


Tencent's robot dog evolves: mastering autonomous decision-making capabilities through deep learning
On June 14, Tencent Robotics Decision-making ability has been greatly improved.
Letting robot dogs move as flexibly and stably as humans and animals is a long-term goal in the field of robotics research. The continuous advancement of deep learning technology allows machines to master relevant abilities through "learning" and learn to deal with complex changes. environment becomes feasible.
Introducing pre-training and reinforcement learning: making the robot dog more agile
By introducing pre-training models and reinforcement learning technology, Tencent Robotics Instead of re-learning, you can reuse the multi-level knowledge of posture, environmental perception, and strategic planning that you have learned, and make inferences about other cases from one instance to flexibly respond to complex environments
This series of learning is divided into three stages:
In the first stage, through the motion capture system commonly used in game technology, the researcher collected the movement posture data of real dogs, including walking, running, jumping, standing and other actions, and used these data to construct an imitation learning task in the simulator. , and then abstract and compress the information in these data into a deep neural network model. These models can not only accurately cover the collected animal movement posture information, but also have high interpretability.
Tencent Robotics These technologies and data play a certain auxiliary role in physical simulation-based agent training and real-world robot strategy deployment.
The neural network model only accepts the proprioceptive information of the robot dog (such as motor status) as input and is trained in an imitation learning manner. In the next step, the model incorporates sensory data from the surrounding environment, such as using other sensors to detect obstacles underfoot.
In the second stage, additional network parameters are used to connect the smart posture of the robot dog mastered in the first stage with external perception, so that the robot dog can respond to the external environment through the smart posture that has been learned. When the robot dog adapts to a variety of complex environments, the knowledge that links smart postures with external perception will also be solidified and stored in the neural network structure.
In the third stage, using the neural network obtained in the above two pre-training stages, the robot dog has the prerequisite and opportunity to focus on solving the top-level policy learning problem, and finally has the ability to solve complex tasks end-to-end. In the third phase, additional networks will be added to collect data related to complex tasks, such as obtaining information about opponents and flags in the game. In addition, by comprehensively analyzing all information, the neural network responsible for strategy learning will learn high-level strategies for the task, such as which direction to run, predicting the opponent's behavior to decide whether to continue chasing, etc.
The knowledge learned at each stage above can be expanded and adjusted without re-learning, so it can be continuously accumulated and learned continuously.
Robot Dog Obstacle Chase Competition: Has autonomous decision-making and control capabilities
In order to test these new skills mastered by Max, the researchers were inspired by the obstacle chasing game "World Chase Tag" and designed a two-dog obstacle chasing game. World Chase Tag is a competitive obstacle chasing organization founded in the United Kingdom in 2014. It is standardized from folk children's chasing games. Generally speaking, each round of the obstacle chasing competition involves two athletes competing against each other. One is the pursuer (called the attacker) and the other is the dodger (called the defender). When an athlete competes throughout the entire The team will receive one point when they successfully evade their opponent (i.e. no contact occurs) during the pursuit round (i.e. 20 seconds). The team that scores the most points in the predetermined number of chasing rounds wins the game.
The venue size of the robot dog obstacle chasing competition is 4.5 meters x 4.5 meters, with some obstacles scattered on it. At the beginning of the game, two MAX robot dogs will be placed at random locations in the field, and one robot dog will be randomly assigned the role of a pursuer and the other a dodger. At the same time, a flag will be placed at a random location in the field.
The goal of the dodger is to get as close to the flag as possible without getting caught by the pursuer. The pursuer's task is to catch the evader. If the dodger successfully touches the flag before being caught, the roles of the two robot dogs will instantly switch, and the flag will reappear in another random location. The game ends when the dodger is caught by the current pursuer and the robot dog playing the role of the pursuer wins. In all games, the average forward speed of the two robot dogs is limited to 0.5m/s.
Judging from this game, based on the pre-trained model, the robot dog already has certain reasoning and decision-making capabilities through deep reinforcement learning:
For example, when the pursuer realizes that he can no longer catch up with the dodger before it touches the flag, the pursuer will give up the pursuit and instead wander away from the dodger in order to wait for the next important step. The set flag appears.
In addition, when the pursuer is about to catch the dodger at the last moment, it likes to jump up and make a "pounce" action towards the dodger, which is very similar to the behavior of animals when catching prey, or when the dodger is about to touch the flag. will exhibit the same behavior at times. These are all proactive acceleration measures taken by the robot dog to ensure its victory.
According to reports, all control strategies of the robot dog in the game are neural network strategies. They are learned in simulation and through zero-shot transfer (zero adjustment transfer), allowing the neural network to simulate human reasoning methods to identify never See new things and deploy this knowledge to real robot dogs. For example, as shown in the figure below, the knowledge of avoiding obstacles that the robot dog learned in the pre-training model is used in the game, even though the scenes with obstacles are not trained in the virtual world of Chase Tag Game (only in the virtual world After training in game scenes on flat ground), the robot dog can also successfully complete the task.
Tencent Robotics Learning technology is introduced into the field of robots to improve the control capabilities of robots and make them more flexible. This also lays a solid foundation for robots to enter real life and serve human beings.
The above is the detailed content of Tencent's robot dog evolves: mastering autonomous decision-making capabilities through deep learning. For more information, please follow other related articles on the PHP Chinese website!

