


Beat LLaMA? The ranking of the most powerful 'Falcon' in history is in doubt, Fu Yao personally tested 7 lines of code, and LeCun forwarded it to like
Some time ago, the fledgling Falcon crushed LLaMA in the LLM rankings, causing waves in the entire community.
But, is Falcon really better than LLaMA?
Short answer: Probably not.
Fu Yao’s team conducted a more in-depth evaluation of the model:
"We The evaluation of LLaMA 65B was reproduced on MMLU and obtained a score of 61.4, which is close to the official score (63.4), much higher than its score on the Open LLM Leaderboard (48.8), and significantly higher than the Falcon (52.7)."
No fancy prompt engineering, no fancy decoding, everything is the default setting.
Currently, the code and test methods have been made public on Github.
There are doubts about the Falcons surpassing LLaMA, LeCun expressed his position, the problem with the test script...
LLaMA is true· Strength
Currently in the OpenLLM rankings, Falcon ranks first, surpassing LLaMA, and has been highly recommended by researchers including Thomas Wolf.
However, some people have their doubts.
First, a netizen questioned where these LLaMA numbers came from. They seemed inconsistent with the numbers in the paper...
Subsequently, OpenAI scientist Andrej Karpathy also expressed concern about why LLaMA 65B’s score on the Open LLM rankings was significantly lower than the official one (48.8 vs. 63.4).
And post, so far I have avoided tweeting about Falcons because of this, not sure.
In order to clarify this problem, Fu Yao and team members decided to conduct a public test on LLaMA 65B, and the result was 61.4 points.
In the test, the researchers did not use any special mechanism, and LLaMA 65B was able to achieve this score.
This result just proves that if you want the model to achieve a level close to GPT-3.5, it is best to use RLHF on LLaMA 65B.
The basis is the findings of a Chain-of-Thought Hub paper recently published by Fu Yao’s team.
Of course, Fu Yao said that their evaluation was not intended to cause a dispute between LLaMA and Falcon. After all, these are great open source projects. Models have made significant contributions to this field!
In addition, Falcon has a more convenient license, which also gives it great development potential.
For this latest review, netizen BlancheMinerva pointed out that a fair comparison should be to run Falcon on MMLU under default settings.
In this regard, Fu Yao said that this was correct and that the work was being carried out and the results were expected to be available in one day.
No matter what the final result is, you must know that the mountain of GPT-4 is the goal that the open source community really wants to pursue.
OpenLLM ranking problem
Researchers from Meta praised Fu Yao for reproducing the LLaMa results well and pointed out the problem with the OpenLLM ranking list.
At the same time, he also shared some questions about the OpenLLM rankings.
First, the MMLU results: The LLaMa 65B MMLU result is 15 points on the leaderboard, but it is the same for the 7B model. There is also a small performance gap between the 13B and 30B models.
OpenLLM really needs to look at this before announcing which model is the best.
Benchmarks: How are these benchmarks chosen?
The ARC 25 shot and the Hellaswag 10 shot don’t seem to be particularly relevant to LLM. It would be better if some generative benchmarks could be included. Although generative benchmarks have their limitations, they can still be useful.
Single Average Score: It is always tempting to reduce the results to a single score, and the average score is easiest.
But in this case, is the average of 4 benchmarks really useful? Is getting 1 point on MMLU the same as getting 1 point on HellaSwag?
In the world of rapid iteration of LLM, there is definitely some value in developing such a ranking list.
And Lucas Beyer, a researcher from Google, also expressed his opinion,
Crazy Yes, NLP researchers have different understandings of the same benchmark, thus leading to completely different results. At the same time, every time one of my colleagues implements a metric, I immediately ask them if they actually check for a perfect reproduction of the official code, and if not, discard their results.
Also, he said that as far as I know, regardless of the model, it will not actually reproduce the results of the original benchmark.
Netizens echoed that this is the reality of LLM benchmark...
Falcon——Open source, commercially available, strong performance
Speaking of Falcon, it is actually worth a good review.
According to LeCun, in the era of large models, open source is the most important.
After Meta’s LLaMA code was leaked, developers from all walks of life began to be eager to try it.
