


Discussion on best practices in data capture and processing using Scrapy framework
Discussion on the best practices of Scrapy framework in data capture and processing
In the Internet era, data has become money, and collecting data has also become a need for many companies and individuals. Capturing data is the most basic part of it. The Scrapy framework, as a crawler framework in Python, is widely used in the field of data capture and processing. This article will discuss the best practices of Scrapy framework in data capture and processing.
1. Fetching data
1.1. Selector
The method of fetching data in the Scrapy framework is through Selector, which uses XPath or CSS selectors to document Parsed class. In the process of using Selector, you need to have a corresponding understanding of XPath or CSS selector syntax in order to improve the accuracy of data capture.
1.2. Pipeline
In the Scrapy framework, Pipeline is a data processing pipeline. The captured data is processed by Pipeline and can be stored in a database or saved to a file. In Pipeline, you can write custom processing logic to clean and filter data to improve data accuracy and usability.
1.3. Request
Request in the Scrapy framework is a class used to obtain page data. Through Request, you can set the corresponding URL address, request method, request header, request parameters and other information. In the process of using Request, you need to have a corresponding understanding of the HTTP protocol in order to specify appropriate request parameters and improve the accuracy and speed of data capture.
2. Processing data
2.1. Item
The Item in the Scrapy framework is returned to the Pipeline for processing as the result of data capture. In the process of capturing data, the format of the Item needs to be defined first so that the Pipeline can process it. In the process of defining Items, it is necessary to design accordingly according to the characteristics of the specific data in order to improve the usability of the data.
2.2. Middleware
Middleware in the Scrapy framework refers to a class that performs a series of customized processing of requests and responses. Requests and responses can be intercepted, modified and filtered through Middleware. In the process of data processing, the data can be further filtered and processed through Middleware to improve the accuracy and usability of the data.
2.3. Output
Output in the Scrapy framework refers to the way to output data. During the process of data processing in the Pipeline, the data can be stored in the database or saved in a file. In the process of data output, it is necessary to design accordingly according to the characteristics of the data in order to improve the readability and usability of the data.
3. Performance Optimization
3.1. Asynchronousization
Asynchronousization in the Scrapy framework refers to the use of asynchronous methods for data capture and processing. Through asynchronousization, the data capture speed and processing efficiency can be improved, and the performance of data processing can be further improved.
3.2. Distribution
Distribution in the Scrapy framework refers to distributing data capture and processing to multiple servers. Through distribution, the data capture speed and processing efficiency can be improved, and the performance of data processing can be further improved.
3.3. Caching
Caching in the Scrapy framework refers to caching the results of data crawling into a local or distributed cache. Caching can reduce visits to the website and improve data crawling speed and processing efficiency.
Conclusion
The Scrapy framework is widely used in the field of data capture and processing. It has the advantages of fast crawling speed, high data processing efficiency, and strong scalability. In the process of using the Scrapy framework for data capture and processing, it is necessary to reasonably use the functions of the Scrapy framework such as Selector, Pipeline, Request, Item, Middleware, and Output for data capture and processing. At the same time, we also need to pay attention to performance optimization and use asynchronous, distributed, cache and other methods to improve the performance and efficiency of data processing to better meet user needs.
The above is the detailed content of Discussion on best practices in data capture and processing using Scrapy framework. For more information, please follow other related articles on the PHP Chinese website!

Hot AI Tools

Undresser.AI Undress
AI-powered app for creating realistic nude photos

AI Clothes Remover
Online AI tool for removing clothes from photos.

Undress AI Tool
Undress images for free

Clothoff.io
AI clothes remover

AI Hentai Generator
Generate AI Hentai for free.

Hot Article

Hot Tools

Notepad++7.3.1
Easy-to-use and free code editor

SublimeText3 Chinese version
Chinese version, very easy to use

Zend Studio 13.0.1
Powerful PHP integrated development environment

Dreamweaver CS6
Visual web development tools

SublimeText3 Mac version
God-level code editing software (SublimeText3)

