


Detailed explanation of Python-based web crawler technology
With the advent of the Internet and big data era, more and more data are dynamically generated and presented on web pages, which brings new challenges to data collection and processing. At this time, Web crawler technology came into being. Web crawler technology refers to technology that automatically obtains information on the Internet by writing programs. As a powerful programming language, Python has the advantages of being easy to learn, efficient and easy to use, and cross-platform. It has become an important choice in web crawler development.
This article will systematically introduce commonly used web crawler technologies in Python, including request modules, parsing modules, storage modules, etc.
1. Request module
The request module is the core of the web crawler. It can simulate the browser to send requests and obtain the required page content. Commonly used request modules include urllib, Requests and Selenium.
- urllib
urllib is an HTTP request module that comes with Python. It can obtain web page data from the network based on the URL. It supports URL encoding, modification of request headers, post, Cookies and other functions. Commonly used functions include urllib.request.urlopen(), urllib.request.urlretrieve(), urllib.request.build_opener(), etc.
You can get the source code of the website through the urllib.request.urlopen() function:
import urllib.request response = urllib.request.urlopen('http://www.example.com/') source_code = response.read().decode('utf-8') print(source_code)
- Requests
Requests is a Python third-party library. It is simpler and easier to use than urllib, and supports cookies, POST, proxy and other functions. Commonly used functions include requests.get(), requests.post(), requests.request(), etc.
You can get the response content through the requests.get() function:
import requests response = requests.get('http://www.example.com/') source_code = response.text print(source_code)
- Selenium
Selenium is an automated testing tool, used in web crawlers , it can simulate human operations by starting a browser, and can achieve functions such as obtaining page data dynamically generated by JS. Commonly used functions include selenium.webdriver.Chrome(), selenium.webdriver.Firefox(), selenium.webdriver.PhantomJS(), etc.
Get the web page source code through Selenium:
from selenium import webdriver browser = webdriver.Chrome() # 打开Chrome浏览器 browser.get('http://www.example.com/') source_code = browser.page_source # 获取网页源代码 print(source_code)
2. Parsing module
After getting the web page source code, the next step is to parse the file. Commonly used parsing modules in Python include regular expressions, BeautifulSoup and PyQuery.
- Regular expression
Regular expression is a magical and powerful tool that can match strings according to patterns and quickly extract the required data. You can use the re module in Python to call regular expressions.
For example, extract all links in the web page:
import re source_code = """ <!DOCTYPE html> <html> <head> <title>Example</title> </head> <body> <a href="http://www.example.com/">example</a> <a href="http://www.google.com/">google</a> </body> </html> """ pattern = re.compile('<a href="(.*?)">(.*?)</a>') # 匹配所有链接 results = re.findall(pattern, source_code) for result in results: print(result[0], result[1])
- BeautifulSoup
Beautiful Soup is a library in Python that can convert HTML files or XML files are parsed into tree structures to easily obtain data in HTML/XML files. It supports a variety of parsers, the commonly used ones are Python's built-in html.parser, lxml and html5lib.
For example, parse out all links in a web page:
from bs4 import BeautifulSoup source_code = """ <!DOCTYPE html> <html> <head> <title>Example</title> </head> <body> <a href="http://www.example.com/">example</a> <a href="http://www.google.com/">google</a> </body> </html> """ soup = BeautifulSoup(source_code, 'html.parser') links = soup.find_all('a') for link in links: print(link.get('href'), link.string)
- PyQuery
PyQuery is a jQuery-like Python library that converts HTML documents Into a structure similar to jQuery, elements in the web page can be directly obtained through CSS selectors. It depends on lxml library.
For example, parse out all the links in the web page:
from pyquery import PyQuery as pq source_code = """ <!DOCTYPE html> <html> <head> <title>Example</title> </head> <body> <a href="http://www.example.com/">example</a> <a href="http://www.google.com/">google</a> </body> </html> """ doc = pq(source_code) links = doc('a') for link in links: print(link.attrib['href'], link.text_content())
3. Storage module
After getting the required data, the next step is to store the data locally or in the database middle. Commonly used storage modules in Python include file modules, MySQLdb, pymongo, etc.
- File module
The file module can store data locally. Commonly used file modules include CSV, JSON, Excel, etc. Among them, the CSV module is one of the most commonly used file modules, which can write data into CSV files.
For example, write data to a CSV file:
import csv filename = 'example.csv' data = [['name', 'age', 'gender'], ['bob', 25, 'male'], ['alice', 22, 'female']] with open(filename, 'w', encoding='utf-8', newline='') as f: writer = csv.writer(f) for row in data: writer.writerow(row)
- MySQLdb
MySQLdb is a library for Python to connect to the MySQL database, which supports transactions , cursor and other functions.
For example, store data into a MySQL database:
import MySQLdb conn = MySQLdb.connect(host='localhost', port=3306, user='root', passwd='password', db='example', charset='utf8') cursor = conn.cursor() data = [('bob', 25, 'male'), ('alice', 22, 'female')] sql = "INSERT INTO users (name, age, gender) VALUES (%s, %s, %s)" try: cursor.executemany(sql, data) conn.commit() except: conn.rollback() cursor.close() conn.close()
- pymongo
pymongo is a library for Python to link to the MongoDB database. It supports a variety of Operations, such as adding, deleting, modifying, checking, etc.
For example, store data in the MongoDB database:
import pymongo client = pymongo.MongoClient('mongodb://localhost:27017/') db = client['example'] collection = db['users'] data = [{'name': 'bob', 'age': 25, 'gender': 'male'}, {'name': 'alice', 'age': 22, 'gender': 'female'}] collection.insert_many(data)
4. Summary
Web crawler technology in Python includes request module, parsing module and storage module, etc. Among them, the request module is the core of the web crawler, the parsing module is an important channel for obtaining data, and the storage module is the only way to persist data. Python has the advantages of being easy to learn, efficient and easy to use, and cross-platform in web crawler development, and has become an important choice in web crawler development.
The above is the detailed content of Detailed explanation of Python-based web crawler technology. For more information, please follow other related articles on the PHP Chinese website!

