Association rule mining techniques in Python
As a powerful programming language, Python can be applied in various fields, including data mining and machine learning. In the field of data mining, association rule mining is a commonly used technique that can be used to discover relationships between different items in a data set and the impact of these relationships on other things. This article will briefly introduce association rule mining techniques in Python.
- Apriori algorithm
The Apriori algorithm is a classic algorithm in the field of association rule mining, which can be used to discover frequent item sets and association rules in data sets. Frequent itemsets refer to the set of items that appear more frequently in the data set, while association rules refer to the relationship between two or more items. They may appear at the same time, or the occurrence of one means that the other is also likely to appear. .
You can use the apriori function in the mlxtend library to implement the Apriori algorithm in Python. The following is a simple sample code:
from mlxtend.frequent_patterns import apriori # 构建数据集 data = [['牛奶', '面包', '啤酒'], ['奶酪', '面包', '黄油'], ['牛奶', '面包', '黄油', '鸡蛋'], ['奶酪', '黄油', '鸡蛋'], ['面包', '啤酒']] # 使用apriori算法挖掘频繁项集 frequent_itemsets = apriori(data, min_support=0.6) # 输出频繁项集 print(frequent_itemsets)
In the above code, we first define a data set, which contains the contents of five shopping baskets. Then use the apriori function in the mlxtend library to mine frequent itemsets. The first parameter of the function is the data set, and the second parameter is the minimum support threshold, which is set to 0.6 here.
In the output result, we can see that the algorithm found two frequent item sets: ['Bread'] and ['Milk', 'Bread']. This means that in this data set, the largest number of people buy bread, followed by milk and bread. We can discover frequent itemsets of different sizes by adjusting the support threshold.
- Extraction of association rules
After discovering frequent itemsets, we can continue to extract association rules. Association rules can help us understand the probability that certain items will appear together, or the probability that one item will appear when another item appears.
You can use the association_rules function in the mlxtend library to extract association rules in Python. The following is a simple sample code:
from mlxtend.frequent_patterns import association_rules, apriori data = [['牛奶', '面包', '啤酒'], ['奶酪', '面包', '黄油'], ['牛奶', '面包', '黄油', '鸡蛋'], ['奶酪', '黄油', '鸡蛋'], ['面包', '啤酒']] # 使用apriori算法挖掘频繁项集 frequent_itemsets = apriori(data, min_support=0.6) # 使用association_rules函数提取关联规则 rules = association_rules(frequent_itemsets, metric="confidence", min_threshold=0.8) # 输出关联规则 print(rules)
In the above code, we first use the Apriori algorithm to find frequent itemsets in the data set. Then use the association_rules function to extract association rules. The first parameter of the function is the frequent itemset, the second parameter is the indicator for evaluating the association rules, here select confidence (confidence), and the third parameter is the minimum confidence threshold, here set to 0.8.
In the output, we can see that the algorithm found an association rule with a confidence level of 1.0: 'Bread' => 'Beer'. This means that 100% of the people who bought bread also bought beer. This association rule can be used to recommend products to users in recommendation systems.
- FP-Growth algorithm
FP-Growth algorithm is another classic algorithm in the field of association rule mining. It is faster than the Apriori algorithm and can handle large-scale of data sets.
The pyfpgrowth library can be used in Python to implement the FP-Growth algorithm. The following is a simple sample code:
import pyfpgrowth # 构建数据集 data = [['牛奶', '面包', '啤酒'], ['奶酪', '面包', '黄油'], ['牛奶', '面包', '黄油', '鸡蛋'], ['奶酪', '黄油', '鸡蛋'], ['面包', '啤酒']] # 使用FP-Growth算法挖掘频繁项集 patterns = pyfpgrowth.find_frequent_patterns(data, 2) # 使用FP-Growth算法提取关联规则 rules = pyfpgrowth.generate_association_rules(patterns, 0.8) # 输出频繁项集和关联规则 print(patterns) print(rules)
In the above code, we first define a data set, and then use the find_frequent_patterns function in the pyfpgrowth library to mine frequent itemsets. The first parameter of the function is the data set, and the second parameter is the support threshold. Here, we set the support threshold to 2, which means that each item set must appear in at least two shopping baskets. The function will return a dictionary containing all frequent itemsets and their support counts.
Then use the generate_association_rules function in the pyfpgrowth library to extract association rules. The first parameter of the function is a dictionary of frequent itemsets, and the second parameter is the confidence threshold. Here, we set the confidence threshold to 0.8.
In the output result, we can see that the algorithm found two frequent item sets: ('bread',) and ('bread', 'milk'). At the same time, the algorithm extracted an association rule with a confidence level of 1.0: ('bread',) => ('beer',). This means that 100% of people who buy bread will buy beer. In addition to this, you can also see other association rules with a confidence level higher than 0.8.
Summary
Association rule mining is a very useful data mining technique that can be used to discover relationships between different items in a data set and the impact of these relationships on other things. Python provides a variety of methods to implement association rule mining, including the Apriori algorithm and FP-Growth algorithm. In the specific implementation, we also need to pay attention to the threshold settings of frequent itemsets and association rules, and how to apply them to actual problems.
The above is the detailed content of Association rule mining techniques in Python. For more information, please follow other related articles on the PHP Chinese website!

Hot AI Tools

Undresser.AI Undress
AI-powered app for creating realistic nude photos

AI Clothes Remover
Online AI tool for removing clothes from photos.

Undress AI Tool
Undress images for free

Clothoff.io
AI clothes remover

AI Hentai Generator
Generate AI Hentai for free.

