


Chen Danqi's ACL academic report is here! Detailed explanation of the 7 major directions and 3 major challenges of the large model 'plug-in' database, 3 hours full of useful information
Tsinghua Yao Class AlumniChen Danqi gave a latest speech at ACL 2023!
The topic is still a very hot research direction recently-
(big)Language models like GPT-3 and PaLM, whether It is necessary to rely on retrieval to make up for its own shortcomings, so as to better implement the application.
In this speech, she and three other speakers jointly introduced several major research directions on this topic, including training methods, applications and challenges.
Picture
The response from the audience during the speech was also very enthusiastic. Many netizens raised their questions seriously, and several speakers tried their best to answer their questions. .
Picture
As for the specific effect of this speech? Some netizens directly said "recommend" to the comment area.
Picture
So, what exactly did they talk about in this 3-hour speech? What other places are worth listening to?
Why do large models need "plug-in" databases?
The core theme of this speech is "Retrieval-based Language Model", which contains two elements: Retrieval and Language Model.
From the definition, it refers to "plugging in" a data retrieval database to the language model, and using this database when performing inference (etc. operations) Perform a search and finally output based on the search results.
This type of plug-in data repository is also called a semi-parametric model or a non-parametric model.
Picture
The reason why we need to study this direction is because of (big) language models such as GPT-3 and PaLM , while showing good results, some troublesome "bugs" also appeared. There are three main problems:
1. The number of parameters is too large. If it is repeated based on new data, Training, the computational cost is too high;
2, poor memory (faced with long text, memorize the following and forget the above), over time it will cause hallucinations, and it is easy to Data leakage;
3, With the current amount of parameters, it is impossible to remember all the knowledge.
In this case, the external retrieval corpus was proposed, which is to "plug-in" a database for the large language model, so that it can answer questions by searching for information at any time, and because this database can be updated at any time, it can also Don't worry about the cost of retraining.
After introducing the definition and background, it is the specific architecture, training, multi-modality, application and challenges of this research direction.
In the architecture, it mainly introduces the content, retrieval method and retrieval "timing" based on the language model retrieval.
Specifically, this type of model mainly retrieves tokens, text blocks and entity words (entity mentions) . The methods and timing of using retrieval are also very diverse, and it is a very flexible type. model architecture.
Picture
In terms of training method, it focuses on independent training(independent training, language model and retrieval Model separate training) , continuous learning (sequential training) , multi-task learning (joint training) and other methods.
Picture
As for application, this type of model involves more, and can be used not only for code generation, It can be used for tasks such as classification and knowledge-intensive NLP, and can be used through methods such as fine-tuning, reinforcement learning, and retrieval-based prompt words.
Application scenarios are also very flexible, including long-tail scenarios, scenarios that require knowledge updates, and scenarios involving privacy and security. This type of model has a place to be used.
Of course, it’s not just about text. This type of model also has the potential for multimodal extension, which can be used for tasks other than text.
Picture
It sounds like this type of model has many advantages, but there are also some challenges based on the retrieval-based language model.
In his final "ending" speech, Chen Danqi highlighted several major problems that need to be solved in this research direction.
First, small language model (continuously expanding) Does a large database essentially mean that the number of parameters of the language model is still very large? How to solve this problem?
For example, although the parameter amount of this type of model can be very small, only 7 billion parameters, the plug-in database can reach 2T...
Picture
Second, the efficiency of similarity search. How to design algorithms to maximize search efficiency is currently a very active research direction.
Picture
Third, complete complex language tasks. Including open-ended text generation tasks and complex text reasoning tasks, how to use retrieval-based language models to complete these tasks is also a direction that requires continued exploration.
Picture
Of course, Chen Danqi also mentioned that these topics are not only challenges, but also research opportunities. Friends who are still looking for thesis topics, you can consider whether to add them to the research list~
