Quickly get started with ElasticSearch dsl with ChatGPT
As a SQL player, after coming into contact with ElasticSearch, I was troubled by the strange way of writing its dsl, such as bool should filter in what scenarios should it be nested, and when should bool be nested? Query is necessary and when can it be done. When troubleshooting problems, it is inevitable to write dsl by hand, and often receive ES errors.
In order to solve this problem, I tried to let ChatGPT translate for me, and the effect was pretty good. Let’s look at it according to the scenario.
Scenario 1: List fuzzy query
In the list page of the B-side backend system, we can usually see many filter boxes. Product managers often put the function of fuzzy matching by ID and Name into a filter box called "better user experience". If written in SQL, it is very simple:
select * from user where client_id = 123 anduser_id like '%张三%' or user_name like '%张三%';
If stored in MySQL, adding the client_id index can greatly reduce the amount of filtered data, and the performance will not be too bad.
If the amount of data is very large, then use ES. We can ask ChatGPT like this:
Start the question:
ES index name "user", has three fields
- user_id string
- user_name string
- client_id int
I want to query records with the following conditions: client_id = 123 and (user_name = 'Zhang San' or user_id = 'Zhang San'). How should I write dsl?
Start of reply:
To query the records in the "user" index that meet the following conditions: client_id = 123 and (user_name = 'Zhang San ' or user_id = 'Zhang San'), you can use bool query combined with term query to achieve this. Here is an example of the corresponding DSL query:
GET /user/_search{"query": {"bool": {"filter": [{"term": {"client_id": 123}},{"bool": {"should": [{"term": {"user_name": "张三"}},{"term": {"user_id": "张三"}}]}}]}}}
In this query, we use a bool query to ensure that the specified criteria are used to filter the documents.
In the filter clause, we have two conditions: one is for the condition that the value of the "client_id" field is 123, using the term query. The second condition is a nested bool query that contains a should clause. The should clause contains two term queries: one for the "user_name" field and the other for the "user_id" field, both of which check whether the value is "Zhang San". By using the should clause, we indicate that any one of the conditions must match.
This DSL query will return document records whose client_id is 123 and the user name is "Zhang San" or the user ID is "Zhang San".
End of reply:
ChatGPT not only gave the answer, but also gave an explanation. Let’s make some modifications to the index in the business. good.
Scenario 2: Filtering based on aggregation results having
Based on the above user table, we add a ticket_id field to represent the processed work orders, we want to check Process users with more than 100 work orders. SQL can be written like this:
select user_id, max(user_name) as user_name,count(ticket_id) as ticket_cnt from user where client_id = 123having count(ticket_id) > 100;
Ask a question to ChatGPT:
Start asking:
ES index name "user", including the following fields:
- ##user_id string
- user_name string
- client_id int
- ticket_id
Start of reply:
To aggregate according to the "user_id" field, and query the "user_id" that meets the condition count(ticket_id) > 100, and the corresponding " user_name", which can be implemented using terms aggregation and bucket_selector pipeline aggregation in aggregate queries. The following is an example of the corresponding DSL query: 在这个查询中,我们使用了 terms 聚合对 "user_id" 字段进行分组,并在聚合结果中嵌套了 user_name 字段的 terms 聚合和 ticket_id 字段的 value_count 聚合。 在 terms 聚合中,我们设置了 min_doc_count 参数为 101,以仅返回满足 count(ticket_id) > 100 的聚合桶。这样可以过滤掉不满足条件的聚合结果。 最终,我们使用 bucket_selector 管道聚合来进行桶过滤,仅保留满足条件 params.count > 100 的聚合桶。这样,最终的聚合结果中将只包含满足条件的 "user_id" 和对应的 "user_name"。 请注意,根据您的具体需求,您可能需要根据实际情况调整聚合查询中的参数和设置,如设置合适的 size 值来控制返回的聚合桶数量等。 回复结束。 向ChatGPT提问: 提问开始: ES index 名字 "user",包含以下字段: 我要查询处理ticket数量top10 的 user_id 以及对应的user_name 回复开始: 在这个查询中,我们使用了 terms 聚合对 "user_id" 字段进行分组,并按照 "ticket_count" 字段的降序对分组结果进行排序。 在 terms 聚合中,我们设置了 size 参数为 10,以仅返回前 10 个处理 ticket 数量最多的 "user_id"。 在每个聚合桶中,我们还嵌套了 user_name 字段的 terms 聚合和 ticket_id 字段的 value_count 聚合,分别用于获取对应的 "user_name" 和计算 ticket 数量。 此外,我们使用了 top_hits 子聚合来获取每个聚合桶中的匹配文档的详细信息。在这里,我们设置了 size 参数为 1,以仅获取每个聚合桶中的一条文档信息。 最终,聚合查询的结果将包含处理 ticket 数量排名前 10 的 "user_id",对应的 "user_name",以及每个用户最新的一条文档信息。 请注意,根据实际情况,您可能需要根据具体需求调整聚合查询中的参数和设置,如设置合适的 size 值来控制返回的聚合桶数量,以及添加其他的聚合或过滤条件等。 回复结束。 本着快速出活/快速解决问题的心态,ChatGPT确实提升了工作效率。不过在解决需求之后,还是要补补课,了解下ElasticSearch的用法和工作机制。因为ChatGPT是语言模型,一旦逻辑复杂起来,ChatGPT就开始瞎说了。GET /user/_search{"size": 0,"aggs": {"users": {"terms": {"field": "user_id","size": 10,"min_doc_count": 101// 设置最小文档数,只返回 count(ticket_id) > 100 的聚合结果},"aggs": {"user_name": {"terms": {"field": "user_name","size": 1}},"ticket_count": {"value_count": {"field": "ticket_id"}},"bucket_filter": {"bucket_selector": {"buckets_path": {"count": "ticket_count"},"script": "params.count > 100"// 仅保留 count(ticket_id) > 100 的聚合桶}}}}}}
场景三: 聚合以后取topK
要查询处理 ticket 数量排名前 10 的 "user_id",以及对应的 "user_name",可以使用 terms 聚合和 top_hits 子聚合来实现。以下是相应的 DSL 查询示例:GET /user/_search{"size": 0,"aggs": {"top_users": {"terms": {"field": "user_id","size": 10,"order": {"ticket_count": "desc"}},"aggs": {"user_name": {"terms": {"field": "user_name","size": 1}},"ticket_count": {"value_count": {"field": "ticket_id"}},"top_user_hits": {"top_hits": {"size": 1}}}}}}
The above is the detailed content of Quickly get started with ElasticSearch dsl with ChatGPT. For more information, please follow other related articles on the PHP Chinese website!

