


How to manage containerization of Python applications using Kubernetes
Aug 02, 2023 am 09:18 AMHow to use Kubernetes to manage containerization of Python applications
Kubernetes is an open source platform for managing containerized deployment, automated scaling, and fault-tolerant recovery of applications. It provides a flexible deployment and expansion mechanism, and can automate the management and monitoring of containers. This article will introduce how to use Kubernetes to manage the containerization of Python applications and provide some simple code examples.
- Preparing a containerized Python application
First, we need to prepare a Python application and containerize it. Suppose we have a simple web application that can be implemented through the Flask framework. Here is a simple example:
# app.py from flask import Flask app = Flask(__name__) @app.route('/') def hello_world(): return 'Hello, World!' if __name__ == '__main__': app.run(host='0.0.0.0', port=5000)
We need to create a Dockerfile to build the container for this application. The following is a simple Dockerfile example:
# Dockerfile FROM python:3.9 WORKDIR /app COPY requirements.txt . RUN pip install --no-cache-dir -r requirements.txt COPY . . EXPOSE 5000 CMD ["python", "app.py"]
In this Dockerfile, we first select a base image (python:3.9) suitable for Python applications, and then copy the application code to the working directory of the container, and installed the required dependencies. Finally, we exposed the application on port 5000 and defined the command to run when the container starts.
- Build Docker Image
After preparing the Dockerfile, we can build the Docker image using the following command:
docker build -t my-python-app .
This will build in the current directory A Docker image named my-python-app.
- Configuring a Kubernetes cluster
Before continuing, we need to configure a Kubernetes cluster. Since Kubernetes installation and configuration is beyond the scope of this article, we assume you already have a working cluster.
- Create Kubernetes Deployment
Next, we need to create a Kubernetes Deployment to manage our application containers. Please create a file called my-python-app-deployment.yaml and add the following content to the file:
# my-python-app-deployment.yaml apiVersion: apps/v1 kind: Deployment metadata: name: my-python-app-deployment spec: replicas: 3 selector: matchLabels: app: my-python-app template: metadata: labels: app: my-python-app spec: containers: - name: my-python-app image: my-python-app ports: - containerPort: 5000
In this Deployment, we have defined 3 replicas to specify the ones we wish to run The number of container replicas. We also define a selector to match our Deployment and specify the name and port of the container image.
- Deploy the application
Next, we can deploy our application using the following command:
kubectl apply -f my-python-app-deployment.yaml
This will create an application called my-python Deployment of -app-deployment and start 3 container copies in the cluster.
- Exposing services
Finally, we need to expose the application's services so that they can be accessed from the outside. Please create a file named my-python-app-service.yaml and add the following content to the file:
# my-python-app-service.yaml apiVersion: v1 kind: Service metadata: name: my-python-app-service spec: selector: app: my-python-app ports: - protocol: TCP port: 80 targetPort: 5000 type: LoadBalancer
In this Service, we specify the port mapping of the container and export it is port 80. We also specify the type of Service as LoadBalancer to automatically create an external load balancer in an environment that supports load balancing.
- Deploy the service
Finally, we can deploy the service to the cluster using the following command:
kubectl apply -f my-python-app-service.yaml
This will create a new file named my-python -Service of app-service and associate it with our Deployment. Kubernetes will automatically create an external load balancer and route traffic to our application container.
Summary
Through the above steps, we successfully used Kubernetes to manage the containerization of a Python application. First, we prepare a Python application and package it as a Docker image. Then, we created a Kubernetes Deployment to containerize the application and defined the number of replicas that needed to be started. Finally, we create a Service to expose the application's services and allow communication with the outside world.
I hope this article will help you understand and use Kubernetes to manage the containerization of Python applications. You can customize the sample code to suit your needs and further extend and optimize the application and its environment.
The above is the detailed content of How to manage containerization of Python applications using Kubernetes. For more information, please follow other related articles on the PHP Chinese website!

Hot Article

Hot tools Tags

Hot Article

Hot Article Tags

Notepad++7.3.1
Easy-to-use and free code editor

SublimeText3 Chinese version
Chinese version, very easy to use

Zend Studio 13.0.1
Powerful PHP integrated development environment

Dreamweaver CS6
Visual web development tools

SublimeText3 Mac version
God-level code editing software (SublimeText3)

Hot Topics
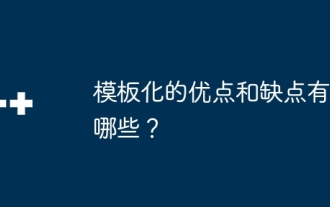
What are the advantages and disadvantages of templating?
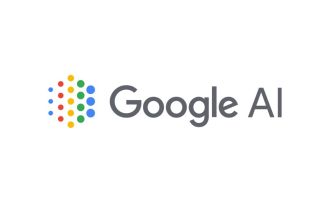
Google AI announces Gemini 1.5 Pro and Gemma 2 for developers
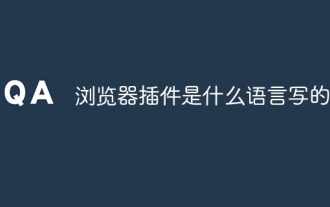
What language is the browser plug-in written in?
