Real-time data processing using Kafka and Spark Streaming in Beego
With the continuous development of Internet and Internet of Things technology, the amount of data generated in our production and life is increasing. This data plays a very important role in the company's business strategy and decision-making. In order to better utilize this data, real-time data processing has become an important part of the daily work of enterprises and scientific research institutions. In this article, we will explore how to use Kafka and Spark Streaming in Beego framework for real-time data processing.
1. What is Kafka
Kafka is a high-throughput, distributed message queue system used to process massive data. Kafka stores message data in multiple topics in a distributed manner, and can be quickly retrieved and distributed. In the data streaming scenario, Kafka has become one of the most popular open source messaging systems and is widely used by many technology companies including LinkedIn, Netflix and Twitter.
2. What is Spark Streaming
Spark Streaming is a component in the Apache Spark ecosystem. It provides a streaming computing framework that can perform real-time batch processing of data streams. Spark Streaming is highly scalable and fault-tolerant, and can support multiple data sources. Spark Streaming can be used in conjunction with message queue systems such as Kafka to implement streaming computing functions.
3. Use Kafka and Spark Streaming in Beego for real-time data processing
When using the Beego framework for real-time data processing, we can combine Kafka and Spark Streaming to achieve data reception and processing. The following is a simple real-time data processing process:
1. Use Kafka to establish a message queue, encapsulate the data into messages and send them to Kafka.
2. Use Spark Streaming to build a streaming application and subscribe to data in the Kafka message queue.
3. For the subscribed data, we can perform various complex processing operations, such as data cleaning, data aggregation, business calculations, etc.
4. Output the processing results to Kafka or display them visually to the user.
Below we will introduce in detail how to implement the above process.
1. Establish a Kafka message queue
First, we need to introduce the Kafka package into Beego. You can use the sarama package in the go language and obtain it through the command:
go get gopkg.in/Shopify/sarama.v1
Then, establish a Kafka message queue in Beego and send the generated data to Kafka. The sample code is as follows:
func initKafka() (err error) {
//配置Kafka连接属性 config := sarama.NewConfig() config.Producer.RequiredAcks = sarama.WaitForAll config.Producer.Partitioner = sarama.NewRandomPartitioner config.Producer.Return.Successes = true //创建Kafka连接器 client, err := sarama.NewSyncProducer([]string{"localhost:9092"}, config) if err != nil { fmt.Println("failed to create producer, err:", err) return } //异步关闭Kafka defer client.Close() //模拟生成数据 for i := 1; i < 5000; i++ { id := uint32(i) userName := fmt.Sprintf("user:%d", i) //数据转为byte格式发送到Kafka message := fmt.Sprintf("%d,%s", id, userName) msg := &sarama.ProducerMessage{} msg.Topic = "test" //topic消息标记 msg.Value = sarama.StringEncoder(message) //消息数据 _, _, err := client.SendMessage(msg) if err != nil { fmt.Println("send message failed:", err) } time.Sleep(time.Second) } return
}
In the above code, we use the SyncProducer method in the Sarama package to create a Kafka connector and set the necessary connection properties. Then use a for loop to generate data, and encapsulate the generated data into messages and send them to Kafka.
2. Use Spark Streaming for real-time data processing
When using Spark Streaming for real-time data processing, we need to install and configure Spark and Kafka, which can be installed through the following command:
sudo apt-get install spark
sudo apt-get install zookeeper
sudo apt-get install kafka
After completing the installation, we need to introduce Spark Streaming into Beego Package:
import org.apache.spark.SparkConf
import org.apache.spark.streaming.{Seconds, StreamingContext}
import org.apache.spark. streaming.kafka.KafkaUtils
Next, we need to process the data stream. The following code implements the logic of receiving data from Kafka and processing each message:
func main() {
//创建SparkConf对象 conf := SparkConf().setAppName("test").setMaster("local[2]") //创建StreamingContext对象,设置1秒钟处理一次 ssc := StreamingContext(conf, Seconds(1)) //从Kafka中订阅test主题中的数据 zkQuorum := "localhost:2181" group := "test-group" topics := map[string]int{"test": 1} directKafkaStream, err := KafkaUtils.CreateDirectStream(ssc, topics, zkQuorum, group) if err != nil { panic(err) } lines := directKafkaStream.Map(func(message *sarama.ConsumerMessage) (string, int) { //从消息中解析出需要的数据 data := message.Value arr := strings.Split(string(data), ",") id, _ := strconv.Atoi(arr[0]) name := arr[1] return name, 1 }) //使用reduceByKey函数对数据进行聚合计算 counts := lines.ReduceByKey(func(a, b int) int { return a + b }) counts.Print() //开启流式处理 ssc.Start() ssc.AwaitTermination()
}
In the above code, we Use the SparkConf method and StreamingContext method to create a Spark Streaming context and set the processing time interval of the data stream. Then we subscribe to the data in the Kafka message queue, use the Map method to parse the required data from the received message, and then use the ReduceByKey method to perform data aggregation calculations. Finally, the calculation results are printed to the console.
4. Summary
This article introduces how to use Kafka and Spark Streaming in the Beego framework for real-time data processing. By establishing a Kafka message queue and using Spark Streaming to process the data stream, a streamlined and efficient real-time data processing process can be achieved. This processing method has been widely used in various fields and provides an important reference for corporate decision-making.
The above is the detailed content of Real-time data processing using Kafka and Spark Streaming in Beego. For more information, please follow other related articles on the PHP Chinese website!