Hot Topics


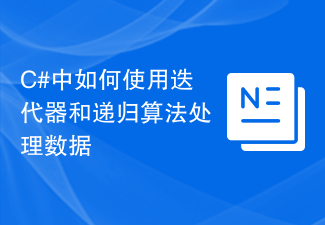
How to use iterators and recursive algorithms to process data in C# requires specific code examples. In C#, iterators and recursive algorithms are two commonly used data processing methods. Iterators can help us traverse the elements in a collection, and recursive algorithms can handle complex problems efficiently. This article details how to use iterators and recursive algorithms to process data, and provides specific code examples. Using Iterators to Process Data In C#, we can use iterators to iterate over the elements in a collection without knowing the size of the collection in advance. Through the iterator, I
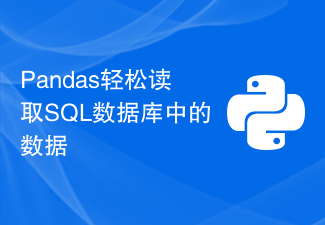
Data processing tool: Pandas reads data in SQL databases and requires specific code examples. As the amount of data continues to grow and its complexity increases, data processing has become an important part of modern society. In the data processing process, Pandas has become one of the preferred tools for many data analysts and scientists. This article will introduce how to use the Pandas library to read data from a SQL database and provide some specific code examples. Pandas is a powerful data processing and analysis tool based on Python
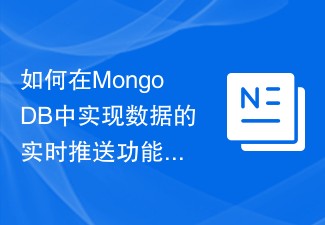
How to implement real-time data push function in MongoDB MongoDB is a document-oriented NoSQL database, which is characterized by high scalability and flexible data model. In some application scenarios, we need to push data updates to the client in real time in order to update the interface or perform corresponding operations in a timely manner. This article will introduce how to implement the real-time push function of data in MongoDB and give specific code examples. There are many ways to implement real-time push functionality, such as using polling, long polling, Web
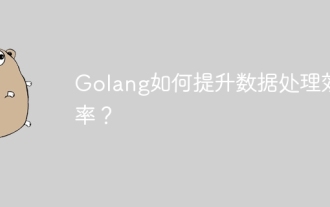
Golang improves data processing efficiency through concurrency, efficient memory management, native data structures and rich third-party libraries. Specific advantages include: Parallel processing: Coroutines support the execution of multiple tasks at the same time. Efficient memory management: The garbage collection mechanism automatically manages memory. Efficient data structures: Data structures such as slices, maps, and channels quickly access and process data. Third-party libraries: covering various data processing libraries such as fasthttp and x/text.
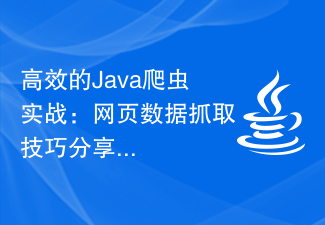
Java crawler practice: How to efficiently crawl web page data Introduction: With the rapid development of the Internet, a large amount of valuable data is stored in various web pages. To obtain this data, it is often necessary to manually access each web page and extract the information one by one, which is undoubtedly a tedious and time-consuming task. In order to solve this problem, people have developed various crawler tools, among which Java crawler is one of the most commonly used. This article will lead readers to understand how to use Java to write an efficient web crawler, and demonstrate the practice through specific code examples. 1. The base of the reptile
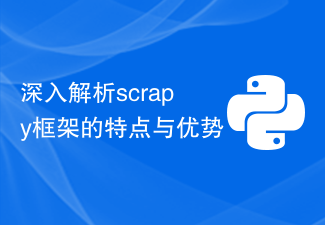
The Scrapy framework is an open source Python crawler framework that can be used to create and manage applications that crawl data. It is one of the most popular crawler frameworks currently on the market. The Scrapy framework uses asynchronous IO for network requests, which can efficiently capture website data and has the advantages of scalability and stability. This article will deeply analyze the characteristics and advantages of the Scrapy framework, and illustrate its efficient and stable operation through specific code examples. Simple and easy to learn Scrapy framework uses Python language, learn
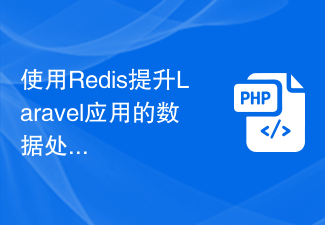
Use Redis to improve the data processing efficiency of Laravel applications. With the continuous development of Internet applications, data processing efficiency has become one of the focuses of developers. When developing applications based on the Laravel framework, we can use Redis to improve data processing efficiency and achieve fast access and caching of data. This article will introduce how to use Redis for data processing in Laravel applications and provide specific code examples. 1. Introduction to Redis Redis is a high-performance memory data
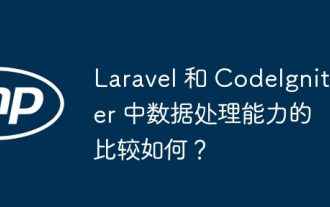
Compare the data processing capabilities of Laravel and CodeIgniter: ORM: Laravel uses EloquentORM, which provides class-object relational mapping, while CodeIgniter uses ActiveRecord to represent the database model as a subclass of PHP classes. Query builder: Laravel has a flexible chained query API, while CodeIgniter’s query builder is simpler and array-based. Data validation: Laravel provides a Validator class that supports custom validation rules, while CodeIgniter has less built-in validation functions and requires manual coding of custom rules. Practical case: User registration example shows Lar