Hot AI Tools

Undresser.AI Undress
AI-powered app for creating realistic nude photos

AI Clothes Remover
Online AI tool for removing clothes from photos.

Undress AI Tool
Undress images for free

Clothoff.io
AI clothes remover

Video Face Swap
Swap faces in any video effortlessly with our completely free AI face swap tool!

Hot Article

Hot Tools

Notepad++7.3.1
Easy-to-use and free code editor

SublimeText3 Chinese version
Chinese version, very easy to use

Zend Studio 13.0.1
Powerful PHP integrated development environment

Dreamweaver CS6
Visual web development tools

SublimeText3 Mac version
God-level code editing software (SublimeText3)

Hot Topics


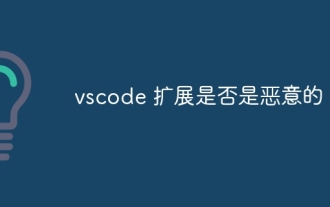
VS Code extensions pose malicious risks, such as hiding malicious code, exploiting vulnerabilities, and masturbating as legitimate extensions. Methods to identify malicious extensions include: checking publishers, reading comments, checking code, and installing with caution. Security measures also include: security awareness, good habits, regular updates and antivirus software.
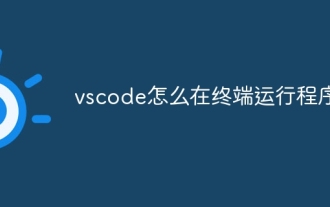
In VS Code, you can run the program in the terminal through the following steps: Prepare the code and open the integrated terminal to ensure that the code directory is consistent with the terminal working directory. Select the run command according to the programming language (such as Python's python your_file_name.py) to check whether it runs successfully and resolve errors. Use the debugger to improve debugging efficiency.
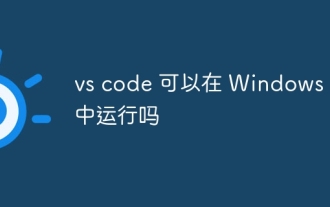
VS Code can run on Windows 8, but the experience may not be great. First make sure the system has been updated to the latest patch, then download the VS Code installation package that matches the system architecture and install it as prompted. After installation, be aware that some extensions may be incompatible with Windows 8 and need to look for alternative extensions or use newer Windows systems in a virtual machine. Install the necessary extensions to check whether they work properly. Although VS Code is feasible on Windows 8, it is recommended to upgrade to a newer Windows system for a better development experience and security.
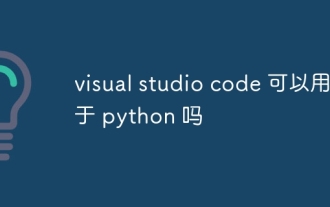
VS Code can be used to write Python and provides many features that make it an ideal tool for developing Python applications. It allows users to: install Python extensions to get functions such as code completion, syntax highlighting, and debugging. Use the debugger to track code step by step, find and fix errors. Integrate Git for version control. Use code formatting tools to maintain code consistency. Use the Linting tool to spot potential problems ahead of time.
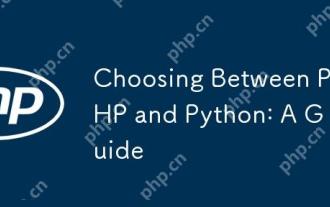
PHP is suitable for web development and rapid prototyping, and Python is suitable for data science and machine learning. 1.PHP is used for dynamic web development, with simple syntax and suitable for rapid development. 2. Python has concise syntax, is suitable for multiple fields, and has a strong library ecosystem.
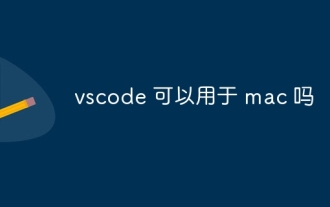
VS Code is available on Mac. It has powerful extensions, Git integration, terminal and debugger, and also offers a wealth of setup options. However, for particularly large projects or highly professional development, VS Code may have performance or functional limitations.
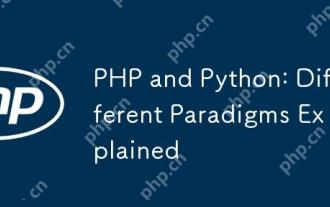
PHP is mainly procedural programming, but also supports object-oriented programming (OOP); Python supports a variety of paradigms, including OOP, functional and procedural programming. PHP is suitable for web development, and Python is suitable for a variety of applications such as data analysis and machine learning.
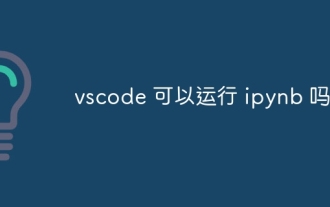
The key to running Jupyter Notebook in VS Code is to ensure that the Python environment is properly configured, understand that the code execution order is consistent with the cell order, and be aware of large files or external libraries that may affect performance. The code completion and debugging functions provided by VS Code can greatly improve coding efficiency and reduce errors.
