


NTU and Shanghai AI Lab compiled 300+ papers: the latest review of visual segmentation based on Transformer is released
SAM (Segment Anything), as a basic visual segmentation model, has attracted the attention and follow-up of many researchers in just 3 months. If you want to systematically understand the technology behind SAM, keep up with the pace of involution, and be able to make your own SAM model, then this Transformer-Based Segmentation Survey is not to be missed! Recently, several researchers from Nanyang Technological University and Shanghai Artificial Intelligence Laboratory wrote a review about Transformer-Based Segmentation, systematically reviewing the segmentation and detection models based on Transformer in recent years, and conducting research. The latest model is as of June this year! At the same time, the review also includes the latest papers in related fields and a large number of experimental analyzes and comparisons, and reveals a number of future research directions with broad prospects!
Visual segmentation aims to segment images, video frames or point clouds into multiple segments or groups. This technology has many real-world applications, such as autonomous driving, image editing, robot perception, and medical analysis. Over the past decade, deep learning-based methods have made significant progress in this field. Recently, Transformer has become a neural network based on a self-attention mechanism, originally designed for natural language processing, which significantly surpasses previous convolutional or recurrent methods in various visual processing tasks. Specifically, the Vision Transformer provides powerful, unified, and even simpler solutions for various segmentation tasks. This review provides a comprehensive overview of Transformer-based visual segmentation, summarizing recent advances. First, this article reviews the background, including problem definition, data sets, and previous convolution methods. Next, this paper summarizes a meta-architecture that unifies all recent Transformer-based methods. Based on this meta-architecture, this article studies various method designs, including modifications to this meta-architecture and related applications. In addition, this article also introduces several related settings, including 3D point cloud segmentation, basic model tuning, domain adaptive segmentation, efficient segmentation and medical segmentation. Furthermore, this paper compiles and re-evaluates these methods on several widely recognized datasets. Finally, the paper identifies open challenges in this field and proposes directions for future research. This article will continue and track the latest Transformer-based segmentation and detection methods.
Picture
Project address: https://github.com/lxtGH/Awesome-Segmentation-With-Transformer
Paper address: https://arxiv.org/pdf/2304.09854.pdf
Research motivation
- The emergence of ViT and DETR has made full progress in the field of segmentation and detection. Currently, the top-ranking methods on almost every data set benchmark are based on Transformer. For this reason, it is necessary to systematically summarize and compare the methods and technical characteristics of this direction.
- Recent large model architectures are all based on the Transformer structure, including multi-modal models and segmentation basic models (SAM), and various visual tasks are moving closer to unified model modeling.
- Segmentation and detection have derived many related downstream tasks, and many of these tasks are also solved using the Transformer structure.
Summary Features
- Systematic and readable. This article systematically reviews each task definition of segmentation, as well as related task definitions and evaluation indicators. And this article starts from the convolution method and summarizes a meta-architecture based on ViT and DETR. Based on this meta-architecture, this review summarizes and summarizes related methods, and systematically reviews recent methods. The specific technical review route is shown in Figure 1.
- Detailed classification from a technical perspective. Compared with previous Transformer reviews, this article’s classification of methods will be more detailed. This article brings together papers with similar ideas and compares their similarities and differences. For example, this article will classify methods that simultaneously modify the decoder side of the meta-architecture into image-based Cross Attention, and video-based spatio-temporal Cross Attention modeling.
- Comprehensiveness of the research question. This article will systematically review all directions of segmentation, including image, video, and point cloud segmentation tasks. At the same time, this article will also review related directions such as open set segmentation and detection models, unsupervised segmentation and weakly supervised segmentation.
Picture
Figure 1. Survey’s content roadmap
Figure 2. Summary of commonly used data sets and segmentation tasks
Summary of Transformer-Based segmentation and detection methods and Comparison
##Figure 3. General Meta-Architecture Framework
This article first summarizes a meta-architecture based on the DETR and MaskFormer frameworks. This model includes the following different modules:
- Backbone: Feature extractor, used to extract image features.
- Neck: Construct multi-scale features to handle multi-scale objects.
- Object Query: Query object, used to represent each entity in the scene, including foreground objects and background objects.
- Decoder: Decoder, used to gradually optimize Object Query and corresponding features.
- End-to-End Training: The design based on Object Query can achieve end-to-end optimization.
Based on this meta-architecture, existing methods can be divided into the following five different directions for optimization and adjustment according to tasks, as shown in Figure 4, each Directions have several different sub-directions.
Figure 4. Summary and comparison of Transformer-Based Segmentation methods
- Better feature expression learning, Representation Learning. Strong visual feature representation will always lead to better segmentation results. This article divides related work into three aspects: better visual Transformer design, hybrid CNN/Transformer/MLP, and self-supervised learning.