Hot AI Tools

Undresser.AI Undress
AI-powered app for creating realistic nude photos

AI Clothes Remover
Online AI tool for removing clothes from photos.

Undress AI Tool
Undress images for free

Clothoff.io
AI clothes remover

AI Hentai Generator
Generate AI Hentai for free.

Hot Article

Hot Tools

Notepad++7.3.1
Easy-to-use and free code editor

SublimeText3 Chinese version
Chinese version, very easy to use

Zend Studio 13.0.1
Powerful PHP integrated development environment

Dreamweaver CS6
Visual web development tools

SublimeText3 Mac version
God-level code editing software (SublimeText3)

Hot Topics



On May 30, Tencent announced a comprehensive upgrade of its Hunyuan model. The App "Tencent Yuanbao" based on the Hunyuan model was officially launched and can be downloaded from Apple and Android app stores. Compared with the Hunyuan applet version in the previous testing stage, Tencent Yuanbao provides core capabilities such as AI search, AI summary, and AI writing for work efficiency scenarios; for daily life scenarios, Yuanbao's gameplay is also richer and provides multiple features. AI application, and new gameplay methods such as creating personal agents are added. "Tencent does not strive to be the first to make large models." Liu Yuhong, vice president of Tencent Cloud and head of Tencent Hunyuan large model, said: "In the past year, we continued to promote the capabilities of Tencent Hunyuan large model. In the rich and massive Polish technology in business scenarios while gaining insights into users’ real needs
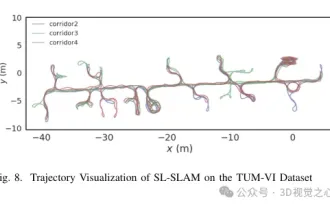
Written previously, today we discuss how deep learning technology can improve the performance of vision-based SLAM (simultaneous localization and mapping) in complex environments. By combining deep feature extraction and depth matching methods, here we introduce a versatile hybrid visual SLAM system designed to improve adaptation in challenging scenarios such as low-light conditions, dynamic lighting, weakly textured areas, and severe jitter. sex. Our system supports multiple modes, including extended monocular, stereo, monocular-inertial, and stereo-inertial configurations. In addition, it also analyzes how to combine visual SLAM with deep learning methods to inspire other research. Through extensive experiments on public datasets and self-sampled data, we demonstrate the superiority of SL-SLAM in terms of positioning accuracy and tracking robustness.
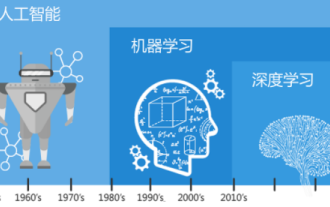
In today's wave of rapid technological changes, Artificial Intelligence (AI), Machine Learning (ML) and Deep Learning (DL) are like bright stars, leading the new wave of information technology. These three words frequently appear in various cutting-edge discussions and practical applications, but for many explorers who are new to this field, their specific meanings and their internal connections may still be shrouded in mystery. So let's take a look at this picture first. It can be seen that there is a close correlation and progressive relationship between deep learning, machine learning and artificial intelligence. Deep learning is a specific field of machine learning, and machine learning
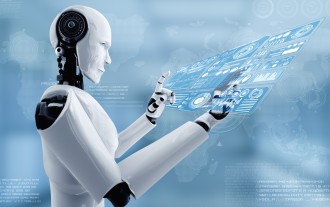
