


How to write a recommendation system-based social network application using Java
In modern social network applications, recommendation systems have become an essential function. Whether it is recommending friends to users, recommending topics of interest, recommending related products, or recommending more valuable content, recommendation systems can effectively improve user experience and stickiness.
In this article, we will introduce how to use Java to write a social network application based on a recommendation system. We will combine actual code and detailed steps to help readers quickly understand and implement a basic recommendation system.
1. Data collection and processing
Before implementing any recommendation system, we need to collect and process a large amount of data. In social network applications, user information, posts, comments, likes and other data are all valuable sources of data.
To facilitate demonstration, we can use an open source virtual data generator to generate these data. The specific steps are as follows:
- Download and install a virtual data generator, such as Mockaroo (https://www.mockaroo.com/).
- Define the data sets that need to be generated, including user information, posts, comments, etc.
- Generate data and export to CSV file.
- Use Java code to read the data in the CSV file and store it in the database. We can use popular relational databases such as MySQL and Oracle to store data. Here, we use MySQL 8.0 as the database for data storage.
2. Representation of users and items
In the recommendation system, we need to convert users and items into vector or matrix form in order to calculate their similarity or Make recommendations. In social network applications, we can use the following methods to represent users and items:
- User vector: We can use data such as the topics that users follow, posts they publish, and friends they interact with to represent them. A vector of users. For example, if a user A follows the topics Java, Python, JavaScript, etc., posts "How to learn Java well" and "Getting started with Java", and interacts with users B and C, then we can use the following vector to represent user A:
User A = [1, 1, 1, 1, 1, 1, 1, 1, 0, 0, 1, 1, 0, 0, 1, 1, 1, 0, 0 , 0, 1, 0, 0, 1]
The vector length is 24, and each position represents a topic or post. 1 means that user A has followed the topic or published the post, and 0 means not.
- Item vector: We can use the tags, content, comments and other data of each post to represent the vector of a post. For example, if a post is tagged "Java, programming" and the content is "Four suggestions for learning Java programming" and has 10 comments, then we can use the following vector to represent the post:
Post A = [1, 1, 1, 0, 0, 1, 0, 0, 0, 0, 0, 0, 0, 0, 0, 0, 0, 0, 0, 0, 0, 0, 10 , 0]
The vector length is 24, and each position represents a label or statistical data. 1 means the post contains the tag or content, 0 means it does not.
3. User-based collaborative filtering recommendation
User-based collaborative filtering is a common method in recommendation systems. It recommends items based on the similarity of user interests. Here, we use user-based collaborative filtering to recommend suitable posts for users. The specific steps are as follows:
- Calculate the similarity between users. Here, we use the Pearson correlation coefficient as the similarity metric.
- Select K users with the highest interest similarity to the target user.
- For each user, select N posts that they like but have not been seen by the target user.
- For the selected N posts, calculate the recommendation score of each post and sort them from high to low.
- Select the top M posts with the highest scores as recommended results.
The following is the Java code implementation of the algorithm:
public class CollaborativeFiltering { /** * 计算用户间的皮尔逊相关系数 * @param user1 用户1 * @param user2 用户2 * @param data 数据集 * @return 皮尔逊相关系数 */ public double pearsonCorrelation(Map<Integer, Double> user1, Map<Integer, Double> user2, Map<Integer, Map<Integer, Double>> data) { double sum1 = 0, sum2 = 0, sum1Sq = 0, sum2Sq = 0, pSum = 0; int n = 0; for (int item : user1.keySet()) { if (user2.containsKey(item)) { sum1 += user1.get(item); sum2 += user2.get(item); sum1Sq += Math.pow(user1.get(item), 2); sum2Sq += Math.pow(user2.get(item), 2); pSum += user1.get(item) * user2.get(item); n++; } } if (n == 0) return 0; double num = pSum - (sum1 * sum2 / n); double den = Math.sqrt((sum1Sq - Math.pow(sum1, 2) / n) * (sum2Sq - Math.pow(sum2, 2) / n)); if (den == 0) return 0; return num / den; } /** * 基于用户的协同过滤推荐算法 * @param data 数据集 * @param userId 目标用户 ID * @param K 最相似的 K 个用户 * @param N 推荐的 N 个帖子 * @return 推荐的帖子 ID 列表 */ public List<Integer> userBasedCollaborativeFiltering(Map<Integer, Map<Integer, Double>> data, int userId, int K, int N) { Map<Integer, Double> targetUser = data.get(userId); // 目标用户 List<Map.Entry<Integer, Double>> similarUsers = new ArrayList<>(); // 与目标用户兴趣相似的用户 for (Map.Entry<Integer, Map<Integer, Double>> entry: data.entrySet()) { int id = entry.getKey(); if (id == userId) continue; double sim = pearsonCorrelation(targetUser, entry.getValue(), data); // 计算皮尔逊相关系数 if (sim > 0) similarUsers.add(new AbstractMap.SimpleEntry<>(id, sim)); } Collections.sort(similarUsers, (a, b) -> b.getValue().compareTo(a.getValue())); // 按相似度从高到低排序 List<Integer> itemIds = new ArrayList<>(); for (int i = 0; i < K && i < similarUsers.size(); i++) { Map.Entry<Integer, Double> entry = similarUsers.get(i); int userId2 = entry.getKey(); Map<Integer, Double> user2 = data.get(userId2); for (int itemId: user2.keySet()) { if (!targetUser.containsKey(itemId)) { // 如果目标用户没看过该帖子 itemIds.add(itemId); } } } Map<Integer, Double> scores = new HashMap<>(); for (int itemId: itemIds) { double score = 0; int count = 0; for (Map.Entry<Integer, Double> entry: similarUsers) { int userId2 = entry.getKey(); Map<Integer, Double> user2 = data.get(userId2); if (user2.containsKey(itemId)) { // 如果用户 2 看过该帖子 score += entry.getValue() * user2.get(itemId); count++; if (count == N) break; } } scores.put(itemId, score); } List<Integer> pickedItemIds = new ArrayList<>(); scores.entrySet().stream().sorted((a, b) -> b.getValue().compareTo(a.getValue())) .limit(N).forEach(entry -> pickedItemIds.add(entry.getKey())); // 按得分从高到低排序并选出前N个 return pickedItemIds; } }
4. Content-based recommendation algorithm
The content-based recommendation algorithm is another type of recommendation system. A common method that recommends items based on the similarity of their attributes. Here, we use a content-based recommendation algorithm to recommend suitable posts to users. The specific steps are as follows:
- For the target users, select the topics they follow, the posts they publish, etc.
- Based on these contents, calculate the similarity of each post to the target user's interests.
- Select the top N posts that are most similar to the target user’s interests.
- Sort according to the scores from high to low, and select the top M posts with the highest scores as the recommended results.
The following is the Java code implementation of the content-based recommendation algorithm:
public class ContentBasedRecommendation { /** * 计算两个向量的余弦相似度 * @param v1 向量1 * @param v2 向量2 * @return 余弦相似度 */ public double cosineSimilarity(double[] v1, double[] v2) { double dotProduct = 0; double norma = 0; double normb = 0; for (int i = 0; i < v1.length; i++) { dotProduct += v1[i] * v2[i]; norma += Math.pow(v1[i], 2); normb += Math.pow(v2[i], 2); } if (norma == 0 || normb == 0) return 0; return dotProduct / (Math.sqrt(norma) * Math.sqrt(normb)); } /** * 基于内容的推荐算法 * @param data 数据集 * @param userId 目标用户 ID * @param N 推荐的 N 个帖子 * @return 推荐的帖子 ID 列表 */ public List<Integer> contentBasedRecommendation(Map<Integer, Map<Integer, Double>> data, int userId, int N) { Map<Integer, Double> targetUser = data.get(userId); // 目标用户 int[] pickedItems = new int[data.size()]; double[][] itemFeatures = new double[pickedItems.length][24]; // 物品特征矩阵 for (Map.Entry<Integer, Map<Integer, Double>> entry: data.entrySet()) { int itemId = entry.getKey(); Map<Integer, Double> item = entry.getValue(); double[] feature = new double[24]; for (int i = 0; i < feature.length; i++) { if (item.containsKey(i+1)) { feature[i] = item.get(i+1); } else { feature[i] = 0; } } itemFeatures[itemId-1] = feature; // 物品 ID 从 1 开始,需要减一 } for (int