Hot AI Tools

Undresser.AI Undress
AI-powered app for creating realistic nude photos

AI Clothes Remover
Online AI tool for removing clothes from photos.

Undress AI Tool
Undress images for free

Clothoff.io
AI clothes remover

AI Hentai Generator
Generate AI Hentai for free.

Hot Article

Hot Tools

Notepad++7.3.1
Easy-to-use and free code editor

SublimeText3 Chinese version
Chinese version, very easy to use

Zend Studio 13.0.1
Powerful PHP integrated development environment

Dreamweaver CS6
Visual web development tools

SublimeText3 Mac version
God-level code editing software (SublimeText3)

Hot Topics


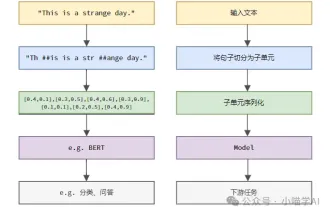
Language models reason about text, which is usually in the form of strings, but the input to the model can only be numbers, so the text needs to be converted into numerical form. Tokenization is a basic task of natural language processing. It can divide a continuous text sequence (such as sentences, paragraphs, etc.) into a character sequence (such as words, phrases, characters, punctuation, etc.) according to specific needs. The units in it Called a token or word. According to the specific process shown in the figure below, the text sentences are first divided into units, then the single elements are digitized (mapped into vectors), then these vectors are input to the model for encoding, and finally output to downstream tasks to further obtain the final result. Text segmentation can be divided into Toke according to the granularity of text segmentation.
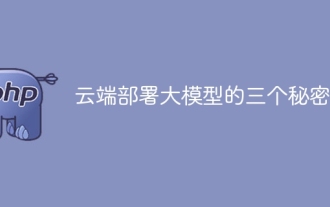
Compilation|Produced by Xingxuan|51CTO Technology Stack (WeChat ID: blog51cto) In the past two years, I have been more involved in generative AI projects using large language models (LLMs) rather than traditional systems. I'm starting to miss serverless cloud computing. Their applications range from enhancing conversational AI to providing complex analytics solutions for various industries, and many other capabilities. Many enterprises deploy these models on cloud platforms because public cloud providers already provide a ready-made ecosystem and it is the path of least resistance. However, it doesn't come cheap. The cloud also offers other benefits such as scalability, efficiency and advanced computing capabilities (GPUs available on demand). There are some little-known aspects of deploying LLM on public cloud platforms
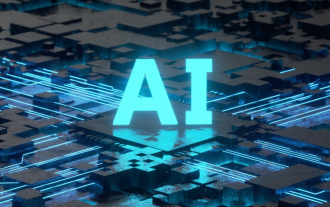
Editor |ScienceAI Question Answering (QA) data set plays a vital role in promoting natural language processing (NLP) research. High-quality QA data sets can not only be used to fine-tune models, but also effectively evaluate the capabilities of large language models (LLM), especially the ability to understand and reason about scientific knowledge. Although there are currently many scientific QA data sets covering medicine, chemistry, biology and other fields, these data sets still have some shortcomings. First, the data form is relatively simple, most of which are multiple-choice questions. They are easy to evaluate, but limit the model's answer selection range and cannot fully test the model's ability to answer scientific questions. In contrast, open-ended Q&A
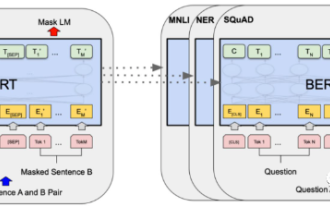
In 2018, Google released BERT. Once it was released, it defeated the State-of-the-art (Sota) results of 11 NLP tasks in one fell swoop, becoming a new milestone in the NLP world. The structure of BERT is shown in the figure below. On the left is the BERT model preset. The training process, on the right is the fine-tuning process for specific tasks. Among them, the fine-tuning stage is for fine-tuning when it is subsequently used in some downstream tasks, such as text classification, part-of-speech tagging, question and answer systems, etc. BERT can be fine-tuned on different tasks without adjusting the structure. Through the task design of "pre-trained language model + downstream task fine-tuning", it brings powerful model effects. Since then, "pre-training language model + downstream task fine-tuning" has become the mainstream training in the NLP field.
