


How to use the keras module for deep learning in Python 3.x
How to use the Keras module for deep learning in Python 3.x
Keras is a high-level neural network library for building and training deep learning models. It is based on Python and supports backends such as TensorFlow, Theano, and MxNet. Keras provides a simple and easy-to-use API, allowing us to quickly build various types of deep learning models, such as Multilayer Perceptron, Convolutional Neural Network and Recurrent Neural Network )wait.
This article will introduce how to use the Keras module for deep learning in the Python 3.x environment. We will first install Keras and its dependent libraries, and then learn how to build and train a simple neural network model through a simple example code.
1. Install Keras
Before we begin, we need to install Keras into our Python environment. Keras can be installed using pip through the following command:
pip install keras
2. Build a simple neural network model
Next, we will use Keras to build a simple neural network model to realize handwritten digit recognition Task. First, we need to import the necessary libraries:
import numpy as np from keras.models import Sequential from keras.layers import Dense from keras.utils import np_utils
Then, we need to load the MNIST data set, which contains 60,000 training samples and 10,000 test samples. Each sample is a 28x28 grayscale image, corresponding to a A number between [0, 9]. The following code can be used to load the dataset:
from keras.datasets import mnist (X_train, Y_train), (X_test, Y_test) = mnist.load_data()
Next, we need to preprocess the data. Since the original image data is a 28x28 grayscale image, we need to flatten it into a 784-dimensional vector and normalize the input data to scale the pixel value from the range of [0, 255] to [0, 1 ] Within the range:
X_train = X_train.reshape(X_train.shape[0], 784).astype('float32') / 255 X_test = X_test.reshape(X_test.shape[0], 784).astype('float32') / 255
In order to be able to train the model, we also need to one-hot encode the label. Keras provides the np_utils.to_categorical() function to help us achieve this step:
Y_train = np_utils.to_categorical(Y_train, 10) Y_test = np_utils.to_categorical(Y_test, 10)
Now, we can build a simple multilayer perceptron (Multilayer Perceptron) model. This model contains an input layer, two hidden layers and an output layer. You can use the Sequential() function to create a sequence model and the Dense() function to add layers:
model = Sequential() model.add(Dense(units=512, input_dim=784, activation='relu')) model.add(Dense(units=512, activation='relu')) model.add(Dense(units=10, activation='softmax'))
After the model is built, we need to compile the model. You can use the compile() function to configure the model training process. Here, we can specify the loss function, optimizer and evaluation index:
model.compile(loss='categorical_crossentropy', optimizer='adam', metrics=['accuracy'])
3. Training model and prediction
After the model is compiled, we can use the fit() function to train the model. You can specify the training data, the number of training rounds, and the number of samples in each batch:
model.fit(X_train, Y_train, epochs=10, batch_size=128)
After the model training is completed, we can use the evaluate() function to evaluate the performance of the model on the test set:
loss, accuracy = model.evaluate(X_test, Y_test) print('Test loss:', loss) print('Test accuracy:', accuracy)
Finally, we can use the predict_classes() function to predict the category of new samples:
predictions = model.predict_classes(X_test)
In this way, we have completed the construction and training process of a simple neural network model.
Summary:
This article introduces how to use the Keras module for deep learning in Python 3.x. We first installed Keras and its dependent libraries, and then learned how to build and train a simple neural network model through sample code. This is just an introduction to deep learning. Keras also provides more functions and models to meet different needs. I hope that readers can have a preliminary understanding of Keras and deep learning through the introduction of this article, and be able to use it in practical applications.
The above is the detailed content of How to use the keras module for deep learning in Python 3.x. For more information, please follow other related articles on the PHP Chinese website!

Hot AI Tools

Undresser.AI Undress
AI-powered app for creating realistic nude photos

AI Clothes Remover
Online AI tool for removing clothes from photos.

Undress AI Tool
Undress images for free

Clothoff.io
AI clothes remover

AI Hentai Generator
Generate AI Hentai for free.