itemId: targetUser.keySet()) { pickedItems[itemId-1] = 1; // 物品 ID 从 1 开始,需要减一 } double[] similarities = new double[pickedItems.length]; for (int i = 0; i < similarities.length; i++) { if (pickedItems[i] == 0) { similarities[i] = cosineSimilarity(targetUser.values().stream().mapToDouble(Double::doubleValue).toArray(), itemFeatures[i]); } } List<Integer> itemIds = new ArrayList<>(); while (itemIds.size() < N) { int maxIndex = -1; for (int i = 0; i < similarities.length; i++) { if (pickedItems[i] == 0 && (maxIndex == -1 || similarities[i] > similarities[maxIndex])) { maxIndex = i; } } if (maxIndex == -1 || similarities[maxIndex] < 0) { break; // 找不到更多相似的物品了 } itemIds.add(maxIndex + 1); // 物品 ID 从 1 开始,需要加一 pickedItems[maxIndex] = 1; } Map<Integer, Double> scores = new HashMap<>(); for (int itemId: itemIds) { double[] features = itemFeatures[itemId-1]; // 物品 ID 从 1 开始,需要减一 double score = cosineSimilarity(targetUser.values().stream().mapToDouble(Double::doubleValue).toArray(), features); scores.put(itemId, score); } List<Integer> pickedItemIds = new ArrayList<>(); scores.entrySet().stream().sorted((a, b) -> b.getValue().compareTo(a.getValue())) .limit(N).forEach(entry -> pickedItemIds.add(entry.getKey())); // 按得分从高到低排序并选出前N个 return pickedItemIds; } }
5. Integrate the recommendation algorithm into the application
After completing the above two recommendation algorithms Once implemented, we can integrate them into the application. The specific steps are as follows:
- Load data and store it in the database. We can use ORM frameworks such as Hibernate to simplify the operation of accessing the database.
- Define a RESTful API that accepts HTTP requests and returns responses in JSON format. We can use Spring Framework to build and deploy RESTful APIs.
- Implement user-based collaborative filtering recommendation and content-based recommendation algorithms and integrate them into RESTful API.
The following is the Java code implementation of the application:
@RestController @RequestMapping("/recommendation") public class RecommendationController { private CollaborativeFiltering collaborativeFiltering = new CollaborativeFiltering(); private ContentBasedRecommendation contentBasedRecommendation = new ContentBasedRecommendation(); @Autowired private UserService userService; @GetMapping("/userbased/{userId}") public List<Integer> userBasedRecommendation(@PathVariable Integer userId) { List<User> allUsers = userService.getAllUsers(); Map<Integer, Map<Integer, Double>> data = new HashMap<>(); for (User user: allUsers) { Map<Integer, Double> userVector = new HashMap<>(); List<Topic> followedTopics = user.getFollowedTopics(); for (Topic topic: followedTopics) { userVector.put(topic.getId(), 1.0); } List<Post> posts = user.getPosts(); for (Post post: posts) { userVector.put(post.getId() + 1000, 1.0); } List<Comment> comments = user.getComments(); for (Comment comment: comments) { userVector.put(comment.getId() + 2000, 1.0); } List<Like> likes = user.getLikes(); for (Like like: likes) { userVector.put(like.getId() + 3000, 1.0); } data.put(user.getId(), userVector); } List<Integer> itemIds = collaborativeFiltering.userBasedCollaborativeFiltering(data, userId, 5, 10); return itemIds; } @GetMapping("/contentbased/{userId}") public List<Integer> contentBasedRecommendation(@PathVariable Integer userId) { List<User> allUsers = userService.getAllUsers(); Map<Integer, Map<Integer, Double>> data = new HashMap<>(); for (User user: allUsers) { Map<Integer, Double> userVector = new HashMap<>(); List<Topic> followedTopics = user.getFollowedTopics(); for (Topic topic: followedTopics) { userVector.put(topic.getId(), 1.0); } List<Post> posts = user.getPosts(); for (Post post: posts) { userVector.put(post.getId() + 1000, 1.0); } List<Comment> comments = user.getComments(); for (Comment comment: comments) { userVector.put(comment.getId() + 2000, 1.0); } List<Like> likes = user.getLikes(); for (Like like: likes) { userVector.put(like.getId() + 3000, 1.0); }
The above is the detailed content of How to write a recommendation system-based social network application using Java. For more information, please follow other related articles on the PHP Chinese website!

