


How to use the numpy module for numerical calculations in Python 3.x
How to use the numpy module for numerical calculations in Python 3.x
Introduction:
In the field of scientific computing in Python, numpy is a very important module. It provides high-performance multidimensional array objects and a series of functions for processing these arrays. By using numpy, we can simplify numerical calculation operations and achieve higher computing efficiency.
This article will introduce how to use the numpy module for numerical calculations in Python 3.x and provide corresponding code examples.
1. Install the numpy module:
Before we start, we need to install the numpy module first. You can use the pip command to install, just execute the following command:
pip install numpy
Of course, you can also use other suitable methods to install.
2. Import the numpy module:
Before starting to use numpy, we need to import the numpy module. You can use the following code to import the numpy module into a Python program:
import numpy as np
When importing, we usually use the alias np
to represent the numpy module. This is to facilitate the use of functions in the numpy module .
3. Create a numpy array:
The first step in using numpy for numerical calculations is to create a numpy array. Numpy arrays are multi-dimensional array objects that can hold data of the same type.
The following are three common ways to create numpy arrays:
- Create from a regular Python list or tuple using the
np.array()
function:
import numpy as np arr1 = np.array([1, 2, 3, 4, 5]) print(arr1)
Output:
[1 2 3 4 5]
- Use the
np.zeros()
function to create an array of all 0s:
import numpy as np arr2 = np.zeros((3, 4)) print(arr2)
Output :
[[0. 0. 0. 0.] [0. 0. 0. 0.] [0. 0. 0. 0.]]
- Use the
np.ones()
function to create an array of all 1s:
import numpy as np arr3 = np.ones((2, 3)) print(arr3)
Output:
[[1. 1. 1.] [1. 1. 1.]]
IV. Properties and operations of numpy arrays:
Numpy array is not just an ordinary array object, it also has some special properties and operations. Here are examples of some common numpy array properties and operations:
- Shape of the array
shape
:
import numpy as np arr = np.array([[1, 2, 3], [4, 5, 6]]) print(arr.shape)
Output:
(2, 3)
- Dimensions of the array
ndim
:
import numpy as np arr = np.array([1, 2, 3, 4]) print(arr.ndim)
Output:
1
- Type of the array
dtype
:
import numpy as np arr = np.array([1, 2, 3, 4]) print(arr.dtype)
Output:
int64
- Number of elements in the array
size
:
import numpy as np arr = np.array([1, 2, 3, 4]) print(arr.size)
Output:
4
5. Numerical calculations of numpy arrays:
numpy arrays provide a wealth of numerical calculation functions that can be used to perform various common mathematical operations. The following are examples of some common numpy numerical calculation functions:
- Addition of arrays
np.add()
:
import numpy as np arr1 = np.array([1, 2, 3]) arr2 = np.array([4, 5, 6]) result = np.add(arr1, arr2) print(result)
Output:
[5 7 9]
- Subtraction of arrays
np.subtract()
:
import numpy as np arr1 = np.array([4, 5, 6]) arr2 = np.array([1, 2, 3]) result = np.subtract(arr1, arr2) print(result)
Output:
[3 3 3]
- Multiplication of arrays
np.multiply()
:
import numpy as np arr1 = np.array([1, 2, 3]) arr2 = np.array([4, 5, 6]) result = np.multiply(arr1, arr2) print(result)
Output:
[4 10 18]
- Division of arrays
np.divide()
:
import numpy as np arr1 = np.array([4, 5, 6]) arr2 = np.array([2, 2, 2]) result = np.divide(arr1, arr2) print(result)
Output:
[2. 2.5 3. ]
The above are just a few examples of numpy numerical calculation functions. Numpy also provides many other commonly used numerical calculation functions, which can be used according to specific needs.
Conclusion:
By using the numpy module, we can easily perform numerical calculations and obtain higher computing efficiency. In this article, we introduce how to install the numpy module, import the numpy module, create numpy arrays, and perform numerical calculations, and provide corresponding code examples.
By learning and mastering the numpy module, we can carry out scientific computing work in Python more efficiently, and at the same time, we have laid a solid foundation for further in-depth study of machine learning, data analysis and other fields.
The above is the detailed content of How to use the numpy module for numerical calculations in Python 3.x. For more information, please follow other related articles on the PHP Chinese website!

Hot AI Tools

Undresser.AI Undress
AI-powered app for creating realistic nude photos

AI Clothes Remover
Online AI tool for removing clothes from photos.

Undress AI Tool
Undress images for free

Clothoff.io
AI clothes remover

AI Hentai Generator
Generate AI Hentai for free.