Hot AI Tools

Undresser.AI Undress
AI-powered app for creating realistic nude photos

AI Clothes Remover
Online AI tool for removing clothes from photos.

Undress AI Tool
Undress images for free

Clothoff.io
AI clothes remover

AI Hentai Generator
Generate AI Hentai for free.

Hot Article

Hot Tools

Notepad++7.3.1
Easy-to-use and free code editor

SublimeText3 Chinese version
Chinese version, very easy to use

Zend Studio 13.0.1
Powerful PHP integrated development environment

Dreamweaver CS6
Visual web development tools

SublimeText3 Mac version
God-level code editing software (SublimeText3)

Hot Topics


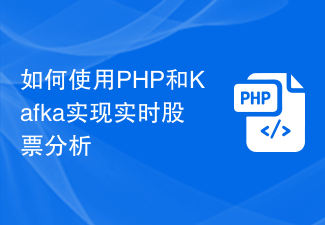
With the development of the Internet and technology, digital investment has become a topic of increasing concern. Many investors continue to explore and study investment strategies, hoping to obtain a higher return on investment. In stock trading, real-time stock analysis is very important for decision-making, and the use of Kafka real-time message queue and PHP technology is an efficient and practical means. 1. Introduction to Kafka Kafka is a high-throughput distributed publish and subscribe messaging system developed by LinkedIn. The main features of Kafka are
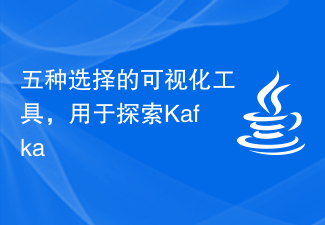
Five options for Kafka visualization tools ApacheKafka is a distributed stream processing platform capable of processing large amounts of real-time data. It is widely used to build real-time data pipelines, message queues, and event-driven applications. Kafka's visualization tools can help users monitor and manage Kafka clusters and better understand Kafka data flows. The following is an introduction to five popular Kafka visualization tools: ConfluentControlCenterConfluent
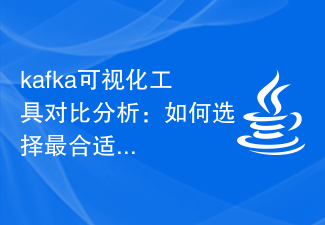
How to choose the right Kafka visualization tool? Comparative analysis of five tools Introduction: Kafka is a high-performance, high-throughput distributed message queue system that is widely used in the field of big data. With the popularity of Kafka, more and more enterprises and developers need a visual tool to easily monitor and manage Kafka clusters. This article will introduce five commonly used Kafka visualization tools and compare their features and functions to help readers choose the tool that suits their needs. 1. KafkaManager
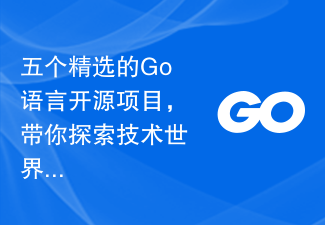