Hot AI Tools

Undresser.AI Undress
AI-powered app for creating realistic nude photos

AI Clothes Remover
Online AI tool for removing clothes from photos.

Undress AI Tool
Undress images for free

Clothoff.io
AI clothes remover

Video Face Swap
Swap faces in any video effortlessly with our completely free AI face swap tool!

Hot Article

Hot Tools

Notepad++7.3.1
Easy-to-use and free code editor

SublimeText3 Chinese version
Chinese version, very easy to use

Zend Studio 13.0.1
Powerful PHP integrated development environment

Dreamweaver CS6
Visual web development tools

SublimeText3 Mac version
God-level code editing software (SublimeText3)

Hot Topics


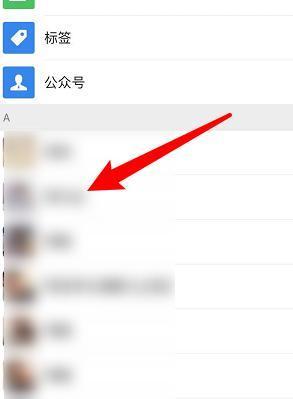
Unfortunately, people often delete certain contacts accidentally for some reasons. WeChat is a widely used social software. To help users solve this problem, this article will introduce how to retrieve deleted contacts in a simple way. 1. Understand the WeChat contact deletion mechanism. This provides us with the possibility to retrieve deleted contacts. The contact deletion mechanism in WeChat removes them from the address book, but does not delete them completely. 2. Use WeChat’s built-in “Contact Book Recovery” function. WeChat provides “Contact Book Recovery” to save time and energy. Users can quickly retrieve previously deleted contacts through this function. 3. Enter the WeChat settings page and click the lower right corner, open the WeChat application "Me" and click the settings icon in the upper right corner to enter the settings page.
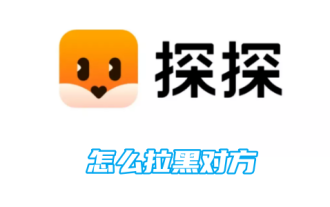
How to block someone in Tantan? In Tantan, you can choose your friends to block them directly. Most users don’t know how to block their friends in Tantan. Next, here is a picture of how to block someone in Tantan. Text tutorial, interested users come and take a look! How to block the other party on Tantan 1. First, unlock the phone, open the desktop and click [Tantan] APP to enter the main page; 2. Then on the Tantan message main page, click on the avatar of the friend you want to block; 3. Then enter the picture below In the interface shown, use the three-dot icon in the upper right corner to enter the special area; 4. Finally, an option box will pop up at the bottom, find [Add to Blacklist] and click to blacklist the other party.
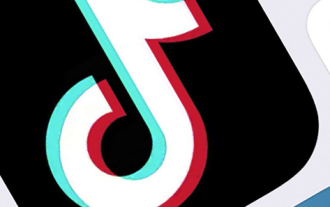
On the vast platform of Douyin, every user likes to use personalized nicknames to express themselves, or to show their inner yearning, or to convey their love for things, but sometimes they find that a friend may be their real-life friend. This aroused everyone's curiosity and wanted to confirm whether the real name was someone they knew. So how to check the real name in Douyin? The following tutorial will give you a detailed introduction, I hope it can help you. How to check the other person's real name on Douyin. The first step is to open the follow list of Douyin app and click on the avatar of the friend you want to view. Step 2: Then we go to the user homepage and click on the note name. Step 3: Then in the pop-up menu, click "View Names"
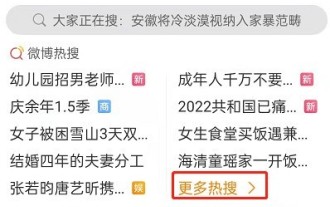