Hot Article

Hot Tools

Notepad++7.3.1
Easy-to-use and free code editor

SublimeText3 Chinese version
Chinese version, very easy to use

Zend Studio 13.0.1
Powerful PHP integrated development environment

Dreamweaver CS6
Visual web development tools

SublimeText3 Mac version
God-level code editing software (SublimeText3)

Hot Topics


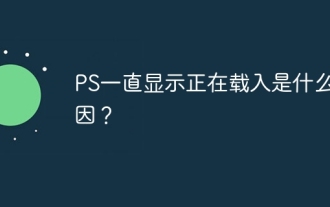
PS "Loading" problems are caused by resource access or processing problems: hard disk reading speed is slow or bad: Use CrystalDiskInfo to check the hard disk health and replace the problematic hard disk. Insufficient memory: Upgrade memory to meet PS's needs for high-resolution images and complex layer processing. Graphics card drivers are outdated or corrupted: Update the drivers to optimize communication between the PS and the graphics card. File paths are too long or file names have special characters: use short paths and avoid special characters. PS's own problem: Reinstall or repair the PS installer.
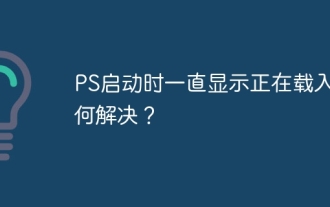
A PS stuck on "Loading" when booting can be caused by various reasons: Disable corrupt or conflicting plugins. Delete or rename a corrupted configuration file. Close unnecessary programs or upgrade memory to avoid insufficient memory. Upgrade to a solid-state drive to speed up hard drive reading. Reinstalling PS to repair corrupt system files or installation package issues. View error information during the startup process of error log analysis.
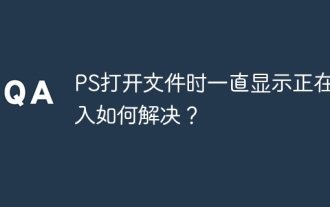
"Loading" stuttering occurs when opening a file on PS. The reasons may include: too large or corrupted file, insufficient memory, slow hard disk speed, graphics card driver problems, PS version or plug-in conflicts. The solutions are: check file size and integrity, increase memory, upgrade hard disk, update graphics card driver, uninstall or disable suspicious plug-ins, and reinstall PS. This problem can be effectively solved by gradually checking and making good use of PS performance settings and developing good file management habits.
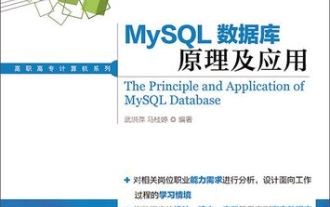
The article introduces the operation of MySQL database. First, you need to install a MySQL client, such as MySQLWorkbench or command line client. 1. Use the mysql-uroot-p command to connect to the server and log in with the root account password; 2. Use CREATEDATABASE to create a database, and USE select a database; 3. Use CREATETABLE to create a table, define fields and data types; 4. Use INSERTINTO to insert data, query data, update data by UPDATE, and delete data by DELETE. Only by mastering these steps, learning to deal with common problems and optimizing database performance can you use MySQL efficiently.
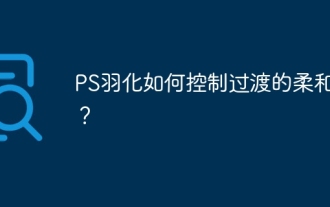
The key to feather control is to understand its gradual nature. PS itself does not provide the option to directly control the gradient curve, but you can flexibly adjust the radius and gradient softness by multiple feathering, matching masks, and fine selections to achieve a natural transition effect.
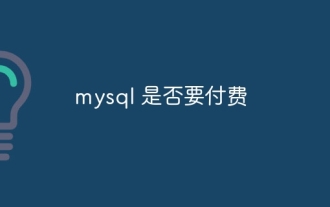
MySQL has a free community version and a paid enterprise version. The community version can be used and modified for free, but the support is limited and is suitable for applications with low stability requirements and strong technical capabilities. The Enterprise Edition provides comprehensive commercial support for applications that require a stable, reliable, high-performance database and willing to pay for support. Factors considered when choosing a version include application criticality, budgeting, and technical skills. There is no perfect option, only the most suitable option, and you need to choose carefully according to the specific situation.
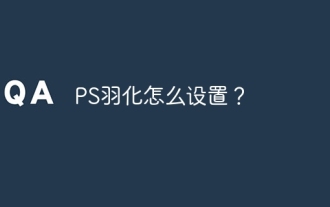
PS feathering is an image edge blur effect, which is achieved by weighted average of pixels in the edge area. Setting the feather radius can control the degree of blur, and the larger the value, the more blurred it is. Flexible adjustment of the radius can optimize the effect according to images and needs. For example, using a smaller radius to maintain details when processing character photos, and using a larger radius to create a hazy feeling when processing art works. However, it should be noted that too large the radius can easily lose edge details, and too small the effect will not be obvious. The feathering effect is affected by the image resolution and needs to be adjusted according to image understanding and effect grasp.
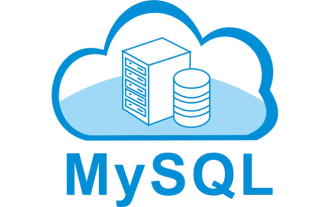
MySQL performance optimization needs to start from three aspects: installation configuration, indexing and query optimization, monitoring and tuning. 1. After installation, you need to adjust the my.cnf file according to the server configuration, such as the innodb_buffer_pool_size parameter, and close query_cache_size; 2. Create a suitable index to avoid excessive indexes, and optimize query statements, such as using the EXPLAIN command to analyze the execution plan; 3. Use MySQL's own monitoring tool (SHOWPROCESSLIST, SHOWSTATUS) to monitor the database health, and regularly back up and organize the database. Only by continuously optimizing these steps can the performance of MySQL database be improved.
