How does ChatGPT output word by word?
#When ChatGPT answers a question, it pops up word by word, giving people the feeling that they are thinking seriously.
The basic content of this conversation is:
Q: How does the large language generative model work
Answer: Large-scale language generation models (such as GPT-3) are neural network models based on deep learning technology. These models often use an architecture called a "Transformer", which has achieved great success in the field of natural language processing.Here is an overview of how a large language generation model works:
... Omit a paragraph...
Generate text: Once the model is trained, it can be used to generate text. Given an initial text or prompt, the model generates the next word based on the probability distribution it has learned, then uses the generated word as input and continues to generate the next word, and so on until the desired text length is reached or generated Termination symbol.
... Omit a paragraph...
Large Language Model, Large Language Model, referred to as LLM.
From a model perspective, LLM generates a token each time it performs inference until the text length limit is reached or a terminator is generated.
From the server's perspective, the generated tokens need to be returned to the browser one by one through the HTTPS protocol.
In Client-Server mode, the conventional interaction method is that the client sends a request and receives a response. Obviously, this cannot meet the scenario of ChatGPT replying to questions.
Secondly, we may think of websocket, which relies on HTTP to implement handshake and is upgraded to WebSocket. However, WebSocket requires both the client and the server to continuously occupy a socket, and the cost on the server side is relatively high.
ChatGPT uses a compromise: server-sent event (SSE for short). We can find this from OpenAI’s API documentation:
In SSE mode, the client only needs to send a request to the server once, and the server can continue to output until the end is required. The entire interaction process is shown in the figure below:
SSE still uses HTTP as the application layer transmission protocol, making full use of HTTP's long connection capability to implement server-side Push capability.
From a code perspective, the differences between SSE mode and a single HTTP request are:
- The client needs to turn on keep -alive ensures that the connection will not time out.
- The HTTP response header contains Content-Type=text/event-stream, Cache-Cnotallow=no-cache, etc.
- The body of the HTTP response is generally a structure like "data: ...".
- The HTTP response may contain some empty data to avoid connection timeout.
Take the ChatGPT API as an example. When sending a request, setting the stream parameter to true enables the SSE feature, but you need to pay attention to the SDK that reads the data. .
In normal mode, after getting http.Response, use ioutil.ReadAll to read the data. code show as below:
func main() {payload := strings.NewReader(`{"model": "gpt-3.5-turbo","messages": [{"role": "user", "content": "大语言生成式模型是如何工作的"}],"max_tokens": 1024,"temperature": 1,"top_p": 1,"n": 1,"stream": false}`)client := &http.Client{}req, _ := http.NewRequest("POST", "https://api.openai.com/v1/chat/completions", payload)req.Header.Add("Content-Type", "application/json")req.Header.Add("Authorization", "Bearer <openai-token>")resp, err := client.Do(req)if err != nil {fmt.Println(err)return}defer resp.Body.Close()body, _ := ioutil.ReadAll(resp.Body)fmt.Println(string(body))}</openai-token>
It takes about 20s to execute and get a complete result:
{"id": "chatcmpl-7KklTf9mag5tyBXLEqM3PWQn4jlfD","object": "chat.completion","created": 1685180679,"model": "gpt-3.5-turbo-0301","usage": {"prompt_tokens": 21,"completion_tokens": 358,"total_tokens": 379},"choices": [{"message": {"role": "assistant","content": "大语言生成式模型通常采用神经网络来实现,具体工作流程如下:\n\n1. 数据预处理:将语料库中的文本数据进行预处理,包括分词、删除停用词(如“的”、“了”等常用词汇)、去重等操作,以减少冗余信息。\n\n2. 模型训练:采用递归神经网络(RNN)、长短期记忆网络(LSTM)或变种的Transformers等模型进行训练,这些模型都具有一定的记忆能力,可以学习到语言的一定规律,并预测下一个可能出现的词语。\n\n3. 模型应用:当模型完成训练后,可以将其应用于实际的生成任务中。模型接收一个输入文本串,并预测下一个可能出现的词语,直到达到一定长度或遇到结束符号为止。\n\n4. 根据生成结果对模型进行调优:生成的结果需要进行评估,如计算生成文本与语料库文本的相似度、流畅度等指标,以此来调优模型,提高其生成质量。\n\n总体而言,大语言生成式模型通过对语言的规律学习,从而生成高质量的文本。"},"finish_reason": "stop","index": 0}]}
If we set stream to true without making any modifications, the total request consumption is 28s, which reflects For many stream messages:
The above picture is a picture of Postman calling the chatgpt api, using the ioutil.ReadAll mode. In order to implement stream reading, we can read http.Response.Body in segments. Here's why this works:
- http.Response.Body is of type io.ReaderCloser , the bottom layer relies on an HTTP connection and supports stream reading.
- The data returned by SSE is split by newline characters\n
So the correction method is to pass bufio.NewReader(resp.Body)Wrap it up and read it in a for-loop. The code is as follows:
// stream event 结构体定义type ChatCompletionRspChoiceItem struct {Deltamap[string]string `json:"delta,omitempty"` // 只有 content 字段Indexint `json:"index,omitempty"`Logprobs *int`json:"logprobs,omitempty"`FinishReason string`json:"finish_reason,omitempty"`}type ChatCompletionRsp struct {IDstring`json:"id"`Objectstring`json:"object"`Created int `json:"created"` // unix secondModel string`json:"model"`Choices []ChatCompletionRspChoiceItem `json:"choices"`}func main() {payload := strings.NewReader(`{"model": "gpt-3.5-turbo","messages": [{"role": "user", "content": "大语言生成式模型是如何工作的"}],"max_tokens": 1024,"temperature": 1,"top_p": 1,"n": 1,"stream": true}`)client := &http.Client{}req, _ := http.NewRequest("POST", "https://api.openai.com/v1/chat/completions", payload)req.Header.Add("Content-Type", "application/json")req.Header.Add("Authorization", "Bearer "+apiKey)req.Header.Set("Accept", "text/event-stream")req.Header.Set("Cache-Control", "no-cache")req.Header.Set("Connection", "keep-alive")resp, err := client.Do(req)if err != nil {fmt.Println(err)return}defer resp.Body.Close()reader := bufio.NewReader(resp.Body)for {line, err := reader.ReadBytes('\n')if err != nil {if err == io.EOF {// 忽略 EOF 错误break} else {if netErr, ok := err.(net.Error); ok && netErr.Timeout() {fmt.Printf("[PostStream] fails to read response body, timeout\n")} else {fmt.Printf("[PostStream] fails to read response body, err=%s\n", err)}}break}line = bytes.TrimSuffix(line, []byte{'\n'})line = bytes.TrimPrefix(line, []byte("data: "))if bytes.Equal(line, []byte("[DONE]")) {break} else if len(line) > 0 {var chatCompletionRsp ChatCompletionRspif err := json.Unmarshal(line, &chatCompletionRsp); err == nil {fmt.Printf(chatCompletionRsp.Choices[0].Delta["content"])} else {fmt.Printf("\ninvalid line=%s\n", line)}}}fmt.Println("the end")}
After reading the client side, let’s look at the server side . Now we try to mock chatgpt server and return a piece of text verbatim. Two points are involved here:
- Response Header needs to set Connection to keep-alive and Content-Type to text/event-stream.
- After writing respnose, it needs to be flushed to the client.
The code is as follows:
func streamHandler(w http.ResponseWriter, req *http.Request) {w.Header().Set("Connection", "keep-alive")w.Header().Set("Content-Type", "text/event-stream")w.Header().Set("Cache-Control", "no-cache")var chatCompletionRsp ChatCompletionRsprunes := []rune(`大语言生成式模型通常使用深度学习技术,例如循环神经网络(RNN)或变压器(Transformer)来建模语言的概率分布。这些模型接收前面的词汇序列,并利用其内部神经网络结构预测下一个词汇的概率分布。然后,模型将概率最高的词汇作为生成的下一个词汇,并递归地生成一个词汇序列,直到到达最大长度或遇到一个终止符号。在训练过程中,模型通过最大化生成的文本样本的概率分布来学习有效的参数。为了避免模型产生过于平凡的、重复的、无意义的语言,我们通常会引入一些技巧,如dropout、序列扰动等。大语言生成模型的重要应用包括文本生成、问答系统、机器翻译、对话建模、摘要生成、文本分类等。`)for _, r := range runes {chatCompletionRsp.Choices = []ChatCompletionRspChoiceItem{{Delta: map[string]string{"content": string(r)}},}bs, _ := json.Marshal(chatCompletionRsp)line := fmt.Sprintf("data: %s\n", bs)fmt.Fprintf(w, line)if f, ok := w.(http.Flusher); ok {f.Flush()}time.Sleep(time.Millisecond * 100)}fmt.Fprintf(w, "data: [DONE]\n")}func main() {http.HandleFunc("/stream", streamHandler)http.ListenAndServe(":8088", nil)}
In a real scenario, the data to be returned comes from another service or function call. If The return time of this service or function call is unstable, which may cause the client to not receive messages for a long time, so the general processing method is:
- The call to the third party is placed in in a goroutine.
- Create a timer through time.Tick and send an empty message to the client.
- Create a timeout channel to avoid too long response time.
In order to read data from different channels, select is a good keyword, such as this demo code:
// 声明一个 event channel// 声明一个 time.Tick channel// 声明一个 timeout channelselect {case ev := <h2 id="Summary-The-process-of-generating-and-responding-to-the-entire-result-of-the-large-language-model-is-relatively-long-but-the-response-generated-token-by-token-is-relatively-fast-ChatGPT-fully-combines-this-feature-with-SSE-technology-to-pop-up-word-by-word-Reply-has-achieved-a-qualitative-improvement-in-user-experience">Summary The process of generating and responding to the entire result of the large language model is relatively long, but the response generated token by token is relatively fast. ChatGPT fully combines this feature with SSE technology to pop up word by word. Reply has achieved a qualitative improvement in user experience. </h2><p style="text-align: justify;"><span style="color: #333333;"></span>Looking at generative models, whether it is LLAMA/Little Alpaca (not commercially available) or Stable Diffusion/Midjourney. When providing online services, SSE technology can be used to improve user experience and save server resources. </p><p style="text-align: justify;"></p>
The above is the detailed content of How does ChatGPT output word by word?. For more information, please follow other related articles on the PHP Chinese website!