- Method design on the decoder side, Interaction Design in Decoder. This chapter reviews the new Transformer decoder design. This paper divides the decoder design into two groups: one is used to improve the cross-attention design in image segmentation, and the other is used to improve the spatio-temporal cross-attention design in video segmentation. The former focuses on designing a better decoder to improve upon the one in the original DETR. The latter extends query object-based object detectors and segmenters to the video domain for video object detection (VOD), video instance segmentation (VIS), and video pixel segmentation (VPS), focusing on modeling temporal consistency and correlation. sex.
- #Try to Optimizing Object Query from the perspective of query object optimization. Compared with Faster-RCNN, DETR requires a longer convergence timetable. Due to the key role of query objects, some existing methods have been studied to speed up training and improve performance. According to the method of object query, this paper divides the following literature into two aspects: adding location information and using additional supervision. Location information provides clues for fast training sampling of query features. Additional supervision focuses on designing specific loss functions in addition to the DETR default loss function.
- Use query objects to associate features and instances, Using Query For Association. Benefiting from the simplicity of query objects, multiple recent studies have used them as correlation tools to solve downstream tasks. There are two main usages: one is instance-level association, and the other is task-level association. The former uses the idea of instance discrimination to solve instance-level matching problems in videos, such as video segmentation and tracking. The latter uses query objects to bridge different subtasks to achieve efficient multi-task learning.
- Multi-modal conditional query object generation, Conditional Query Generation. This chapter mainly focuses on multi-modal segmentation tasks. Conditional query query objects are mainly used to handle cross-modal and cross-image feature matching tasks. Depending on the task input conditions, the decoder head uses different queries to obtain the corresponding segmentation masks. According to the sources of different inputs, this paper divides these works into two aspects: language features and image features. These methods are based on the strategy of fusing query objects with different model features, and have achieved good results in multiple multi-modal segmentation tasks and few-shot segmentation.
Figure 5 shows some representative work comparisons in these five different directions. For more specific method details and comparisons, please refer to the content of the paper.
Picture
Figure 5. Summary and comparison of Transformer-based segmentation and representativeness detection methods
Summary and comparison of methods in related research fields
This article also explores several related fields: 1. Point cloud segmentation method based on Transformer. 2. Vision and multi-modal large model tuning. 3. Research on domain-related segmentation models, including domain transfer learning and domain generalization learning. 4. Efficient semantic segmentation: unsupervised and weakly supervised segmentation models. 5. Class-independent segmentation and tracking. 6. Medical image segmentation.
Picture
Figure 6. Summary and comparison of Transformer-based methods in related research fields
Comparison of experimental results of different methods
Figure 7. Benchmark experiment of semantic segmentation data set
Figure 8. Benchmark experiment on panoramic segmentation data set
This article also uniformly uses the same experimental design conditions to compare the results of several representative works on panoramic segmentation and semantic segmentation on multiple data sets. It was found that when using the same training strategy and encoder, the gap between method performance will narrow.
In addition, this article also compares the results of recent Transformer-based segmentation methods on multiple different data sets and tasks. (Semantic segmentation, instance segmentation, panoramic segmentation, and corresponding video segmentation tasks)
Future Direction
In addition, this article also gives some Analysis of some possible future research directions. Three different directions are given here as examples.
- UpdateAdd a general and unified segmentation model. Using the Transformer structure to unify different segmentation tasks is a trend. Recent research uses query object-based Transformers to perform different segmentation tasks under one architecture. One possible research direction is to unify image and video segmentation tasks on various segmentation datasets through one model. These general models can achieve versatile and robust segmentation in various scenarios. For example, detecting and segmenting rare categories in various scenarios helps robots make better decisions.
- Segmentation model combined with visual reasoning. Visual reasoning requires the robot to understand the connections between objects in the scene, and this understanding plays a key role in motion planning. Previous research has explored using segmentation results as input to visual reasoning models for various applications such as object tracking and scene understanding. Joint segmentation and visual reasoning can be a promising direction, with mutually beneficial potential for both segmentation and relational classification. By incorporating visual reasoning into the segmentation process, researchers can leverage the power of reasoning to improve segmentation accuracy, while segmentation results can also provide better input for visual reasoning.
- Research on segmentation model of continuous learning. Existing segmentation methods are usually benchmarked on closed-world datasets with a predefined set of categories, i.e., it is assumed that the training and test samples have the same categories known in advance and feature space. However, real-world scenarios are often open-world and non-stable, and new categories of data may constantly emerge. For example, in autonomous vehicles and medical diagnostics, unanticipated situations may suddenly arise. There is a clear gap between the performance and capabilities of existing methods in real-world and closed-world scenarios. Therefore, it is hoped that new concepts can be gradually and continuously incorporated into the existing knowledge base of the segmentation model, so that the model can engage in lifelong learning.
For more research directions, please refer to the original paper.
The above is the detailed content of NTU and Shanghai AI Lab compiled 300+ papers: the latest review of visual segmentation based on Transformer is released. For more information, please follow other related articles on the PHP Chinese website!

