How to extract texture from images using Python
How to use Python to extract texture from images
Introduction: Texture extraction is an important technology in image processing, which can extract texture features from images for Image analysis, classification, matching and other tasks. As a popular programming language, Python has a wealth of image processing libraries and tools, such as PIL, opencv-python, etc. This article will introduce how to use Python to extract texture from images, and attach code examples.
1. Install and import necessary libraries
Before starting, we need to install and import some necessary libraries, including PIL, opencv-python and numpy. They can be installed using pip:
$ pip install Pillow $ pip install opencv-python $ pip install numpy
The code to import the required libraries is as follows:
import cv2 import numpy as np from PIL import Image
2. Reading and displaying images
First, we need to read an image to be processed , and displayed. Here, we selected a natural scenery picture and saved it as "texture.jpg".
# 读取图片 image = Image.open("texture.jpg") # 显示图片 image.show()
3. Convert the image to a grayscale image
Texture extraction is generally performed on a grayscale image, so we need to convert the read color image into a grayscale image.
# 转换为灰度图像 gray_image = image.convert("L") # 显示灰度图像 gray_image.show()
4. Calculate the gray level co-occurrence matrix (GLCM)
The gray level co-occurrence matrix is a common method for texture analysis, which can describe the gray level changes and spatial relationships between pixels.
In Python, we can use the opencv-python library (cv2) to calculate the gray level co-occurrence matrix. The specific steps are as follows:
- First, we need to convert the grayscale image into a numpy array for subsequent processing.
# 将灰度图像转换为numpy数组 gray_array = np.array(gray_image)
- Next, we can use the
cv2.calcHist()
function in the cv2 library to calculate the gray level co-occurrence matrix. This function requires input of a grayscale image and some parameters, such as the number of grayscale levels, pixel intervals, image height and width, etc.
# 计算灰度共生矩阵 glcm = cv2.calcHist([gray_array], [0], None, [256], [0, 256])
- Finally, we can normalize the gray-level co-occurrence matrix to facilitate subsequent analysis and feature extraction.
# 规范化灰度共生矩阵 glcm /= glcm.sum()
5. Texture feature extraction
After obtaining the gray level co-occurrence matrix, we can use it to extract some common texture features, such as energy, contrast, correlation Correlation and homogeneity, etc.
The following are several common texture feature calculation methods:
- Energy (energy): used to measure the clarity of texture. The calculation formula is that the energy is equal to the sum of the squares of each element in the gray-level co-occurrence matrix.
# 计算能量(energy) energy = np.sum(glcm ** 2) print("能量:", energy)
- Contrast: Used to measure the contrast between gray levels in a texture. The calculation formula is that the contrast is equal to the sum of each element in the gray-level co-occurrence matrix multiplied by the pixel difference at the corresponding position.
# 计算对比度(contrast) contrast = np.sum(glcm * np.abs(np.arange(256) - np.arange(256)[:,None])) print("对比度:", contrast)
- Correlation: used to measure the linear correlation of textures. The calculation formula is that the correlation is equal to the sum of the products of each element in the gray-level co-occurrence matrix multiplied by the pixels at the corresponding positions.
# 计算相关性(correlation) correlation = np.sum(glcm * (np.arange(256) - np.mean(glcm)) * (np.arange(256)[:,None] - np.mean(glcm))) / (np.std(glcm) ** 2) print("相关性:", correlation)
- Homogeneity: Used to measure the smoothness between gray levels in a texture. The calculation formula is that uniformity is equal to the sum of each element in the gray-level co-occurrence matrix divided by the pixel difference at the corresponding position.
# 计算均匀性(homogeneity) homogeneity = np.sum(glcm / (1 + np.abs(np.arange(256) - np.arange(256)[:,None]))) print("均匀性:", homogeneity)
6. Summary
This article introduces how to use Python to extract texture from images and how to calculate common texture features. By using PIL and opencv-python, two commonly used image processing libraries, we can easily read and display images, convert images into grayscale images, and calculate the grayscale co-occurrence matrix of the image. After obtaining the gray-level co-occurrence matrix, we also introduced how to calculate common texture features, including energy, contrast, correlation, and uniformity.
Texture analysis is an important research direction in computer vision and plays an important role in image analysis, classification, retrieval and other tasks. I hope this article will be helpful to readers who learn and apply texture extraction technology.
The above is the detailed content of How to extract texture from images using Python. For more information, please follow other related articles on the PHP Chinese website!

Hot AI Tools

Undresser.AI Undress
AI-powered app for creating realistic nude photos

AI Clothes Remover
Online AI tool for removing clothes from photos.

Undress AI Tool
Undress images for free

Clothoff.io
AI clothes remover

Video Face Swap
Swap faces in any video effortlessly with our completely free AI face swap tool!

