


MySQL vs. Oracle: Performance comparison for real-time data analysis and reporting
MySQL and Oracle: Performance comparison for real-time data analysis and reporting
Introduction:
In the modern data-driven decision-making environment, real-time data analysis and reporting have become critical. Database systems are one of the core components of data analysis and reporting. MySQL and Oracle are two widely used relational database management systems. This article will compare their performance in real-time data analysis and reporting, and illustrate it through code examples.
Background:
MySQL is an open source relational database management system widely used in web applications and small businesses. Its main features are ease of use and high performance, but also low cost and easy management. Oracle, on the other hand, is a powerful commercial-grade database management system suitable for large enterprises and complex data processing needs. Oracle has the advantages of high reliability, scalability and security.
Performance comparison:
In terms of real-time data analysis and reporting, performance is one of the important indicators for evaluating database systems. This article will compare the performance of MySQL and Oracle from the following aspects.
- Data import performance:
Data import is the prerequisite for real-time data analysis and reporting. Both MySQL and Oracle have good performance in data import, but Oracle is generally better suited for large-scale data import. The following is a sample code for MySQL and Oracle data import:
MySQL import data code sample:
LOAD DATA LOCAL INFILE 'data.csv' INTO TABLE my_table FIELDS TERMINATED BY ',' LINES TERMINATED BY ' ';
Oracle data import code sample:
LOAD DATA INFILE 'data.csv' INTO TABLE my_table FIELDS TERMINATED BY ',' LINES TERMINATED BY ' ';
- Query performance:
Querying is one of the core operations for real-time data analysis and reporting. Both MySQL and Oracle have powerful query optimizers and indexing mechanisms that can provide fast query performance. However, for complex queries and large data sets, Oracle's performance is usually superior. Below is a sample code for MySQL and Oracle queries:
MySQL query code sample:
SELECT * FROM my_table WHERE category = 'A' AND price > 100;
Oracle query code sample:
SELECT * FROM my_table WHERE category = 'A' AND price > 100;
- Aggregation and grouping Performance:
Aggregating and grouping data are common operations in real-time data analysis and reporting. Both MySQL and Oracle support common aggregation and grouping functions such as SUM, COUNT, and GROUP BY. However, Oracle usually performs better when the data size is larger. The following is a MySQL and Oracle aggregation and grouping code example:
MySQL aggregation and grouping code example:
SELECT category, SUM(price) as total_price, COUNT(*) as total_count FROM my_table GROUP BY category;
Oracle aggregation and grouping code example:
SELECT category, SUM(price) as total_price, COUNT(*) as total_count FROM my_table GROUP BY category;
Conclusion:
MySQL and Oracle are both powerful database management systems suitable for real-time data analysis and reporting. However, they may vary in performance depending on the specific application and needs. For small-scale data and simple queries, MySQL may be more suitable; for large-scale data and complex queries, Oracle may have more advantages. Therefore, when selecting a database system, factors such as data size, query complexity, and acceptable performance should be considered based on the specific situation.
Reference materials:
- MySQL official website: https://www.mysql.com/
- Oracle official website: https://www.oracle. com/
Note: The above code examples are for demonstration purposes only and are not actual executable codes.
Conclusion:
This article compares the performance of MySQL and Oracle in real-time data analysis and reporting, and illustrates it through code examples. I hope readers can understand the differences between the two and choose a database system that suits their needs.
The above is the detailed content of MySQL vs. Oracle: Performance comparison for real-time data analysis and reporting. For more information, please follow other related articles on the PHP Chinese website!

Hot AI Tools

Undresser.AI Undress
AI-powered app for creating realistic nude photos

AI Clothes Remover
Online AI tool for removing clothes from photos.

Undress AI Tool
Undress images for free

Clothoff.io
AI clothes remover

Video Face Swap
Swap faces in any video effortlessly with our completely free AI face swap tool!

Hot Article

Hot Tools

Notepad++7.3.1
Easy-to-use and free code editor

SublimeText3 Chinese version
Chinese version, very easy to use

Zend Studio 13.0.1
Powerful PHP integrated development environment

Dreamweaver CS6
Visual web development tools

SublimeText3 Mac version
God-level code editing software (SublimeText3)