Falcon is a surprise weapon developed by the Technology Innovation Institute (TII) in Abu Dhabi, United Arab Emirates.
In terms of performance when it was first released, Falcon performed better than LLaMA.
Currently, "Falcon" has three versions-1B, 7B and 40B.
TII stated that Falcon is the most powerful open source language model to date. Its largest version, Falcon 40B, has 40 billion parameters, which is still a bit smaller in scale than LLaMA, which has 65 billion parameters.
However, TII has previously stated that despite its small scale, Falcon has great performance.
Faisal Al Bannai, Secretary General of the Advanced Technology Research Council (ATRC), believes that the release of “Falcon” will break the way to obtain LLM and allow researchers and entrepreneurs to propose the best solutions. Most innovative use cases.
The two versions of FalconLM, Falcon 40B Instruct and Falcon 40B, rank in the top two on the Hugging Face OpenLLM rankings, while Meta’s LLaMA Located in third place.
The problem with the rankings mentioned above is exactly this.
Although the "Falcon" paper has not yet been publicly released, Falcon 40B has been extensively trained on a carefully screened 1 trillion token network data set.
Researchers have revealed that "Falcon" attaches great importance to the importance of achieving high performance on large-scale data during the training process.
What we all know is that LLM is very sensitive to the quality of training data, which is why researchers spend a lot of effort building one that can perform efficient processing on tens of thousands of CPU cores data pipeline.
The purpose is to extract high-quality content from the Internet based on filtering and deduplication.
Currently, TII has released a refined network data set, which is a carefully filtered and deduplicated data set. Practice has proved that it is very effective.
The model trained using only this data set can be on par with other LLMs, or even surpass them in performance. This demonstrates the excellent quality and influence of "Falcon".
In addition, the Falcon model also has multi-language capabilities.
It understands English, German, Spanish and French, and some small European languages such as Dutch, Italian, Romanian, Portuguese, Czech, Polish and Swedish I also know a lot about it.
Falcon 40B is the second truly open source model after the release of the H2O.ai model.
In addition, there is another very important point - Falcon is currently the only open source model that can be used commercially for free.
In the early days, TII required that if Falcon is used for commercial purposes and generates more than $1 million in attributable income, a 10% "use tax" will be charged.
But it didn’t take long for the wealthy Middle Eastern tycoons to lift this restriction.
At least so far, all commercial use and fine-tuning of Falcon will be free of charge.
The wealthy people said that they do not need to make money through this model for the time being.
Moreover, TII is also soliciting commercialization plans from around the world.
For potential scientific research and commercialization solutions, they will also provide more "training computing power support" or provide further commercialization opportunities.
This is simply saying: as long as the project is good, the model is free! Enough computing power! If you don’t have enough money, we can still collect it for you!
For start-ups, this is simply a "one-stop solution for AI large model entrepreneurship" from the Middle East tycoon.
According to the development team, an important aspect of FalconLM’s competitive advantage is the selection of training data.
The research team developed a process to extract high-quality data from public crawled datasets and remove duplicate data.
After thorough cleaning of redundant and duplicate content, 5 trillion tokens were retained—enough to train powerful language models.
The 40B Falcon LM uses 1 trillion tokens for training, and the 7B version of the model uses 1.5 trillion tokens for training.
(The research team’s goal is to filter out only the highest quality raw data from the Common Crawl using the RefinedWeb dataset)
In addition, Falcon’s training costs are relatively more controllable.
TII stated that compared with GPT-3, Falcon achieved significant performance improvements while using only 75% of the training computing budget.
And only requires 20% of the calculation time during inference, which was successfully implemented Efficient utilization of computing resources.
The above is the detailed content of Beat LLaMA? The ranking of the most powerful 'Falcon' in history is in doubt, Fu Yao personally tested 7 lines of code, and LeCun forwarded it to like. For more information, please follow other related articles on the PHP Chinese website!

