


BLIP-2 and InstructBLIP are firmly in the top three! Twelve major models, sixteen lists, comprehensive evaluation of 'multimodal large language model'
Multimodal Large Language Model (MLLM) relies on LLM’s rich knowledge reserves and powerful reasoning and generalization capabilities to solve multimodal problems. Some amazing models have emerged so far. Ability, such as reading pictures and writing and looking at pictures and writing code.
However, it is difficult to fully reflect the performance of MLLM based on these examples alone, and there is still a lack of comprehensive evaluation of MLLM.
To this end, Tencent Youtu Lab and Xiamen University conducted a comprehensive quantitative evaluation of the existing 12 open source MLLM models for the first time on the newly created evaluation benchmark MM and published 16 rankings List, including two general lists of perception and cognition and 14 sub-lists:
Paper link: https://arxiv.org/pdf /2306.13394.pdf
Project link: https://github.com/BradyFU/Awesome-Multimodal-Large-Language-Models/tree/Evaluation
The existing quantitative evaluation methods of MLLM are mainly divided into three categories, but they all have certain limitations that make it difficult to fully reflect their performance.
The first type of methods are evaluated on traditional public datasets, such as Image Caption and Visual Question Answering (VQA) datasets.
But on the one hand, these traditional data sets may be difficult to reflect the new capabilities of MLLM. On the other hand, since the training sets in the large model era are no longer unified, it is difficult to guarantee these evaluation data sets. It has not been trained by other MLLMs.
The second way is to collect new data for open evaluation, but these data are either not public [1] or the number is too small (only 50 pictures) [2].
The third method focuses on a specific aspect of MLLM, such as object hallucination [3] or adversarial robustness [4], and cannot be fully evaluated.
There is an urgent need for a comprehensive evaluation benchmark to match the rapid development of MLLM. Researchers believe that a universal comprehensive assessment benchmark should have the following characteristics:
(1) It should cover as much scope as possible, including perceptual and cognitive abilities. The former refers to identifying objects, including their existence, quantity, location and color. The latter refers to integrating sensory information and knowledge in LLM to perform more complex reasoning. The former is the basis of the latter.
(2) Data or annotations should avoid using existing public data sets as much as possible to reduce the risk of data leakage.
(3) Instructions should be as concise as possible and consistent with human cognitive habits. Different instruction designs may greatly affect the output of the model, but all models are evaluated under unified and concise instructions to ensure fairness. A good MLLM model should have the ability to generalize to such concise instructions to avoid falling into prompt engineering.
(4) The output of MLLM under this concise instruction should be intuitive and convenient for quantitative statistics. The open-ended answers of MLLM pose great challenges to quantitative statistics. Existing methods tend to use GPT or manual scoring, but may face problems of inaccuracy and subjectivity.
# Figure 1. MME evaluation benchmark example. Each picture corresponds to two questions, and the answers are Yes[Y] and No[N] respectively. The question plus "Please answer yes or no" together form the command.
Based on the above reasons, a new MLLM evaluation benchmark MME was constructed, which has the above four characteristics at the same time:
1. MME Perceptual and cognitive abilities are assessed simultaneously. In addition to OCR, sensing capabilities include coarse-grained and fine-grained target recognition. The former identifies the presence, quantity, location and color of objects. The latter identifies movie posters, celebrities, scenes, landmarks and artwork. Cognitive abilities include common sense reasoning, numerical calculations, text translation, and code reasoning. The total number of subtasks reaches 14, as shown in Figure 1.
2. All command-answer pairs in MME are constructed manually. For the few public datasets used, only their images are used without relying on their original annotations. At the same time, researchers also try their best to collect data through manual photography and image generation.