Hot AI Tools

Undresser.AI Undress
AI-powered app for creating realistic nude photos

AI Clothes Remover
Online AI tool for removing clothes from photos.

Undress AI Tool
Undress images for free

Clothoff.io
AI clothes remover

AI Hentai Generator
Generate AI Hentai for free.

Hot Article

Hot Tools

Notepad++7.3.1
Easy-to-use and free code editor

SublimeText3 Chinese version
Chinese version, very easy to use

Zend Studio 13.0.1
Powerful PHP integrated development environment

Dreamweaver CS6
Visual web development tools

SublimeText3 Mac version
God-level code editing software (SublimeText3)

Hot Topics


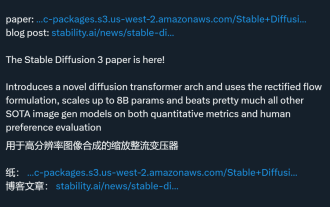
StableDiffusion3’s paper is finally here! This model was released two weeks ago and uses the same DiT (DiffusionTransformer) architecture as Sora. It caused quite a stir once it was released. Compared with the previous version, the quality of the images generated by StableDiffusion3 has been significantly improved. It now supports multi-theme prompts, and the text writing effect has also been improved, and garbled characters no longer appear. StabilityAI pointed out that StableDiffusion3 is a series of models with parameter sizes ranging from 800M to 8B. This parameter range means that the model can be run directly on many portable devices, significantly reducing the use of AI
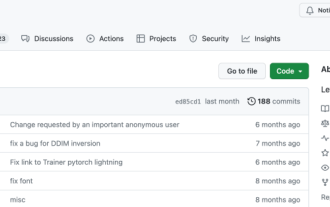
ICCV2023, the top computer vision conference held in Paris, France, has just ended! This year's best paper award is simply a "fight between gods". For example, the two papers that won the Best Paper Award included ControlNet, a work that subverted the field of Vincentian graph AI. Since being open sourced, ControlNet has received 24k stars on GitHub. Whether it is for diffusion models or the entire field of computer vision, this paper's award is well-deserved. The honorable mention for the best paper award was awarded to another equally famous paper, Meta's "Separate Everything" ”Model SAM. Since its launch, "Segment Everything" has become the "benchmark" for various image segmentation AI models, including those that came from behind.
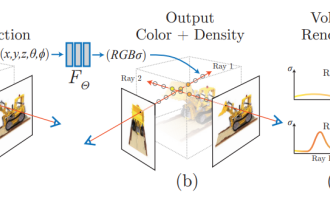
Since Neural Radiance Fields was proposed in 2020, the number of related papers has increased exponentially. It has not only become an important branch of three-dimensional reconstruction, but has also gradually become active at the research frontier as an important tool for autonomous driving. NeRF has suddenly emerged in the past two years, mainly because it skips the feature point extraction and matching, epipolar geometry and triangulation, PnP plus Bundle Adjustment and other steps of the traditional CV reconstruction pipeline, and even skips mesh reconstruction, mapping and light tracing, directly from 2D The input image is used to learn a radiation field, and then a rendered image that approximates a real photo is output from the radiation field. In other words, let an implicit three-dimensional model based on a neural network fit the specified perspective
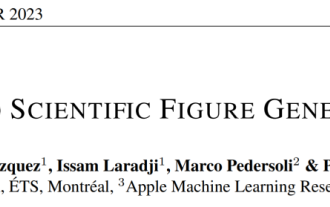
Generative AI has taken the artificial intelligence community by storm. Both individuals and enterprises have begun to be keen on creating related modal conversion applications, such as Vincent pictures, Vincent videos, Vincent music, etc. Recently, several researchers from scientific research institutions such as ServiceNow Research and LIVIA have tried to generate charts in papers based on text descriptions. To this end, they proposed a new method of FigGen, and the related paper was also included in ICLR2023 as TinyPaper. Picture paper address: https://arxiv.org/pdf/2306.00800.pdf Some people may ask, what is so difficult about generating the charts in the paper? How does this help scientific research?
