


The visual talent of large language models: GPT can also solve visual tasks through contextual learning
Currently, large language models (LLM) have set off a wave of changes in the field of natural language processing (NLP). We see that LLM has strong emergence capabilities and performs well on complex language understanding tasks, generation tasks and even reasoning tasks. This inspires people to further explore the potential of LLM in another subfield of machine learning - computer vision (CV).
One of the remarkable talents of LLMs is their ability to learn in context. Contextual learning does not update any parameters of the LLM, but it shows amazing results in various NLP tasks. So, can GPT solve visual tasks through contextual learning?
Recently, a paper jointly published by researchers from Google and Carnegie Mellon University (CMU) shows that as long as we can convert images (or other non-verbal modalities) Translated into a language that LLM can understand, this seems feasible.
Picture
Paper address: https://arxiv.org/abs/2306.17842
This paper reveals the ability of PaLM or GPT in solving visual tasks through contextual learning, and proposes a new method SPAE (Semantic Pyramid AutoEncoder). This new approach enables LLM to perform image generation tasks without any parameter updates. This is also the first successful method to use contextual learning to enable LLM to generate image content.
Let’s first take a look at the experimental effect of LLM on generating image content through context learning.
For example, by providing 50 images of handwriting in a given context, the paper asks PaLM 2 to answer a complex query that requires generating a digital image as output:
Pictures
can also generate realistic realistic images with image context input:
Picture
In addition to generating images, through context learning, PaLM 2 can also perform image description:
# #There are also visual Q&A for image-related questions:
Pictures
You can even generate videos with denoising:
Picture
Method Overview
In fact, convert the image into a language that LLM can understand , is a problem that has been studied in the Visual Transformer (ViT) paper. In this paper from Google and CMU, they take it to the next level — using actual words to represent images.
This approach is like building a tower filled with text, capturing the semantics and detail of the image. This text-filled representation allows image descriptions to be easily generated and allows LLMs to answer image-related questions and even reconstruct image pixels.
Specifically, this research proposes to use a trained encoder and CLIP model to convert the image into a token space; and then use LLM to generate a suitable lexical tokens; finally using a trained decoder to convert these tokens back to pixel space. This ingenious process converts images into a language that LLM can understand, allowing us to exploit the generative power of LLM in vision tasks.
Experiments and results
This study experimentally compared SPAE with SOTA methods Frozen and LQAE, and the results are shown in Table 1 below. SPAEGPT outperforms LQAE on all tasks while using only 2% of tokens.
Picture
Overall, testing on the mini-ImageNet benchmark shows that the SPAE method outperforms the previous SOTA The method improves performance by 25%.
Picture
In order to verify the effectiveness of the SPAE design method, this study conducted an ablation experiment. The experimental results are as follows Table 4 and Shown in Figure 10:
Picture
Picture
Feeling Interested readers can read the original text of the paper to learn more about the research content.
The above is the detailed content of The visual talent of large language models: GPT can also solve visual tasks through contextual learning. For more information, please follow other related articles on the PHP Chinese website!

Hot AI Tools

Undresser.AI Undress
AI-powered app for creating realistic nude photos

AI Clothes Remover
Online AI tool for removing clothes from photos.

Undress AI Tool
Undress images for free

Clothoff.io
AI clothes remover

AI Hentai Generator
Generate AI Hentai for free.

Hot Article

Hot Tools

Notepad++7.3.1
Easy-to-use and free code editor

SublimeText3 Chinese version
Chinese version, very easy to use

Zend Studio 13.0.1
Powerful PHP integrated development environment

Dreamweaver CS6
Visual web development tools

SublimeText3 Mac version
God-level code editing software (SublimeText3)