3. MME instructions are designed to be as concise as possible to avoid the impact of Prompt Engineering on model output. The researchers reiterate that a good MLLM should generalize to such concise and frequently used instructions, which is fair to all models. The instructions for each subtask are shown in Figure 1.
4. Thanks to the instruction design "Please answer yes or no", quantitative statistics can be easily performed based on the "Yes" or "No" output by the model. This method can simultaneously Ensure accuracy and objectivity. It is worth noting that researchers have also tried to design instructions for multiple-choice questions, but found that the current MLLM is still difficult to follow such more complex instructions.
The researchers evaluated a total of 12 advanced MLLM models, including BLIP-2 [5], LLaVA [6], MiniGPT-4 [7], mPLUG-Owl [2] , LLaMA-Adapter-v2 [8], Otter [9], Multimodal-GPT [10], InstructBLIP [11], VisualGLM-6B [12], PandaGPT [13], ImageBind-LLM [14] and LaVIN [15] .
There are three statistical indicators, including Accuracy, Accuracy and Score. For each task, Accuracy is based on question statistics, Accuracy is based on picture statistics (both questions corresponding to the pictures need to be answered correctly), and Score is the sum of Accuracy and Accuracy.
The total score of perception is the sum of the scores of 10 perceptual sub-tasks, and the total score of cognition is the sum of the scores of 4 cognitive tasks. See the project link for details.
The test comparison of 12 models on 14 sub-tasks is shown in Figure 2:
## Figure 2. Comparison of 12 models on 14 subtasks. The full score for each sub-task is 200 points.
A total of 16 lists, including the overall list of perception and cognition categories and the lists of 14 sub-tasks have also been released. The two overall lists are shown in Figures 3 and 4 respectively. It is worth noting that BLIP-2 and InstructBLIP remain in the top three in both lists.
Picture
Figure 3. Overall list of perception tasks
Figure 4. Overall list of cognitive tasks
Figure 5. All lists
In addition, the researchers also summarized some common problems exposed by the MLLM model in experiments, as shown in Figure 6, hoping to provide guidance for subsequent model optimization.
Picture
Figure 6. Common problems exposed by MLLM. [Y]/[N] means the real answer is Yes/No. [R] is the answer generated by MLLM.
The first problem is not following instructions.
Although a very concise instruction design has been adopted, there is still MLLM freedom to answer questions rather than follow instructions.
As shown in the first line of Figure 6, the command has stated "Please answer yes or no", but MLLM only gave a declarative answer. If "Yes" or "No" does not appear at the beginning of the answer, the answer is judged to be incorrect. A good MLLM, especially after fine-tuning the instructions, should be able to generalize to such simple instructions.
The second problem is the lack of perception.
As shown in the second row of Figure 6, MLLM incorrectly identifies the number of bananas in the first image and the second image numbers in, resulting in incorrect answers. The researchers also noticed that perceptual performance was easily affected by changes in instructions, as two instructions for the same picture that differed by just one word resulted in completely different perceptual results.
The third problem is the lack of reasoning ability.
As shown in the third line of Figure 6, it can be seen from the red text that MLLM already knows that the first picture is not an office space, but still gives Got an incorrect answer "Yes".
Similarly, in the second picture, MLLM has calculated the correct arithmetic result, but ultimately also gave the wrong answer. Adding a thought chain prompt, such as “Let’s think step by step” may bring better results. Looking forward to more in-depth research in this area.
The fourth question follows the object vision of the command. As shown in the fourth line of Figure 6, when the instruction contains an object that does not exist in the picture, MLLM will imagine that the object exists and finally give a "Yes" answer.
This approach of always answering "Yes" results in Accuracy close to 50% and Accuracy close to 0. This demonstrates the importance of suppressing target hallucinations and also requires further thinking about the reliability of answers generated by MLLM.
The above is the detailed content of BLIP-2 and InstructBLIP are firmly in the top three! Twelve major models, sixteen lists, comprehensive evaluation of 'multimodal large language model'. For more information, please follow other related articles on the PHP Chinese website!