Hot AI Tools

Undresser.AI Undress
AI-powered app for creating realistic nude photos

AI Clothes Remover
Online AI tool for removing clothes from photos.

Undress AI Tool
Undress images for free

Clothoff.io
AI clothes remover

AI Hentai Generator
Generate AI Hentai for free.

Hot Article

Hot Tools

Notepad++7.3.1
Easy-to-use and free code editor

SublimeText3 Chinese version
Chinese version, very easy to use

Zend Studio 13.0.1
Powerful PHP integrated development environment

Dreamweaver CS6
Visual web development tools

SublimeText3 Mac version
God-level code editing software (SublimeText3)

Hot Topics


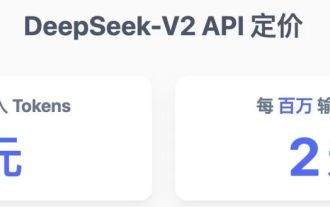
Imagine an artificial intelligence model that not only has the ability to surpass traditional computing, but also achieves more efficient performance at a lower cost. This is not science fiction, DeepSeek-V2[1], the world’s most powerful open source MoE model is here. DeepSeek-V2 is a powerful mixture of experts (MoE) language model with the characteristics of economical training and efficient inference. It consists of 236B parameters, 21B of which are used to activate each marker. Compared with DeepSeek67B, DeepSeek-V2 has stronger performance, while saving 42.5% of training costs, reducing KV cache by 93.3%, and increasing the maximum generation throughput to 5.76 times. DeepSeek is a company exploring general artificial intelligence
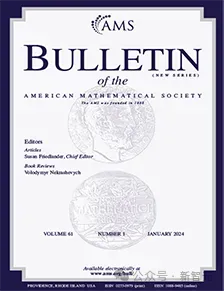
AI is indeed changing mathematics. Recently, Tao Zhexuan, who has been paying close attention to this issue, forwarded the latest issue of "Bulletin of the American Mathematical Society" (Bulletin of the American Mathematical Society). Focusing on the topic "Will machines change mathematics?", many mathematicians expressed their opinions. The whole process was full of sparks, hardcore and exciting. The author has a strong lineup, including Fields Medal winner Akshay Venkatesh, Chinese mathematician Zheng Lejun, NYU computer scientist Ernest Davis and many other well-known scholars in the industry. The world of AI has changed dramatically. You know, many of these articles were submitted a year ago.
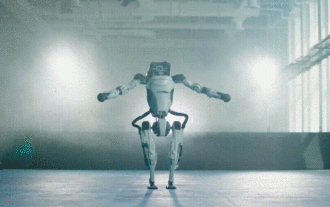
Boston Dynamics Atlas officially enters the era of electric robots! Yesterday, the hydraulic Atlas just "tearfully" withdrew from the stage of history. Today, Boston Dynamics announced that the electric Atlas is on the job. It seems that in the field of commercial humanoid robots, Boston Dynamics is determined to compete with Tesla. After the new video was released, it had already been viewed by more than one million people in just ten hours. The old people leave and new roles appear. This is a historical necessity. There is no doubt that this year is the explosive year of humanoid robots. Netizens commented: The advancement of robots has made this year's opening ceremony look like a human, and the degree of freedom is far greater than that of humans. But is this really not a horror movie? At the beginning of the video, Atlas is lying calmly on the ground, seemingly on his back. What follows is jaw-dropping
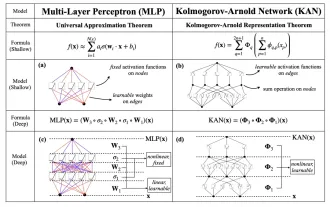
Earlier this month, researchers from MIT and other institutions proposed a very promising alternative to MLP - KAN. KAN outperforms MLP in terms of accuracy and interpretability. And it can outperform MLP running with a larger number of parameters with a very small number of parameters. For example, the authors stated that they used KAN to reproduce DeepMind's results with a smaller network and a higher degree of automation. Specifically, DeepMind's MLP has about 300,000 parameters, while KAN only has about 200 parameters. KAN has a strong mathematical foundation like MLP. MLP is based on the universal approximation theorem, while KAN is based on the Kolmogorov-Arnold representation theorem. As shown in the figure below, KAN has
