


Open-world understanding of 3D point clouds, classification, retrieval, subtitles and image generation
Input the three-dimensional shapes of a rocking chair and a horse. What can you get?
##Wooden cart plus horse? Get a carriage and an electric horse; a banana and a sailboat? Get a banana sailboat; eggs plus deck chairs? Get the egg chair.
Researchers from UCSD, Shanghai Jiao Tong University, and Qualcomm teams have proposed the latest three-dimensional representation model OpenShape, making it possible to understand the open world of three-dimensional shapes.
- Paper address: https://arxiv.org/pdf/2305.10764.pdf
- Project homepage: https://colin97.github.io/OpenShape/
- Interactive demo: https://huggingface.co/spaces/OpenShape/openshape-demo
- Code address: https://github.com/Colin97/OpenShape_code
By learning a native encoder of 3D point clouds on multi-modal data (point cloud - text - image), OpenShape builds a representation space of 3D shapes and aligns it with CLIP's text and image spaces. Thanks to large-scale and diverse 3D pre-training, OpenShape achieves open-world understanding of 3D shapes for the first time, supporting zero-shot 3D shape classification, multi-modal 3D shape retrieval (text/image/point cloud input), and subtitles of 3D point clouds. Cross-modal tasks such as image generation and 3D point cloud-based image generation.
Three-dimensional shape zero-shot classificationOpenShape’s top3 and top5 accuracy on ModelNet40 reached 96.5% and 98.0% respectively.
Multimodal 3D shape retrieval
With OpenShape’s multimodal representation, users can perform 3D shape retrieval on image, text, or point cloud input. Study the retrieval of 3D shapes from integrated datasets by computing the cosine similarity between the input representation and the 3D shape representation and finding kNN.
The above figure shows the input image and two retrieved 3D shapes. Three-dimensional shape retrieval of text input The above image shows the input text and the retrieved three-dimensional shape. OpenShape learns a wide range of visual and semantic concepts, enabling fine-grained subcategory (first two lines) and attribute control (last two lines, such as color, shape, style, and their combinations). 3D shape retrieval of 3D point cloud input The above figure shows the input 3D point cloud and two retrieved 3D shapes.
#Double input three-dimensional shape retrieval
The above figure takes two 3D shapes as input and uses their OpenShape representations to retrieve the 3D shape that is closest to both inputs at the same time. The retrieved shape cleverly combines semantic and geometric elements from both input shapes.
Three-dimensional shape-based text and image generationBecause OpenShape's three-dimensional shape representation is aligned with CLIP's image and text representation space, they can be used with many based on Derived models from CLIP are combined to support a variety of cross-modal applications.
Subtitle generation of three-dimensional point cloud
By combining with the ready-made image subtitle model (ClipCap), OpenShape implements subtitle generation for 3D point clouds.
Image generation based on three-dimensional point cloud
By combining with the ready-made text-to-image diffusion model (Stable unCLIP), OpenShape implements image generation based on 3D point clouds (supporting optional text hints).
##More examples of image generation based on three-dimensional point clouds Training details
Multimodal representation alignment based on contrastive learning: OpenShape trains a 3D native encoder that will The 3D point cloud is used as input to extract the representation of the 3D shape. Following previous work, we exploit multimodal contrastive learning to align with CLIP's image and text representation spaces. Unlike previous work, OpenShape aims to learn a more general and scalable joint representation space. The focus of the research is mainly to expand the scale of 3D representation learning and address the corresponding challenges, so as to truly realize 3D shape understanding in the open world.