Hot Article

Hot Tools

Notepad++7.3.1
Easy-to-use and free code editor

SublimeText3 Chinese version
Chinese version, very easy to use

Zend Studio 13.0.1
Powerful PHP integrated development environment

Dreamweaver CS6
Visual web development tools

SublimeText3 Mac version
God-level code editing software (SublimeText3)

Hot Topics


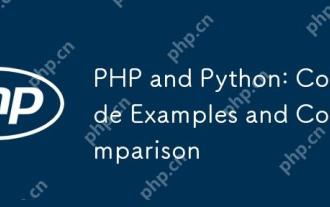
PHP and Python have their own advantages and disadvantages, and the choice depends on project needs and personal preferences. 1.PHP is suitable for rapid development and maintenance of large-scale web applications. 2. Python dominates the field of data science and machine learning.
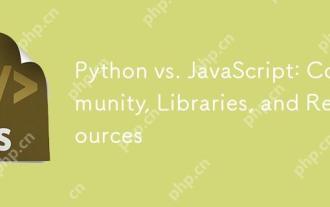
Python and JavaScript have their own advantages and disadvantages in terms of community, libraries and resources. 1) The Python community is friendly and suitable for beginners, but the front-end development resources are not as rich as JavaScript. 2) Python is powerful in data science and machine learning libraries, while JavaScript is better in front-end development libraries and frameworks. 3) Both have rich learning resources, but Python is suitable for starting with official documents, while JavaScript is better with MDNWebDocs. The choice should be based on project needs and personal interests.
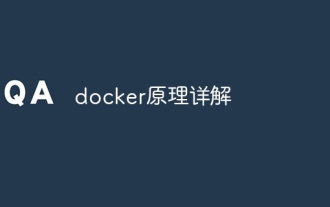
Docker uses Linux kernel features to provide an efficient and isolated application running environment. Its working principle is as follows: 1. The mirror is used as a read-only template, which contains everything you need to run the application; 2. The Union File System (UnionFS) stacks multiple file systems, only storing the differences, saving space and speeding up; 3. The daemon manages the mirrors and containers, and the client uses them for interaction; 4. Namespaces and cgroups implement container isolation and resource limitations; 5. Multiple network modes support container interconnection. Only by understanding these core concepts can you better utilize Docker.
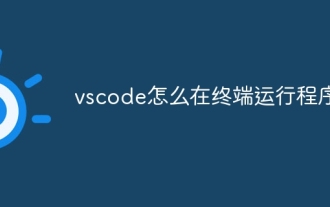
In VS Code, you can run the program in the terminal through the following steps: Prepare the code and open the integrated terminal to ensure that the code directory is consistent with the terminal working directory. Select the run command according to the programming language (such as Python's python your_file_name.py) to check whether it runs successfully and resolve errors. Use the debugger to improve debugging efficiency.
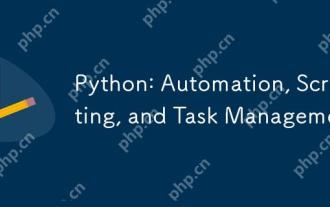
Python excels in automation, scripting, and task management. 1) Automation: File backup is realized through standard libraries such as os and shutil. 2) Script writing: Use the psutil library to monitor system resources. 3) Task management: Use the schedule library to schedule tasks. Python's ease of use and rich library support makes it the preferred tool in these areas.
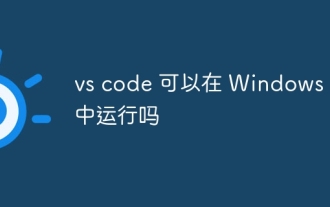
VS Code can run on Windows 8, but the experience may not be great. First make sure the system has been updated to the latest patch, then download the VS Code installation package that matches the system architecture and install it as prompted. After installation, be aware that some extensions may be incompatible with Windows 8 and need to look for alternative extensions or use newer Windows systems in a virtual machine. Install the necessary extensions to check whether they work properly. Although VS Code is feasible on Windows 8, it is recommended to upgrade to a newer Windows system for a better development experience and security.
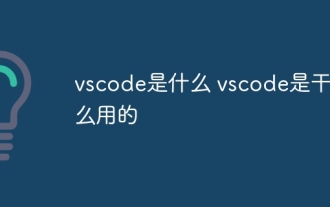
VS Code is the full name Visual Studio Code, which is a free and open source cross-platform code editor and development environment developed by Microsoft. It supports a wide range of programming languages and provides syntax highlighting, code automatic completion, code snippets and smart prompts to improve development efficiency. Through a rich extension ecosystem, users can add extensions to specific needs and languages, such as debuggers, code formatting tools, and Git integrations. VS Code also includes an intuitive debugger that helps quickly find and resolve bugs in your code.
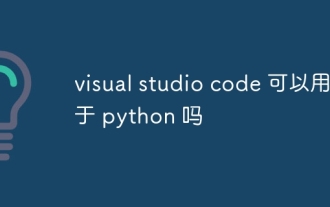
VS Code can be used to write Python and provides many features that make it an ideal tool for developing Python applications. It allows users to: install Python extensions to get functions such as code completion, syntax highlighting, and debugging. Use the debugger to track code step by step, find and fix errors. Integrate Git for version control. Use code formatting tools to maintain code consistency. Use the Linting tool to spot potential problems ahead of time.