It is worth mentioning that this speech is not a topic "out of thin air", 4 speakers I thoughtfully posted a link to the paper reference for the speech on the official website.
From model architecture, training methods, applications, multi-modality to challenges, if you are interested in any part of these topics, you can go to the official website to find the corresponding classic papers:
Picture
Answer the audience’s confusion on the spot
It was such a informative speech, and the four keynote speakers were not without background. They also patiently spoke during the speech. Questions raised by the audience were answered.
Let’s first talk about who the speakers are at Kangkang.
The first is Chen Danqi, assistant professor of computer science at Princeton University who led this speech.
Picture
CDQ divide and conquer algorithm is named after her. In 2008, she won an IOI gold medal on behalf of the Chinese team.
And her 156-page doctoral thesis "Neural Reading Comprehension and Beyond" once became very popular. Not only did it win the Stanford Best Doctoral Thesis Award that year, it also became a recent Stanford University award. One of the most popular graduation thesis of the decade. Now, in addition to being an assistant professor of computer science at Princeton University, Chen Danqi is also the co-leader of the school’s NLP team and a member of the AIML team. Her research interests mainly focus on natural language processing and machine learning, and she is interested in simple and reliable methods that are feasible, scalable and generalizable in practical problems. Also from Princeton University, there is also Chen Danqi’s apprenticeZhong Zexuan(Zexuan Zhong).
Picture
His latest research focuses on extracting structured information from unstructured text, extracting factual information from pre-trained language models, analyzing the generalization ability of dense retrieval models, and developing language models suitable for retrieval-based training techniques.
In addition, the keynote speakers include Akari Asai and Sewon Min from Washington University.
Picture
Akari Asai is a fourth-year doctoral student at the University of Washington, majoring in natural language processing. He graduated from the University of Tokyo, Japan.
She is primarily interested in developing reliable and adaptable natural language processing systems to improve information acquisition.
Recently, her research focuses on general knowledge retrieval systems, efficient adaptive NLP models and other fields.
Picture
Sewon Min is a doctoral candidate in the Natural Language Processing Group at the University of Washington. During his doctoral studies, he worked at Meta AI He worked part-time as a researcher for four years and graduated from Seoul National University with a bachelor's degree.
Recently she focuses on language modeling, retrieval and the intersection of the two.
During the speech, the audience also enthusiastically raised many questions, such as why perplexity (perplexity) is used as the main indicator of the speech.
Picture
The speaker gave a detailed answer:
When comparing parameterized language models, the degree of confusion (PPL) is often used. But whether improvements in perplexity can be translated into downstream applications remains a research question.
Research has shown that perplexity has a good correlation with downstream tasks (especially generation tasks) , and perplexity usually provides very stable results, which can be Evaluation on large-scale evaluation data (Compared to downstream tasks, evaluation data is unlabeled, and downstream tasks may be affected by the sensitivity of cues and the lack of large-scale labeled data, resulting in unstable results) .
Picture
Some netizens raised this question:
About "The training cost of language model is high, and Introducing retrieval may solve this problem", did you just replace time complexity with space complexity(data storage)?
The answer given by the speaker is Aunt Jiang’s:
The focus of our discussion is how to reduce the language model to a smaller size. Small, thus reducing time and space requirements. However, data storage also actually adds additional overhead, which needs to be carefully weighed and studied, and we believe this is a current challenge.
Compared with training a language model with more than 10 billion parameters, I think the most important thing at present is to reduce the training cost.
Pictures
If you want to find the PPT of this speech, or the specific playback, you can go to the official website to have a look~
Official website: https://acl2023-retrieval-lm.github.io/
The above is the detailed content of Chen Danqi's ACL academic report is here! Detailed explanation of the 7 major directions and 3 major challenges of the large model 'plug-in' database, 3 hours full of useful information. For more information, please follow other related articles on the PHP Chinese website!