Hot AI Tools

Undresser.AI Undress
AI-powered app for creating realistic nude photos

AI Clothes Remover
Online AI tool for removing clothes from photos.

Undress AI Tool
Undress images for free

Clothoff.io
AI clothes remover

AI Hentai Generator
Generate AI Hentai for free.

Hot Article

Hot Tools

Notepad++7.3.1
Easy-to-use and free code editor

SublimeText3 Chinese version
Chinese version, very easy to use

Zend Studio 13.0.1
Powerful PHP integrated development environment

Dreamweaver CS6
Visual web development tools

SublimeText3 Mac version
God-level code editing software (SublimeText3)

Hot Topics


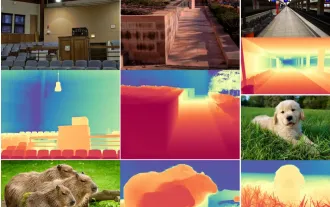
0.What does this article do? We propose DepthFM: a versatile and fast state-of-the-art generative monocular depth estimation model. In addition to traditional depth estimation tasks, DepthFM also demonstrates state-of-the-art capabilities in downstream tasks such as depth inpainting. DepthFM is efficient and can synthesize depth maps within a few inference steps. Let’s read about this work together ~ 1. Paper information title: DepthFM: FastMonocularDepthEstimationwithFlowMatching Author: MingGui, JohannesS.Fischer, UlrichPrestel, PingchuanMa, Dmytr
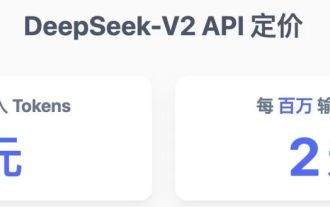
Imagine an artificial intelligence model that not only has the ability to surpass traditional computing, but also achieves more efficient performance at a lower cost. This is not science fiction, DeepSeek-V2[1], the world’s most powerful open source MoE model is here. DeepSeek-V2 is a powerful mixture of experts (MoE) language model with the characteristics of economical training and efficient inference. It consists of 236B parameters, 21B of which are used to activate each marker. Compared with DeepSeek67B, DeepSeek-V2 has stronger performance, while saving 42.5% of training costs, reducing KV cache by 93.3%, and increasing the maximum generation throughput to 5.76 times. DeepSeek is a company exploring general artificial intelligence
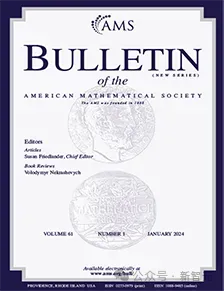
AI is indeed changing mathematics. Recently, Tao Zhexuan, who has been paying close attention to this issue, forwarded the latest issue of "Bulletin of the American Mathematical Society" (Bulletin of the American Mathematical Society). Focusing on the topic "Will machines change mathematics?", many mathematicians expressed their opinions. The whole process was full of sparks, hardcore and exciting. The author has a strong lineup, including Fields Medal winner Akshay Venkatesh, Chinese mathematician Zheng Lejun, NYU computer scientist Ernest Davis and many other well-known scholars in the industry. The world of AI has changed dramatically. You know, many of these articles were submitted a year ago.
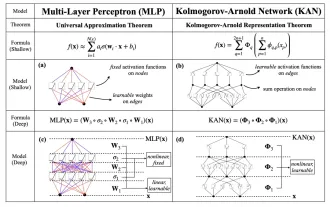
Earlier this month, researchers from MIT and other institutions proposed a very promising alternative to MLP - KAN. KAN outperforms MLP in terms of accuracy and interpretability. And it can outperform MLP running with a larger number of parameters with a very small number of parameters. For example, the authors stated that they used KAN to reproduce DeepMind's results with a smaller network and a higher degree of automation. Specifically, DeepMind's MLP has about 300,000 parameters, while KAN only has about 200 parameters. KAN has a strong mathematical foundation like MLP. MLP is based on the universal approximation theorem, while KAN is based on the Kolmogorov-Arnold representation theorem. As shown in the figure below, KAN has
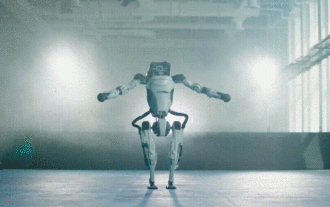