Hot Article

Hot Tools

Notepad++7.3.1
Easy-to-use and free code editor

SublimeText3 Chinese version
Chinese version, very easy to use

Zend Studio 13.0.1
Powerful PHP integrated development environment

Dreamweaver CS6
Visual web development tools

SublimeText3 Mac version
God-level code editing software (SublimeText3)

Hot Topics
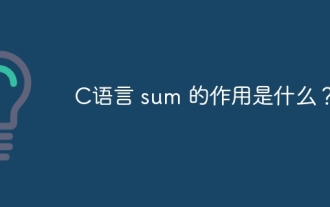
There is no built-in sum function in C language, so it needs to be written by yourself. Sum can be achieved by traversing the array and accumulating elements: Loop version: Sum is calculated using for loop and array length. Pointer version: Use pointers to point to array elements, and efficient summing is achieved through self-increment pointers. Dynamically allocate array version: Dynamically allocate arrays and manage memory yourself, ensuring that allocated memory is freed to prevent memory leaks.
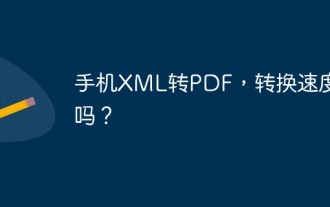
The speed of mobile XML to PDF depends on the following factors: the complexity of XML structure. Mobile hardware configuration conversion method (library, algorithm) code quality optimization methods (select efficient libraries, optimize algorithms, cache data, and utilize multi-threading). Overall, there is no absolute answer and it needs to be optimized according to the specific situation.
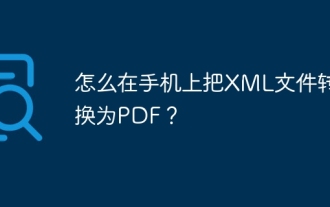
It is impossible to complete XML to PDF conversion directly on your phone with a single application. It is necessary to use cloud services, which can be achieved through two steps: 1. Convert XML to PDF in the cloud, 2. Access or download the converted PDF file on the mobile phone.
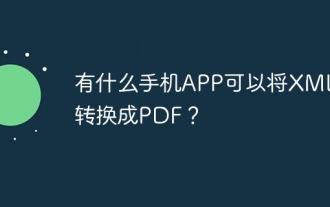
An application that converts XML directly to PDF cannot be found because they are two fundamentally different formats. XML is used to store data, while PDF is used to display documents. To complete the transformation, you can use programming languages and libraries such as Python and ReportLab to parse XML data and generate PDF documents.
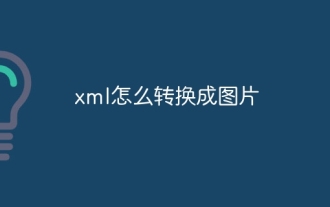
XML can be converted to images by using an XSLT converter or image library. XSLT Converter: Use an XSLT processor and stylesheet to convert XML to images. Image Library: Use libraries such as PIL or ImageMagick to create images from XML data, such as drawing shapes and text.
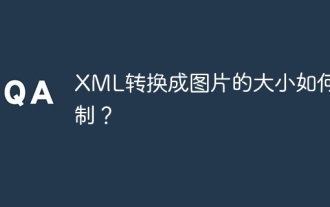
To generate images through XML, you need to use graph libraries (such as Pillow and JFreeChart) as bridges to generate images based on metadata (size, color) in XML. The key to controlling the size of the image is to adjust the values of the <width> and <height> tags in XML. However, in practical applications, the complexity of XML structure, the fineness of graph drawing, the speed of image generation and memory consumption, and the selection of image formats all have an impact on the generated image size. Therefore, it is necessary to have a deep understanding of XML structure, proficient in the graphics library, and consider factors such as optimization algorithms and image format selection.
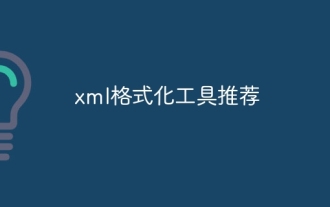
XML formatting tools can type code according to rules to improve readability and understanding. When selecting a tool, pay attention to customization capabilities, handling of special circumstances, performance and ease of use. Commonly used tool types include online tools, IDE plug-ins, and command-line tools.
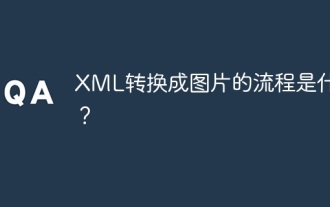
To convert XML images, you need to determine the XML data structure first, then select a suitable graphical library (such as Python's matplotlib) and method, select a visualization strategy based on the data structure, consider the data volume and image format, perform batch processing or use efficient libraries, and finally save it as PNG, JPEG, or SVG according to the needs.