Hot Article

Hot Tools

Notepad++7.3.1
Easy-to-use and free code editor

SublimeText3 Chinese version
Chinese version, very easy to use

Zend Studio 13.0.1
Powerful PHP integrated development environment

Dreamweaver CS6
Visual web development tools

SublimeText3 Mac version
God-level code editing software (SublimeText3)

Hot Topics


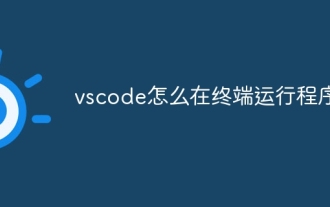
In VS Code, you can run the program in the terminal through the following steps: Prepare the code and open the integrated terminal to ensure that the code directory is consistent with the terminal working directory. Select the run command according to the programming language (such as Python's python your_file_name.py) to check whether it runs successfully and resolve errors. Use the debugger to improve debugging efficiency.
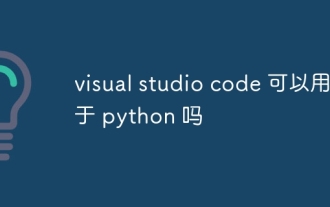
VS Code can be used to write Python and provides many features that make it an ideal tool for developing Python applications. It allows users to: install Python extensions to get functions such as code completion, syntax highlighting, and debugging. Use the debugger to track code step by step, find and fix errors. Integrate Git for version control. Use code formatting tools to maintain code consistency. Use the Linting tool to spot potential problems ahead of time.
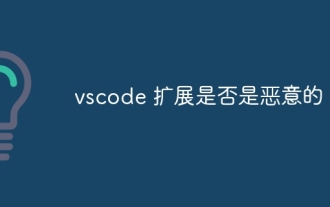
VS Code extensions pose malicious risks, such as hiding malicious code, exploiting vulnerabilities, and masturbating as legitimate extensions. Methods to identify malicious extensions include: checking publishers, reading comments, checking code, and installing with caution. Security measures also include: security awareness, good habits, regular updates and antivirus software.
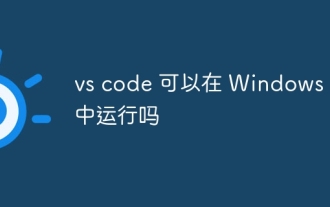
VS Code can run on Windows 8, but the experience may not be great. First make sure the system has been updated to the latest patch, then download the VS Code installation package that matches the system architecture and install it as prompted. After installation, be aware that some extensions may be incompatible with Windows 8 and need to look for alternative extensions or use newer Windows systems in a virtual machine. Install the necessary extensions to check whether they work properly. Although VS Code is feasible on Windows 8, it is recommended to upgrade to a newer Windows system for a better development experience and security.
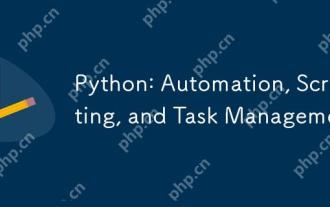
Python excels in automation, scripting, and task management. 1) Automation: File backup is realized through standard libraries such as os and shutil. 2) Script writing: Use the psutil library to monitor system resources. 3) Task management: Use the schedule library to schedule tasks. Python's ease of use and rich library support makes it the preferred tool in these areas.
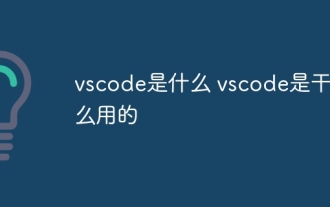
VS Code is the full name Visual Studio Code, which is a free and open source cross-platform code editor and development environment developed by Microsoft. It supports a wide range of programming languages and provides syntax highlighting, code automatic completion, code snippets and smart prompts to improve development efficiency. Through a rich extension ecosystem, users can add extensions to specific needs and languages, such as debuggers, code formatting tools, and Git integrations. VS Code also includes an intuitive debugger that helps quickly find and resolve bugs in your code.
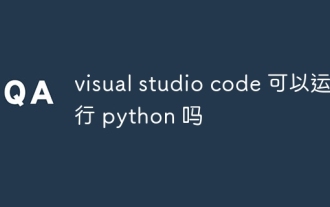
VS Code not only can run Python, but also provides powerful functions, including: automatically identifying Python files after installing Python extensions, providing functions such as code completion, syntax highlighting, and debugging. Relying on the installed Python environment, extensions act as bridge connection editing and Python environment. The debugging functions include setting breakpoints, step-by-step debugging, viewing variable values, and improving debugging efficiency. The integrated terminal supports running complex commands such as unit testing and package management. Supports extended configuration and enhances features such as code formatting, analysis and version control.
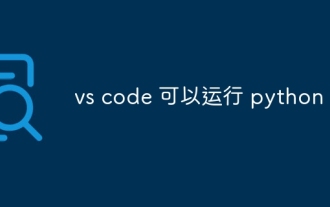
Yes, VS Code can run Python code. To run Python efficiently in VS Code, complete the following steps: Install the Python interpreter and configure environment variables. Install the Python extension in VS Code. Run Python code in VS Code's terminal via the command line. Use VS Code's debugging capabilities and code formatting to improve development efficiency. Adopt good programming habits and use performance analysis tools to optimize code performance.