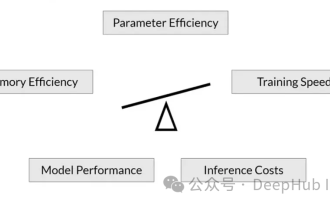
As language models scale to unprecedented scale, comprehensive fine-tuning for downstream tasks becomes prohibitively expensive. In order to solve this problem, researchers began to pay attention to and adopt the PEFT method. The main idea of the PEFT method is to limit the scope of fine-tuning to a small set of parameters to reduce computational costs while still achieving state-of-the-art performance on natural language understanding tasks. In this way, researchers can save computing resources while maintaining high performance, bringing new research hotspots to the field of natural language processing. RoSA is a new PEFT technique that, through experiments on a set of benchmarks, is found to outperform previous low-rank adaptive (LoRA) and pure sparse fine-tuning methods using the same parameter budget. This article will go into depth
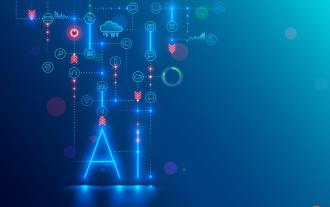
According to news on February 25, Meta announced on Friday local time that it will launch a new large-scale language model based on artificial intelligence (AI) for the research community, joining Microsoft, Google and other companies stimulated by ChatGPT to join artificial intelligence. Intelligent competition. Meta's LLaMA is the abbreviation of "Large Language Model MetaAI" (LargeLanguageModelMetaAI), which is available under a non-commercial license to researchers and entities in government, community, and academia. The company will make the underlying code available to users, so they can tweak the model themselves and use it for research-related use cases. Meta stated that the model’s requirements for computing power
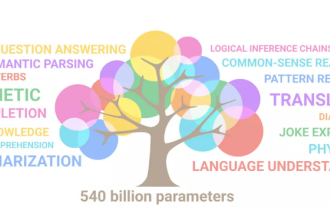
The progress of natural language processing in recent years has largely come from large-scale language models. Each new model released pushes the amount of parameters and training data to new highs, and at the same time, the existing benchmark rankings will be slaughtered. ! For example, in April this year, Google released the 540 billion-parameter language model PaLM (Pathways Language Model), which successfully surpassed humans in a series of language and reasoning tests, especially its excellent performance in few-shot small sample learning scenarios. PaLM is considered to be the development direction of the next generation language model. In the same way, visual language models actually work wonders, and performance can be improved by increasing the scale of the model. Of course, if it is just a multi-tasking visual language model
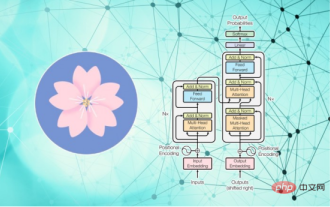
Translator | Reviewed by Li Rui | Sun Shujuan BigScience research project recently released a large language model BLOOM. At first glance, it looks like another attempt to copy OpenAI's GPT-3. But what sets BLOOM apart from other large-scale natural language models (LLMs) is its efforts to research, develop, train, and release machine learning models. In recent years, large technology companies have hidden large-scale natural language models (LLMs) like strict trade secrets, and the BigScience team has put transparency and openness at the center of BLOOM from the beginning of the project. The result is a large-scale language model that can be studied and studied, and made available to everyone. B