1. Open Weibo, click Discover, click More Hot Searches, and find the hot search list (as shown in the picture). 2. Select a hot search topic to enter (as shown in the picture). 3. Click "Discuss Together" below the hot search, and post on Weibo with the hot search topic (as shown in the picture). Method 2: Complete your personal information. 1. Open Weibo, enter your personal homepage, and click the down button icon (as shown in the picture). 2. Click to view and edit basic information and enter editing (as shown in the picture). Method 3: Have more users with high credibility, big V and small V fans, and interact with each other. 1. Open the homepage, see some influential bloggers, and click on the comments below to interact (as shown in the picture). Method 4: Participate in charity donations on Weibo. 1. Click My to enter my wallet (as shown in the picture). 2:00
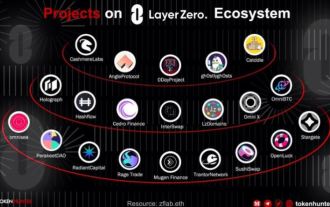
What other airdrop projects are worthy of your attention in 2024.5? A list of six airdrop projects worthy of attention! Several airdrop chasers in May are turning to other targets — DeFi protocols without native tokens. This expectation often causes liquidity to flood into the platform as users prepare for the airdrop. While the current market slowdown hampered crypto tokens’ price gains earlier this year, here are some projects attracting hope. Today, the editor of this website will introduce to you in detail six airdrop projects worthy of your attention. I wish you all to make money soon! Airdrop hopefuls continue to develop tokenless projects. Cryptocurrencies are driving investor deposits. Airdrop recipients were not swayed by the project team’s attempts to deny the possibility of token distribution. April is an important month for airdrops
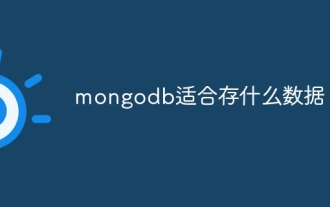
MongoDB is suitable for storing various types of data, including: Unstructured and semi-structured data Data with complex relationships Big data Data sets Time series data Geospatial data Others: Binary data, web data, metadata
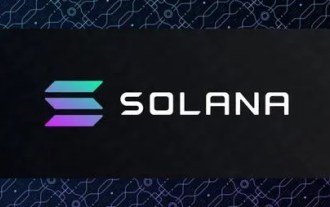
Solana and Bitcoin differ in blockchain architecture, transaction speed and cost, scalability, smart contracts, use cases, market cap and circulating supply. Solana has higher scalability, low transaction costs and support for smart contracts, while Bitcoin is known for its security, decentralization and properties as a store of value. Depending on different needs and preferences, these two cryptocurrencies can serve different market needs.
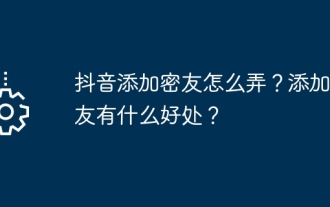
As Douyin becomes more popular, more and more people are starting to make new friends on the platform. The addition of close friends function on Douyin provides more possibilities for interaction between users. So, how can you add close friends on TikTok? 1. How to add close friends on Douyin? 1. Open the Douyin App, enter the homepage, and click the "Me" button in the lower right corner to enter the personal center. 2. On the personal center page, find the "Add Close Friends on Douyin" option and click to enter. 3. On the add close friends page, you can add the friends you want by searching for Douyin accounts, mobile phone contacts, WeChat friends, etc. 4. Enter the Douyin account or mobile phone number of the friend you want to add, and click the search button. 5. The search results show friends who meet the conditions, and you can choose to add them as close friends. 6.