Hot AI Tools

Undresser.AI Undress
AI-powered app for creating realistic nude photos

AI Clothes Remover
Online AI tool for removing clothes from photos.

Undress AI Tool
Undress images for free

Clothoff.io
AI clothes remover

Video Face Swap
Swap faces in any video effortlessly with our completely free AI face swap tool!

Hot Article

Hot Tools

Notepad++7.3.1
Easy-to-use and free code editor

SublimeText3 Chinese version
Chinese version, very easy to use

Zend Studio 13.0.1
Powerful PHP integrated development environment

Dreamweaver CS6
Visual web development tools

SublimeText3 Mac version
God-level code editing software (SublimeText3)

Hot Topics


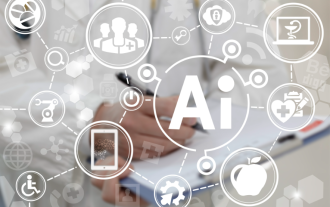
Translator | Bugatti Review | Chonglou This article describes how to use the GroqLPU inference engine to generate ultra-fast responses in JanAI and VSCode. Everyone is working on building better large language models (LLMs), such as Groq focusing on the infrastructure side of AI. Rapid response from these large models is key to ensuring that these large models respond more quickly. This tutorial will introduce the GroqLPU parsing engine and how to access it locally on your laptop using the API and JanAI. This article will also integrate it into VSCode to help us generate code, refactor code, enter documentation and generate test units. This article will create our own artificial intelligence programming assistant for free. Introduction to GroqLPU inference engine Groq