Hot Topics
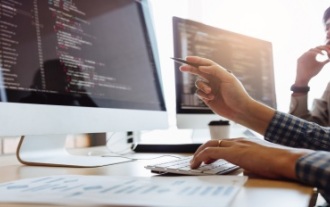
Image annotation is the process of associating labels or descriptive information with images to give deeper meaning and explanation to the image content. This process is critical to machine learning, which helps train vision models to more accurately identify individual elements in images. By adding annotations to images, the computer can understand the semantics and context behind the images, thereby improving the ability to understand and analyze the image content. Image annotation has a wide range of applications, covering many fields, such as computer vision, natural language processing, and graph vision models. It has a wide range of applications, such as assisting vehicles in identifying obstacles on the road, and helping in the detection and diagnosis of diseases through medical image recognition. . This article mainly recommends some better open source and free image annotation tools. 1.Makesens
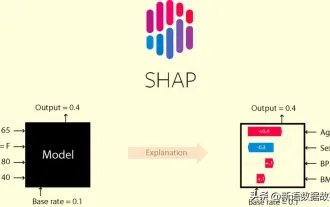
In the fields of machine learning and data science, model interpretability has always been a focus of researchers and practitioners. With the widespread application of complex models such as deep learning and ensemble methods, understanding the model's decision-making process has become particularly important. Explainable AI|XAI helps build trust and confidence in machine learning models by increasing the transparency of the model. Improving model transparency can be achieved through methods such as the widespread use of multiple complex models, as well as the decision-making processes used to explain the models. These methods include feature importance analysis, model prediction interval estimation, local interpretability algorithms, etc. Feature importance analysis can explain the decision-making process of a model by evaluating the degree of influence of the model on the input features. Model prediction interval estimate
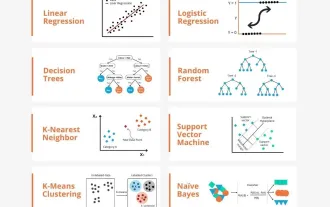
In layman’s terms, a machine learning model is a mathematical function that maps input data to a predicted output. More specifically, a machine learning model is a mathematical function that adjusts model parameters by learning from training data to minimize the error between the predicted output and the true label. There are many models in machine learning, such as logistic regression models, decision tree models, support vector machine models, etc. Each model has its applicable data types and problem types. At the same time, there are many commonalities between different models, or there is a hidden path for model evolution. Taking the connectionist perceptron as an example, by increasing the number of hidden layers of the perceptron, we can transform it into a deep neural network. If a kernel function is added to the perceptron, it can be converted into an SVM. this one
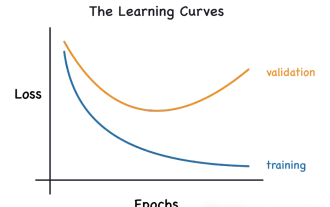
This article will introduce how to effectively identify overfitting and underfitting in machine learning models through learning curves. Underfitting and overfitting 1. Overfitting If a model is overtrained on the data so that it learns noise from it, then the model is said to be overfitting. An overfitted model learns every example so perfectly that it will misclassify an unseen/new example. For an overfitted model, we will get a perfect/near-perfect training set score and a terrible validation set/test score. Slightly modified: "Cause of overfitting: Use a complex model to solve a simple problem and extract noise from the data. Because a small data set as a training set may not represent the correct representation of all data." 2. Underfitting Heru
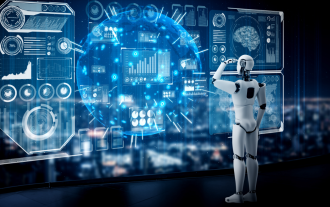
In the 1950s, artificial intelligence (AI) was born. That's when researchers discovered that machines could perform human-like tasks, such as thinking. Later, in the 1960s, the U.S. Department of Defense funded artificial intelligence and established laboratories for further development. Researchers are finding applications for artificial intelligence in many areas, such as space exploration and survival in extreme environments. Space exploration is the study of the universe, which covers the entire universe beyond the earth. Space is classified as an extreme environment because its conditions are different from those on Earth. To survive in space, many factors must be considered and precautions must be taken. Scientists and researchers believe that exploring space and understanding the current state of everything can help understand how the universe works and prepare for potential environmental crises
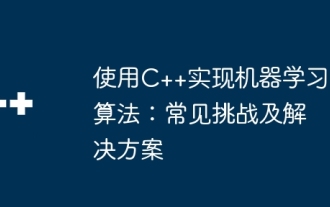
Common challenges faced by machine learning algorithms in C++ include memory management, multi-threading, performance optimization, and maintainability. Solutions include using smart pointers, modern threading libraries, SIMD instructions and third-party libraries, as well as following coding style guidelines and using automation tools. Practical cases show how to use the Eigen library to implement linear regression algorithms, effectively manage memory and use high-performance matrix operations.
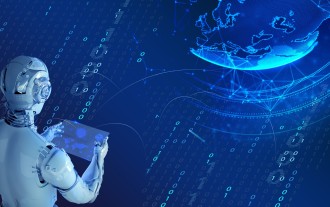
Translator | Reviewed by Li Rui | Chonglou Artificial intelligence (AI) and machine learning (ML) models are becoming increasingly complex today, and the output produced by these models is a black box – unable to be explained to stakeholders. Explainable AI (XAI) aims to solve this problem by enabling stakeholders to understand how these models work, ensuring they understand how these models actually make decisions, and ensuring transparency in AI systems, Trust and accountability to address this issue. This article explores various explainable artificial intelligence (XAI) techniques to illustrate their underlying principles. Several reasons why explainable AI is crucial Trust and transparency: For AI systems to be widely accepted and trusted, users need to understand how decisions are made
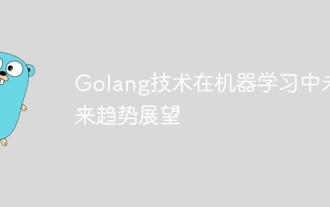
The application potential of Go language in the field of machine learning is huge. Its advantages are: Concurrency: It supports parallel programming and is suitable for computationally intensive operations in machine learning tasks. Efficiency: The garbage collector and language features ensure that the code is efficient, even when processing large data sets. Ease of use: The syntax is concise, making it easy to learn and write machine learning applications.
