully understood what intelligence is, whether natural or artificial.
It is obviously necessary to understand the principles of intelligence. How to understand the intelligence of large language models? The solution given by OpenAI is: ask what GPT-4 says.
On May 9, OpenAI released its latest research, which used GPT-4 to automatically interpret neuron behavior in large language models and obtained many interesting results.
A simple way to study interpretability is to first understand the various components of the AI model (neurons and attention heads) )doing what. Traditional methods require humans to manually inspect neurons to determine which features of the data they represent. This process is difficult to scale, and applying it to neural networks with hundreds or hundreds of billions of parameters is prohibitively expensive.
So OpenAI proposed an automated method - using GPT-4 to generate and score natural language explanations of neuron behavior and apply it to another language Neurons in the model - Here they chose GPT-2 as the experimental sample and published a data set of interpretations and scores of these GPT-2 neurons.
- Paper address: https://openaipublic.blob.core.windows.net/ neuron-explainer/paper/index.html
- GPT-2 neuron diagram: https://openaipublic.blob.core.windows.net/neuron- explainer/neuron-viewer/index.html
- Code and dataset: https://github.com/openai/automated-interpretability
This technology allows people to use GPT-4 to define and automatically measure the quantitative concept of explainability of AI models: it is used to measure language models using natural language compression and reconstruction The ability of neurons to activate. Due to their quantitative nature, we can now measure progress in understanding the computational goals of neural networks.
OpenAI said that using the benchmark they established, using AI to explain AI can achieve scores close to human levels.
## OpenAI co-founder Greg Brockman also said that we have taken an important step towards using AI to automate alignment research.
Specific methodThe method of using AI to explain AI involves running three steps on each neuron:
Step 1: Use GPT-4 to generate explanations
Explanations of model generation: References to movies, characters, and entertainment.
Step 2: Use GPT-4 to simulate
Use GPT-4 again to simulate the interpreted neural What will Yuan do.
Step 3: Comparison
Explanations are scored based on how well simulated activations match real activations - in this case, GPT-4 scored 0.34.
Using its own scoring method, OpenAI began measuring the effectiveness of their technology on different parts of the network and trying to improve the technology for parts that are currently unclear. For example, their technique does not work well with larger models, possibly because later layers are more difficult to interpret.
OpenAI says that while the vast majority of their explanations didn’t score highly, they believe they can now use ML technology to further enhance their ability to generate explanations. For example, they found that the following helped improve their scores:
- Iterative explanations. They could improve their scores by asking GPT-4 to think of possible counterexamples and then modify the explanation based on their activation.
- Use a larger model for explanation. As the ability of the explainer model improves, the average score will also increase. However, even GPT-4 gave worse explanations than humans, suggesting there is room for improvement.
- Change the structure of the explained model. Training the model with different activation functions improves the explanation score.
OpenAI says it is making open source the dataset and visualization tools written by GPT-4 that interpret all 307,200 neurons in GPT-2. At the same time, they also provide code for interpretation and scoring using models publicly available on the OpenAI API. They hope the research community will develop new techniques to generate higher-scoring explanations, as well as better tools to explore GPT-2 through explanations.
They found that more than 1,000 neurons had an explanation score of at least 0.8, meaning they accounted for most of the neuron's top activation behavior according to GPT-4. Most of these well-explained neurons are not very interesting. However, they also found many interesting neurons that GPT-4 did not understand. OpenAI hopes that as explanations improve, they may quickly uncover interesting qualitative insights into model computations.
Here are some examples of neurons being activated in different layers, with higher layers being more abstract:
Future work of OpenAI
Currently, this method still has some limitations, and OpenAI hopes to solve these problems in future work:- This method focuses on short natural language explanations, but neurons may have very complex behaviors that cannot be described concisely;
- OpenAI The hope is to eventually automatically find and interpret entire neural circuits to achieve complex behaviors, with neurons and attention heads working together. Current methods simply interpret the behavior of neurons as a function of raw text input without accounting for its downstream effects. For example, a neuron that fires on a period could indicate that the next word should start with a capital letter, or increment a sentence counter;
- #OpenAI explains this behavior of neurons , without attempting to explain the mechanism that produces this behavior. This means that even high-scoring explanations may perform poorly on out-of-distribution texts because they simply describe a correlation;
- The whole process consumes a lot of computing power.
Ultimately, OpenAI hopes to use models to form, test, and iterate completely general hypotheses, just as explainability researchers do. Additionally, OpenAI hopes to interpret its largest models as a way to detect alignment and security issues before and after deployment. However, there is still a long way to go before that happens.
The above is the detailed content of OpenAI uses GPT-4 to explain GPT-2's 300,000 neurons: This is what wisdom looks like. For more information, please follow other related articles on the PHP Chinese website!

Hot Article

Hot tools Tags

Hot Article

Hot Article Tags

Notepad++7.3.1
Easy-to-use and free code editor

SublimeText3 Chinese version
Chinese version, very easy to use

Zend Studio 13.0.1
Powerful PHP integrated development environment

Dreamweaver CS6
Visual web development tools

SublimeText3 Mac version
God-level code editing software (SublimeText3)

Hot Topics
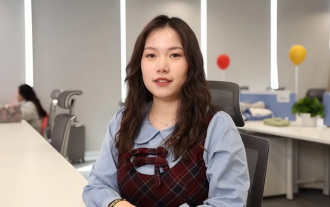
Behind the first Android access to DeepSeek: Seeing the power of women
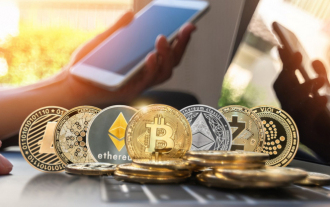
The latest ranking of the top ten trading apps in 2025
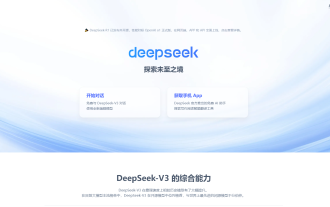
How to solve the problem of busy servers for deepseek
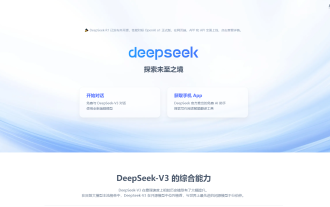
deepseek web version official entrance
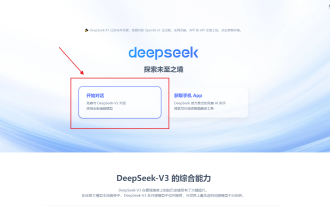
In-depth search deepseek official website entrance
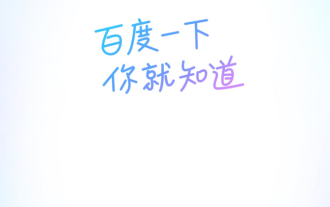
Another national product from Baidu is connected to DeepSeek. Is it open or follow the trend?
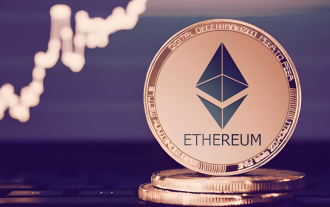
Top 10 recommended for crypto digital asset trading APP (2025 global ranking)
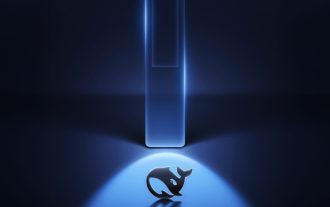
Midea launches its first DeepSeek air conditioner: AI voice interaction can achieve 400,000 commands!