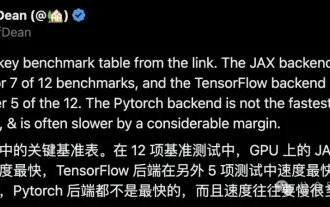
The performance of JAX, promoted by Google, has surpassed that of Pytorch and TensorFlow in recent benchmark tests, ranking first in 7 indicators. And the test was not done on the TPU with the best JAX performance. Although among developers, Pytorch is still more popular than Tensorflow. But in the future, perhaps more large models will be trained and run based on the JAX platform. Models Recently, the Keras team benchmarked three backends (TensorFlow, JAX, PyTorch) with the native PyTorch implementation and Keras2 with TensorFlow. First, they select a set of mainstream
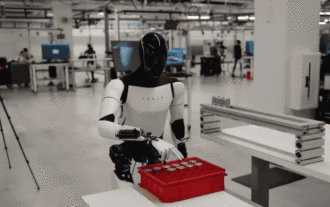
The latest video of Tesla's robot Optimus is released, and it can already work in the factory. At normal speed, it sorts batteries (Tesla's 4680 batteries) like this: The official also released what it looks like at 20x speed - on a small "workstation", picking and picking and picking: This time it is released One of the highlights of the video is that Optimus completes this work in the factory, completely autonomously, without human intervention throughout the process. And from the perspective of Optimus, it can also pick up and place the crooked battery, focusing on automatic error correction: Regarding Optimus's hand, NVIDIA scientist Jim Fan gave a high evaluation: Optimus's hand is the world's five-fingered robot. One of the most dexterous. Its hands are not only tactile
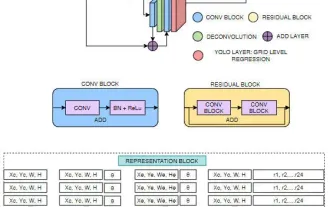
Target detection is a relatively mature problem in autonomous driving systems, among which pedestrian detection is one of the earliest algorithms to be deployed. Very comprehensive research has been carried out in most papers. However, distance perception using fisheye cameras for surround view is relatively less studied. Due to large radial distortion, standard bounding box representation is difficult to implement in fisheye cameras. To alleviate the above description, we explore extended bounding box, ellipse, and general polygon designs into polar/angular representations and define an instance segmentation mIOU metric to analyze these representations. The proposed model fisheyeDetNet with polygonal shape outperforms other models and simultaneously achieves 49.5% mAP on the Valeo fisheye camera dataset for autonomous driving
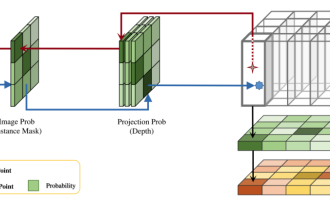
This paper explores the problem of accurately detecting objects from different viewing angles (such as perspective and bird's-eye view) in autonomous driving, especially how to effectively transform features from perspective (PV) to bird's-eye view (BEV) space. Transformation is implemented via the Visual Transformation (VT) module. Existing methods are broadly divided into two strategies: 2D to 3D and 3D to 2D conversion. 2D-to-3D methods improve dense 2D features by predicting depth probabilities, but the inherent uncertainty of depth predictions, especially in distant regions, may introduce inaccuracies. While 3D to 2D methods usually use 3D queries to sample 2D features and learn the attention weights of the correspondence between 3D and 2D features through a Transformer, which increases the computational and deployment time.