Integrating multiple 3D shape datasets: Since the scale and diversity of training data play a crucial role in learning large-scale 3D shape representations, the study integrated four Training on the currently largest publicly available 3D dataset. As shown in the figure below, the training data studied contains 876,000 training shapes. Among the four datasets, ShapeNetCore, 3D-FUTURE, and ABO contain high-quality human-verified 3D shapes, but only cover a limited number of shapes and dozens of categories. The Objaverse dataset is a recently released 3D dataset that contains significantly more 3D shapes and covers a more diverse object class. However, the shapes in the Objaverse are mainly uploaded by Internet users and have not been manually verified. Therefore, the quality is uneven and the distribution is extremely uneven, requiring further processing.
Text filtering and enrichment: Study found only between 3D shapes and 2D images Applying contrastive learning is insufficient to drive alignment of 3D shape and text spaces, even when trained on large-scale datasets. Research speculates that this is due to the inherent domain gap in CLIP's language and image representation spaces. Therefore, research needs to explicitly align 3D shapes with text. However, text annotations from original 3D data sets often face problems such as missing, wrong, or rough and single content. To this end, this paper proposes three strategies to filter and enrich text to improve the quality of text annotation: text filtering using GPT-4, subtitle generation and image retrieval of 2D renderings of 3D models.
#The study proposed three strategies to automatically Filter and enrich noisy text in raw datasets.
##Text filtering and enrichment examples
In each example, the left section shows the thumbnail, original shape name, and GPT-4 filtered results. The upper right part shows the image captions from the two captioning models, while the lower right part shows the retrieved image and its corresponding text.
Expand the three-dimensional backbone network. Since previous work on 3D point cloud learning mainly targeted small-scale 3D data sets like ShapeNet, these backbone networks may not be directly applicable to our large-scale 3D training, and the scale of the backbone network needs to be expanded accordingly. The study found that different 3D backbone networks exhibited different behaviors and scalability when trained on data sets of different sizes. Among them, PointBERT based on Transformer and SparseConv based on three-dimensional convolution show more powerful performance and scalability, so they were selected as the three-dimensional backbone network.
When scaling up the size of the 3D backbone model on the integrated dataset, the Performance and scalability comparison.
Difficult Negative Example Mining: The ensemble dataset of this study exhibits a high degree of class imbalance. Some common categories, like architecture, may occupy tens of thousands of shapes, while many other categories, like walruses and wallets, are underrepresented with only a few dozen or even fewer shapes. Therefore, when batches are randomly constructed for contrastive learning, shapes from two easily confused categories (e.g., apples and cherries) are unlikely to appear in the same batch to be contrasted. To this end, this paper proposes an offline difficult negative example mining strategy to improve training efficiency and performance.
Welcome to try the interactive demo on HuggingFace.The above is the detailed content of Open-world understanding of 3D point clouds, classification, retrieval, subtitles and image generation. For more information, please follow other related articles on the PHP Chinese website!