Boston Dynamics Atlas officially enters the era of electric robots! Yesterday, the hydraulic Atlas just "tearfully" withdrew from the stage of history. Today, Boston Dynamics announced that the electric Atlas is on the job. It seems that in the field of commercial humanoid robots, Boston Dynamics is determined to compete with Tesla. After the new video was released, it had already been viewed by more than one million people in just ten hours. The old people leave and new roles appear. This is a historical necessity. There is no doubt that this year is the explosive year of humanoid robots. Netizens commented: The advancement of robots has made this year's opening ceremony look like a human, and the degree of freedom is far greater than that of humans. But is this really not a horror movie? At the beginning of the video, Atlas is lying calmly on the ground, seemingly on his back. What follows is jaw-dropping
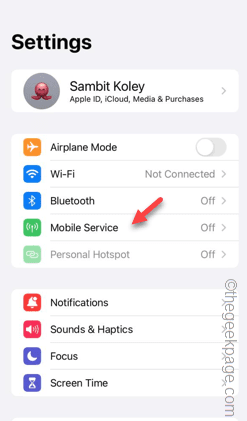
Facing lag, slow mobile data connection on iPhone? Typically, the strength of cellular internet on your phone depends on several factors such as region, cellular network type, roaming type, etc. There are some things you can do to get a faster, more reliable cellular Internet connection. Fix 1 – Force Restart iPhone Sometimes, force restarting your device just resets a lot of things, including the cellular connection. Step 1 – Just press the volume up key once and release. Next, press the Volume Down key and release it again. Step 2 – The next part of the process is to hold the button on the right side. Let the iPhone finish restarting. Enable cellular data and check network speed. Check again Fix 2 – Change data mode While 5G offers better network speeds, it works better when the signal is weaker
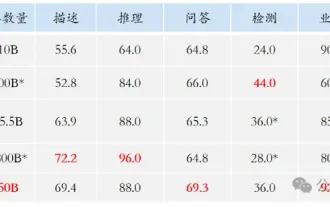
I cry to death. The world is madly building big models. The data on the Internet is not enough. It is not enough at all. The training model looks like "The Hunger Games", and AI researchers around the world are worrying about how to feed these data voracious eaters. This problem is particularly prominent in multi-modal tasks. At a time when nothing could be done, a start-up team from the Department of Renmin University of China used its own new model to become the first in China to make "model-generated data feed itself" a reality. Moreover, it is a two-pronged approach on the understanding side and the generation side. Both sides can generate high-quality, multi-modal new data and provide data feedback to the model itself. What is a model? Awaker 1.0, a large multi-modal model that just appeared on the Zhongguancun Forum. Who is the team? Sophon engine. Founded by Gao Yizhao, a doctoral student at Renmin University’s Hillhouse School of Artificial Intelligence.
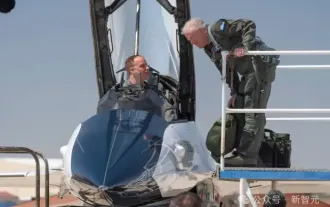
Recently, the military circle has been overwhelmed by the news: US military fighter jets can now complete fully automatic air combat using AI. Yes, just recently, the US military’s AI fighter jet was made public for the first time and the mystery was unveiled. The full name of this fighter is the Variable Stability Simulator Test Aircraft (VISTA). It was personally flown by the Secretary of the US Air Force to simulate a one-on-one air battle. On May 2, U.S. Air Force Secretary Frank Kendall took off in an X-62AVISTA at Edwards Air Force Base. Note that during the one-hour flight, all flight actions were completed autonomously by AI! Kendall said - "For the past few decades, we have been thinking about the unlimited potential of autonomous air-to-air combat, but it has always seemed out of reach." However now,