Hot Topics


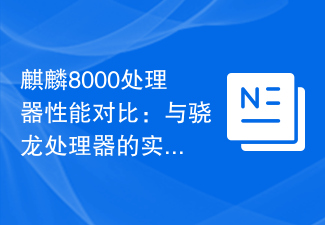
In the current smartphone market, processors can be said to be one of the focuses of competition for major mobile phone manufacturers. As the "brain" of a mobile phone, the performance of the processor directly affects the mobile phone's running speed, multi-tasking capabilities, and gaming experience. At present, the Kirin 8000 processor, as the equipment of Huawei's flagship mobile phone, has attracted much attention. So, what are the advantages and disadvantages of it compared with the Snapdragon processor? First of all, from a performance perspective, the Kirin 8000 processor is manufactured using the latest TSMC 5nm process. It has more advanced process technology and improved performance.
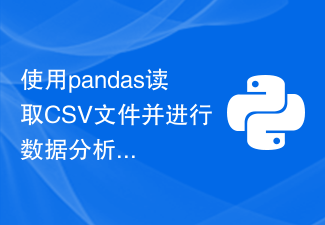
Pandas is a powerful data analysis tool that can easily read and process various types of data files. Among them, CSV files are one of the most common and commonly used data file formats. This article will introduce how to use Pandas to read CSV files and perform data analysis, and provide specific code examples. 1. Import the necessary libraries First, we need to import the Pandas library and other related libraries that may be needed, as shown below: importpandasaspd 2. Read the CSV file using Pan
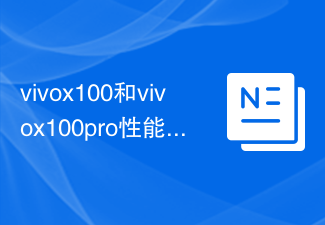
Comparative analysis of performance of vivoX100 and vivoX100Pro As competition in the smartphone market becomes increasingly fierce, major brands continue to launch new products to meet consumer needs. As a well-known mobile phone brand, vivo has launched many well-received products in recent years, among which vivoX100 and vivoX100Pro are highly anticipated new products. What are the differences between the two mobile phones in terms of appearance design, performance configuration, camera functions, etc.? Today we will compare and analyze vivoX100
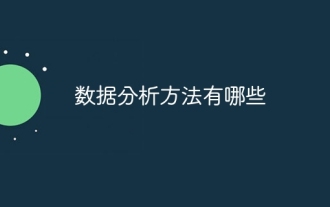
Common data analysis methods: 1. Comparative analysis method; 2. Structural analysis method; 3. Cross analysis method; 4. Trend analysis method; 5. Cause and effect analysis method; 6. Association analysis method; 7. Cluster analysis method; 8 , Principal component analysis method; 9. Scatter analysis method; 10. Matrix analysis method. Detailed introduction: 1. Comparative analysis method: Comparative analysis of two or more data to find the differences and patterns; 2. Structural analysis method: A method of comparative analysis between each part of the whole and the whole. ; 3. Cross analysis method, etc.
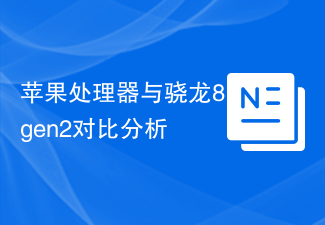
Comparative analysis of Apple processor and Snapdragon 8gen2 With the continuous development of mobile smart devices, the processor, as the core component of device performance, has always attracted much attention. Apple and Qualcomm have always been leaders in the field of mobile processors, launching their own processor products, namely Apple processors and Snapdragon processors. Among them, Apple processors are known for their powerful performance and excellent power consumption control, while Qualcomm Snapdragon processors have won the favor of users for their excellent communication technology and versatility. This article will review Apple processors and Snapdragon 8g
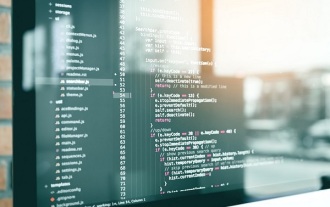
Following the last inventory of "11 Basic Charts Data Scientists Use 95% of the Time", today we will bring you 11 basic distributions that data scientists use 95% of the time. Mastering these distributions helps us understand the nature of the data more deeply and make more accurate inferences and predictions during data analysis and decision-making. 1. Normal Distribution Normal Distribution, also known as Gaussian Distribution, is a continuous probability distribution. It has a symmetrical bell-shaped curve with the mean (μ) as the center and the standard deviation (σ) as the width. The normal distribution has important application value in many fields such as statistics, probability theory, and engineering.
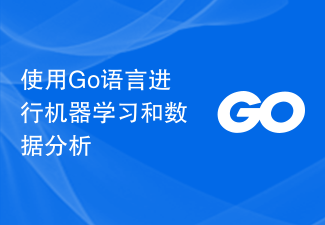
In today's intelligent society, machine learning and data analysis are indispensable tools that can help people better understand and utilize large amounts of data. In these fields, Go language has also become a programming language that has attracted much attention. Its speed and efficiency make it the choice of many programmers. This article introduces how to use Go language for machine learning and data analysis. 1. The ecosystem of machine learning Go language is not as rich as Python and R. However, as more and more people start to use it, some machine learning libraries and frameworks
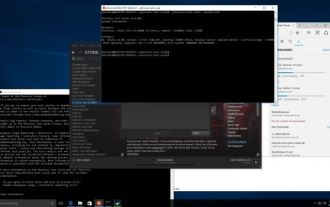
At the beginning of this year, when Microsoft and Canonical released Windows 10 Bash and Ubuntu user space, I tried to do some preliminary performance tests on Ubuntu on Windows 10 compared to native Ubuntu. This time I published more about the benchmark comparison between native pure Ubuntu and Windows 10. The Linux subsystem test for Windows completed all tests and was released with the Windows 10 Anniversary Update. The default Ubuntu user space is still Ubuntu14.04, but it can be upgraded to 16.04. So the test is first tested on 14.04, and after completion, the system will be upgraded to 16.04