In today's era of rapid technological development, programming languages are springing up like mushrooms after a rain. One of the languages that has attracted much attention is the Go language, which is loved by many developers for its simplicity, efficiency, concurrency safety and other features. The Go language is known for its strong ecosystem with many excellent open source projects. This article will introduce five selected Go language open source projects and lead readers to explore the world of Go language open source projects. KubernetesKubernetes is an open source container orchestration engine for automated
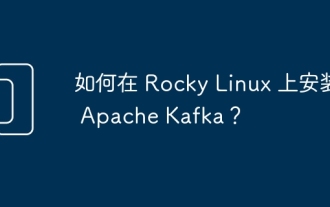
To install ApacheKafka on RockyLinux, you can follow the following steps: Update system: First, make sure your RockyLinux system is up to date, execute the following command to update the system package: sudoyumupdate Install Java: ApacheKafka depends on Java, so you need to install JavaDevelopmentKit (JDK) first ). OpenJDK can be installed through the following command: sudoyuminstalljava-1.8.0-openjdk-devel Download and decompress: Visit the ApacheKafka official website () to download the latest binary package. Choose a stable version
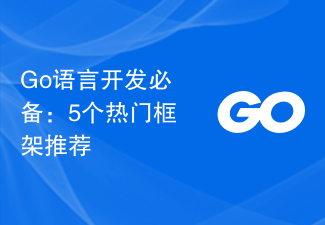
"Go Language Development Essentials: 5 Popular Framework Recommendations" As a fast and efficient programming language, Go language is favored by more and more developers. In order to improve development efficiency and optimize code structure, many developers choose to use frameworks to quickly build applications. In the world of Go language, there are many excellent frameworks to choose from. This article will introduce 5 popular Go language frameworks and provide specific code examples to help readers better understand and use these frameworks. 1.GinGin is a lightweight web framework with fast
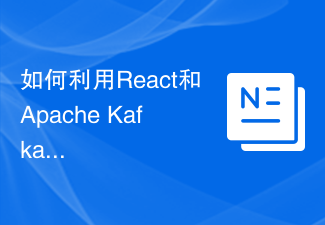
How to use React and Apache Kafka to build real-time data processing applications Introduction: With the rise of big data and real-time data processing, building real-time data processing applications has become the pursuit of many developers. The combination of React, a popular front-end framework, and Apache Kafka, a high-performance distributed messaging system, can help us build real-time data processing applications. This article will introduce how to use React and Apache Kafka to build real-time data processing applications, and
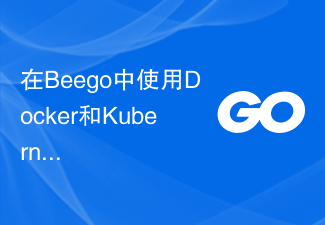
With the rapid development of the Internet, more and more enterprises have begun to migrate their applications to cloud platforms. Docker and Kubernetes have become two very popular and powerful tools for application deployment and management on cloud platforms. Beego is a web framework developed using Golang. It provides rich functions such as HTTP routing, MVC layering, logging, configuration management, Session management, etc. In this article we will cover how to use Docker and Kub