To learn more about AIGC, please visit: 51CTOAI.x Community https://www.51cto.com/aigc/Translator|Jingyan Reviewer|Chonglou is different from the traditional question bank that can be seen everywhere on the Internet. These questions It requires thinking outside the box. Large Language Models (LLMs) are increasingly important in the fields of data science, generative artificial intelligence (GenAI), and artificial intelligence. These complex algorithms enhance human skills and drive efficiency and innovation in many industries, becoming the key for companies to remain competitive. LLM has a wide range of applications. It can be used in fields such as natural language processing, text generation, speech recognition and recommendation systems. By learning from large amounts of data, LLM is able to generate text
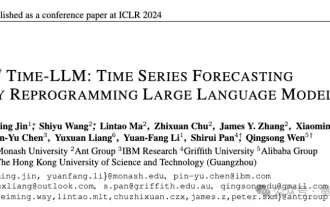
The potential of large language models is stimulated - high-precision time series prediction can be achieved without training large language models, surpassing all traditional time series models. Monash University, Ant and IBM Research jointly developed a general framework that successfully promoted the ability of large language models to process sequence data across modalities. The framework has become an important technological innovation. Time series prediction is beneficial to decision-making in typical complex systems such as cities, energy, transportation, and remote sensing. Since then, large models are expected to revolutionize time series/spatiotemporal data mining. The general large language model reprogramming framework research team proposed a general framework to easily use large language models for general time series prediction without any training. Two key technologies are mainly proposed: timing input reprogramming; prompt prefixing. Time-
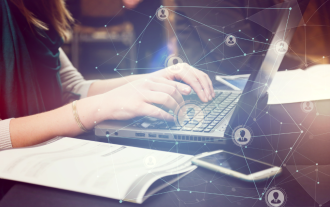
This article will open source the results of "Local Deployment of Large Language Models in OpenHarmony" demonstrated at the 2nd OpenHarmony Technology Conference. Open source address: https://gitee.com/openharmony-sig/tpc_c_cplusplus/blob/master/thirdparty/InferLLM/docs/ hap_integrate.md. The implementation ideas and steps are to transplant the lightweight LLM model inference framework InferLLM to the OpenHarmony standard system, and compile a binary product that can run on OpenHarmony. InferLLM is a simple and efficient L
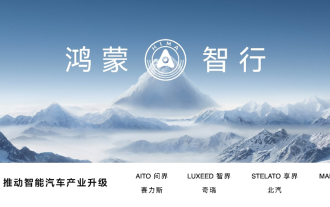
This afternoon, Hongmeng Zhixing officially welcomed new brands and new cars. On August 6, Huawei held the Hongmeng Smart Xingxing S9 and Huawei full-scenario new product launch conference, bringing the panoramic smart flagship sedan Xiangjie S9, the new M7Pro and Huawei novaFlip, MatePad Pro 12.2 inches, the new MatePad Air, Huawei Bisheng With many new all-scenario smart products including the laser printer X1 series, FreeBuds6i, WATCHFIT3 and smart screen S5Pro, from smart travel, smart office to smart wear, Huawei continues to build a full-scenario smart ecosystem to bring consumers a smart experience of the Internet of Everything. Hongmeng Zhixing: In-depth empowerment to promote the upgrading of the smart car industry Huawei joins hands with Chinese automotive industry partners to provide
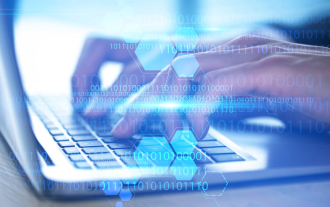
Large language models (LLMs) demonstrate impressive performance in language understanding and various reasoning tasks. However, their role in spatial reasoning, a key aspect of human cognition, remains understudied. Humans have the ability to create mental images of unseen objects and actions through a process known as the mind's eye, making it possible to imagine the unseen world. Inspired by this cognitive ability, researchers proposed "Visualization of Thought" (VoT). VoT aims to guide the spatial reasoning of LLMs by visualizing their reasoning signs, thereby guiding subsequent reasoning steps. Researchers apply VoT to multi-hop spatial reasoning tasks, including natural language navigation, vision
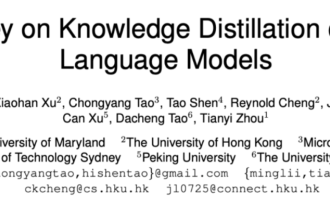
Large Language Models (LLMs) have developed rapidly in the past two years, and some phenomenal models and products have emerged, such as GPT-4, Gemini, Claude, etc., but most of them are closed source. There is a large gap between most open source LLMs currently accessible to the research community and closed source LLMs. Therefore, improving the capabilities of open source LLMs and other small models to reduce the gap between them and closed source large models has become a research hotspot in this field. The powerful capabilities of LLM, especially closed-source LLM, enable scientific researchers and industrial practitioners to utilize the output and knowledge of these large models when training their own models. This process is essentially knowledge distillation (Knowledge, Dist
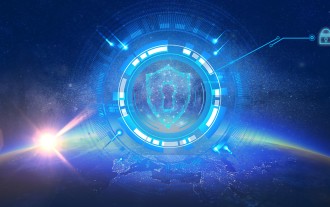
The biggest risk currently faced by artificial intelligence technology is that the development and application speed of large language models (LLM) and generative artificial intelligence technology have far exceeded the speed of security and governance. Use of generative AI and large language model products from companies like OpenAI, Anthropic, Google, and Microsoft is growing exponentially. At the same time, open source large language model solutions are also growing rapidly. Open source artificial intelligence communities such as HuggingFace provide a large number of open source models, data sets and AI applications. In order to promote the development of artificial intelligence, industry organizations such as OWASP, OpenSSF, and CISA are actively developing and providing key assets for artificial intelligence security and governance, such as OWASPAIExchange,