Hot AI Tools

Undresser.AI Undress
AI-powered app for creating realistic nude photos

AI Clothes Remover
Online AI tool for removing clothes from photos.

Undress AI Tool
Undress images for free

Clothoff.io
AI clothes remover

AI Hentai Generator
Generate AI Hentai for free.

Hot Article

Hot Tools

Notepad++7.3.1
Easy-to-use and free code editor

SublimeText3 Chinese version
Chinese version, very easy to use

Zend Studio 13.0.1
Powerful PHP integrated development environment

Dreamweaver CS6
Visual web development tools

SublimeText3 Mac version
God-level code editing software (SublimeText3)

Hot Topics


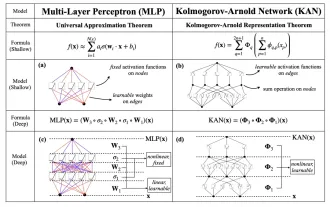
Earlier this month, researchers from MIT and other institutions proposed a very promising alternative to MLP - KAN. KAN outperforms MLP in terms of accuracy and interpretability. And it can outperform MLP running with a larger number of parameters with a very small number of parameters. For example, the authors stated that they used KAN to reproduce DeepMind's results with a smaller network and a higher degree of automation. Specifically, DeepMind's MLP has about 300,000 parameters, while KAN only has about 200 parameters. KAN has a strong mathematical foundation like MLP. MLP is based on the universal approximation theorem, while KAN is based on the Kolmogorov-Arnold representation theorem. As shown in the figure below, KAN has
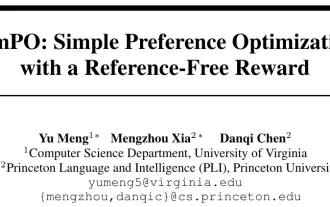
In order to align large language models (LLMs) with human values and intentions, it is critical to learn human feedback to ensure that they are useful, honest, and harmless. In terms of aligning LLM, an effective method is reinforcement learning based on human feedback (RLHF). Although the results of the RLHF method are excellent, there are some optimization challenges involved. This involves training a reward model and then optimizing a policy model to maximize that reward. Recently, some researchers have explored simpler offline algorithms, one of which is direct preference optimization (DPO). DPO learns the policy model directly based on preference data by parameterizing the reward function in RLHF, thus eliminating the need for an explicit reward model. This method is simple and stable
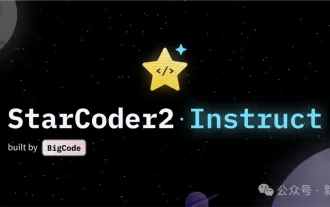
At the forefront of software technology, UIUC Zhang Lingming's group, together with researchers from the BigCode organization, recently announced the StarCoder2-15B-Instruct large code model. This innovative achievement achieved a significant breakthrough in code generation tasks, successfully surpassing CodeLlama-70B-Instruct and reaching the top of the code generation performance list. The unique feature of StarCoder2-15B-Instruct is its pure self-alignment strategy. The entire training process is open, transparent, and completely autonomous and controllable. The model generates thousands of instructions via StarCoder2-15B in response to fine-tuning the StarCoder-15B base model without relying on expensive manual annotation.
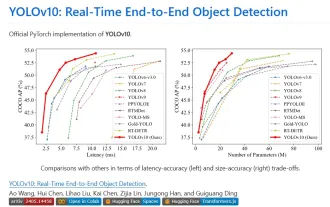
1. Introduction Over the past few years, YOLOs have become the dominant paradigm in the field of real-time object detection due to its effective balance between computational cost and detection performance. Researchers have explored YOLO's architectural design, optimization goals, data expansion strategies, etc., and have made significant progress. At the same time, relying on non-maximum suppression (NMS) for post-processing hinders end-to-end deployment of YOLO and adversely affects inference latency. In YOLOs, the design of various components lacks comprehensive and thorough inspection, resulting in significant computational redundancy and limiting the capabilities of the model. It offers suboptimal efficiency, and relatively large potential for performance improvement. In this work, the goal is to further improve the performance efficiency boundary of YOLO from both post-processing and model architecture. to this end
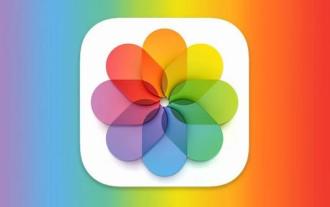
Apple's latest releases of iOS18, iPadOS18 and macOS Sequoia systems have added an important feature to the Photos application, designed to help users easily recover photos and videos lost or damaged due to various reasons. The new feature introduces an album called "Recovered" in the Tools section of the Photos app that will automatically appear when a user has pictures or videos on their device that are not part of their photo library. The emergence of the "Recovered" album provides a solution for photos and videos lost due to database corruption, the camera application not saving to the photo library correctly, or a third-party application managing the photo library. Users only need a few simple steps
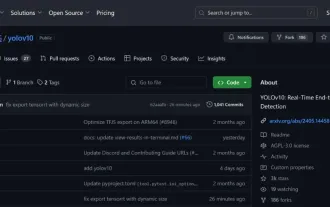
The benchmark YOLO series of target detection systems has once again received a major upgrade. Since the release of YOLOv9 in February this year, the baton of the YOLO (YouOnlyLookOnce) series has been passed to the hands of researchers at Tsinghua University. Last weekend, the news of the launch of YOLOv10 attracted the attention of the AI community. It is considered a breakthrough framework in the field of computer vision and is known for its real-time end-to-end object detection capabilities, continuing the legacy of the YOLO series by providing a powerful solution that combines efficiency and accuracy. Paper address: https://arxiv.org/pdf/2405.14458 Project address: https://github.com/THU-MIG/yo
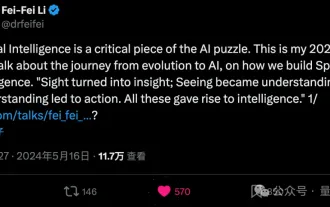
After Stanford's Feifei Li started his business, he unveiled the new concept "spatial intelligence" for the first time. This is not only her entrepreneurial direction, but also the "North Star" that guides her. She considers it "the key puzzle piece to solve the artificial intelligence problem." Visualization leads to insight; seeing leads to understanding; understanding leads to action. Based on Li Feifei's 15-minute TED talk, which is fully open to the public, it starts from the origin of life evolution hundreds of millions of years ago, to how humans are not satisfied with what nature has given them and develops artificial intelligence, to how to build spatial intelligence in the next step. Nine years ago, Li Feifei introduced the newly born ImageNet to the world on the same stage - one of the starting points for this round of deep learning explosion. She herself also encouraged netizens: If you watch both videos, you will be able to understand the computer vision of the past 10 years.
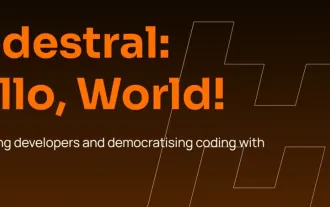
French AI unicorn MistralAI, which is targeting OpenAI, has made a new move: Codestral, the first large code model, was born. As an open generative AI model designed specifically for code generation tasks, Codestral helps developers write and interact with code by sharing instructions and completion API endpoints. Codestral's proficiency in coding and English allows software developers to design advanced AI applications. The parameter size of Codestral is 22B, it complies with the new MistralAINon-ProductionLicense, and can be used for research and testing purposes, but commercial use is prohibited. Currently, the model is available for download on HuggingFace. download link