Hot AI Tools

Undresser.AI Undress
AI-powered app for creating realistic nude photos

AI Clothes Remover
Online AI tool for removing clothes from photos.

Undress AI Tool
Undress images for free

Clothoff.io
AI clothes remover

AI Hentai Generator
Generate AI Hentai for free.

Hot Article

Hot Tools

Notepad++7.3.1
Easy-to-use and free code editor

SublimeText3 Chinese version
Chinese version, very easy to use

Zend Studio 13.0.1
Powerful PHP integrated development environment

Dreamweaver CS6
Visual web development tools

SublimeText3 Mac version
God-level code editing software (SublimeText3)

Hot Topics


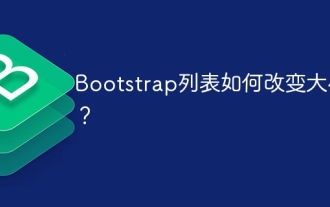
The size of a Bootstrap list depends on the size of the container that contains the list, not the list itself. Using Bootstrap's grid system or Flexbox can control the size of the container, thereby indirectly resizing the list items.
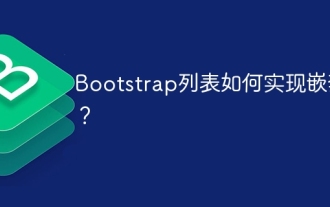
Nested lists in Bootstrap require the use of Bootstrap's grid system to control the style. First, use the outer layer <ul> and <li> to create a list, then wrap the inner layer list in <div class="row> and add <div class="col-md-6"> to the inner layer list to specify that the inner layer list occupies half the width of a row. In this way, the inner list can have the right one
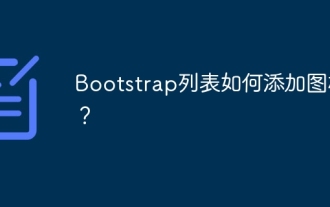
How to add icons to the Bootstrap list: directly stuff the icon into the list item <li>, using the class name provided by the icon library (such as Font Awesome). Use the Bootstrap class to align icons and text (for example, d-flex, justify-content-between, align-items-center). Use the Bootstrap tag component (badge) to display numbers or status. Adjust the icon position (flex-direction: row-reverse;), control the style (CSS style). Common error: The icon does not display (not
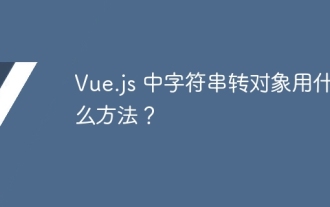
When converting strings to objects in Vue.js, JSON.parse() is preferred for standard JSON strings. For non-standard JSON strings, the string can be processed by using regular expressions and reduce methods according to the format or decoded URL-encoded. Select the appropriate method according to the string format and pay attention to security and encoding issues to avoid bugs.
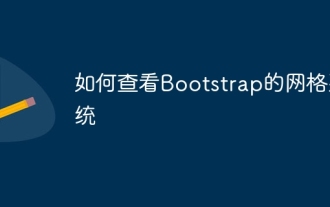
Bootstrap's mesh system is a rule for quickly building responsive layouts, consisting of three main classes: container (container), row (row), and col (column). By default, 12-column grids are provided, and the width of each column can be adjusted through auxiliary classes such as col-md-, thereby achieving layout optimization for different screen sizes. By using offset classes and nested meshes, layout flexibility can be extended. When using a grid system, make sure that each element has the correct nesting structure and consider performance optimization to improve page loading speed. Only by in-depth understanding and practice can we master the Bootstrap grid system proficiently.
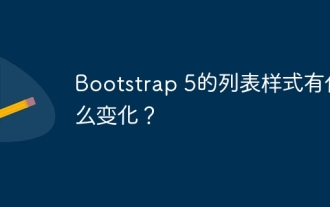
Bootstrap 5 list style changes are mainly due to detail optimization and semantic improvement, including: the default margins of unordered lists are simplified, and the visual effects are cleaner and neat; the list style emphasizes semantics, enhancing accessibility and maintainability.
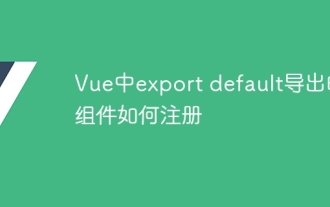
Question: How to register a Vue component exported through export default? Answer: There are three registration methods: Global registration: Use the Vue.component() method to register as a global component. Local Registration: Register in the components option, available only in the current component and its subcomponents. Dynamic registration: Use the Vue.component() method to register after the component is loaded.
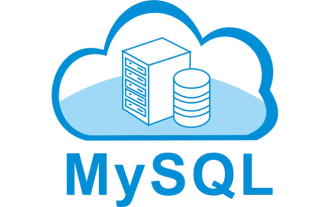
MySQL performance optimization needs to start from three aspects: installation configuration, indexing and query optimization, monitoring and tuning. 1. After installation, you need to adjust the my.cnf file according to the server configuration, such as the innodb_buffer_pool_size parameter, and close query_cache_size; 2. Create a suitable index to avoid excessive indexes, and optimize query statements, such as using the EXPLAIN command to analyze the execution plan; 3. Use MySQL's own monitoring tool (SHOWPROCESSLIST, SHOWSTATUS) to monitor the database health, and regularly back up and organize the database. Only by continuously optimizing these steps can the performance of MySQL database be improved.