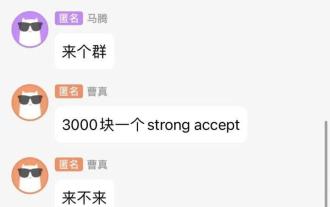
Just as the AAAI 2023 paper submission deadline was approaching, a screenshot of an anonymous chat in the AI submission group suddenly appeared on Zhihu. One of them claimed that he could provide "3,000 yuan a strong accept" service. As soon as the news came out, it immediately aroused public outrage among netizens. However, don’t rush yet. Zhihu boss "Fine Tuning" said that this is most likely just a "verbal pleasure". According to "Fine Tuning", greetings and gang crimes are unavoidable problems in any field. With the rise of openreview, the various shortcomings of cmt have become more and more clear. The space left for small circles to operate will become smaller in the future, but there will always be room. Because this is a personal problem, not a problem with the submission system and mechanism. Introducing open r
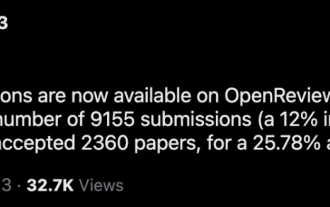
Just now, CVPR 2023 issued an article saying: This year, we received a record 9155 papers (12% more than CVPR2022), and accepted 2360 papers, with an acceptance rate of 25.78%. According to statistics, the number of submissions to CVPR only increased from 1,724 to 2,145 in the 7 years from 2010 to 2016. After 2017, it soared rapidly and entered a period of rapid growth. In 2019, it exceeded 5,000 for the first time, and by 2022, the number of submissions had reached 8,161. As you can see, a total of 9,155 papers were submitted this year, indeed setting a record. After the epidemic is relaxed, this year’s CVPR summit will be held in Canada. This year it will be a single-track conference and the traditional Oral selection will be cancelled. google research
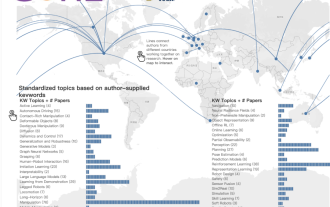
Since it was first held in 2017, CoRL has become one of the world's top academic conferences in the intersection of robotics and machine learning. CoRL is a single-theme conference for robot learning research, covering multiple topics such as robotics, machine learning and control, including theory and application. The 2023 CoRL Conference will be held in Atlanta, USA, from November 6th to 9th. According to official data, 199 papers from 25 countries were selected for CoRL this year. Popular topics include operations, reinforcement learning, and more. Although CoRL is smaller in scale than large AI academic conferences such as AAAI and CVPR, as the popularity of concepts such as large models, embodied intelligence, and humanoid robots increases this year, relevant research worthy of attention will also
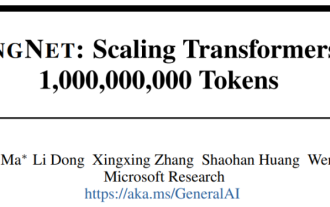
As everyone continues to upgrade and iterate their own large models, the ability of LLM (large language model) to process context windows has also become an important evaluation indicator. For example, the star model GPT-4 supports 32k tokens, which is equivalent to 50 pages of text; Anthropic, founded by a former member of OpenAI, has increased Claude's token processing capabilities to 100k, which is about 75,000 words, which is roughly equivalent to summarizing "Harry Potter" with one click "First. In Microsoft's latest research, they directly expanded Transformer to 1 billion tokens this time. This opens up new possibilities for modeling very long sequences, such as treating an entire corpus or even the entire Internet as one sequence. For comparison, common