Almost 20 years have passed since the concept of deep learning was proposed in 2006. Deep learning, as a revolution in the field of artificial intelligence, has spawned many influential algorithms. So, what do you think are the top 10 algorithms for deep learning? The following are the top algorithms for deep learning in my opinion. They all occupy an important position in terms of innovation, application value and influence. 1. Deep neural network (DNN) background: Deep neural network (DNN), also called multi-layer perceptron, is the most common deep learning algorithm. When it was first invented, it was questioned due to the computing power bottleneck. Until recent years, computing power, The breakthrough came with the explosion of data. DNN is a neural network model that contains multiple hidden layers. In this model, each layer passes input to the next layer and
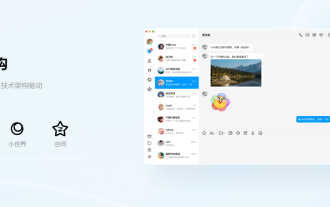
It is understood that Tencent QQ desktop client has undergone a series of drastic reforms. In response to user issues such as high memory usage, oversized installation packages, and slow startup, the QQ technical team has made special optimizations on memory and has made phased progress. Recently, the QQ technical team published an introductory article on the InfoQ platform, sharing its phased progress in special optimization of memory. According to reports, the memory challenges of the new version of QQ are mainly reflected in the following four aspects: Product form: It consists of a complex large panel (100+ modules of varying complexity) and a series of independent functional windows. There is a one-to-one correspondence between windows and rendering processes. The number of window processes greatly affects Electron’s memory usage. For that complex large panel, once there is no
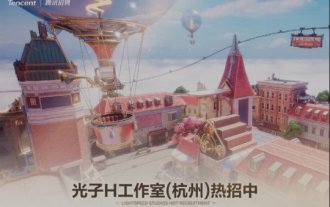
Recently, Tencent Interactive Entertainment Recruitment released a recruitment information, indicating that Photon H Studio is committed to developing a content-rich, AAA-level open world RPG project. The hot recruitment positions cover multiple fields such as UE5 engineers, backend, level design, action scene design, character modeling, special effects and distribution. The target working location of these positions is in Hangzhou, where NetEase is headquartered.

Editor | Radish Skin Since the release of the powerful AlphaFold2 in 2021, scientists have been using protein structure prediction models to map various protein structures within cells, discover drugs, and draw a "cosmic map" of every known protein interaction. . Just now, Google DeepMind released the AlphaFold3 model, which can perform joint structure predictions for complexes including proteins, nucleic acids, small molecules, ions and modified residues. The accuracy of AlphaFold3 has been significantly improved compared to many dedicated tools in the past (protein-ligand interaction, protein-nucleic acid interaction, antibody-antigen prediction). This shows that within a single unified deep learning framework, it is possible to achieve
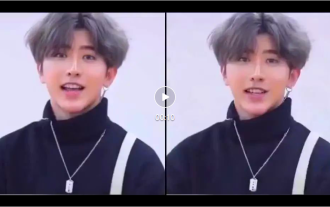
AniPortrait models are open source and can be played with freely. "A new productivity tool for Xiaopozhan Ghost Zone." Recently, a new project released by Tencent Open Source received such evaluation on Twitter. This project is AniPortrait, which generates high-quality animated portraits based on audio and a reference image. Without further ado, let’s take a look at the demo that may be warned by a lawyer’s letter: Anime images can also speak easily: The project has already received widespread praise after just a few days since it was launched: the number of GitHub Stars has exceeded 2,800. Let’s take a look at the innovations of AniPortrait. Paper title: AniPortrait:Audio-DrivenSynthesisof
