How to deal with data accuracy issues in C++ development
How to deal with data precision issues in C development
Abstract: C is a powerful programming language, but when dealing with floating point numbers, due to the limitations of its built-in data types, there will be a problem of loss of precision. . This article will introduce some common data accuracy issues and give corresponding solutions to help C developers better deal with data accuracy issues.
Introduction:
In C development, data accuracy has always been a headache for developers. Whether it is simple mathematical operations or complex scientific calculations, the accuracy requirements for data are very high. Therefore, how to deal with the problem of data accuracy has become an important issue that C developers need to solve.
1. Problem Analysis
Among the built-in data types of C, floating-point number types (float and double) cannot accurately represent all decimal numbers, and rounding errors will occur. This is because floating point numbers are represented in binary, and binary cannot represent certain decimal numbers with complete accuracy. For example, 0.1 loops infinitely in binary representation, so there will be rounding errors when stored. This rounding error accumulates over multiple operations, causing the results to be inconsistent with expectations.
2. Solution
- Use higher-precision data types: C provides some high-precision numerical libraries, such as Boost library and GNU multi-precision library (GMP). These libraries provide data types that can accurately represent large numerical values and can solve floating point precision issues. Developers can use these libraries to replace the built-in floating point types to improve the accuracy of calculations.
- Use fixed-point numbers instead of floating-point numbers: Compared with floating-point numbers, fixed-point numbers are represented in a more precise manner. Fixed-point numbers are represented using a fixed number of decimal places, thus avoiding the rounding errors of floating-point numbers. The disadvantage of fixed-point numbers is that the representation range is smaller, but for some specific application scenarios, such as financial calculations, currency calculations, etc., fixed-point numbers can provide more accurate calculation results.
- Avoid inexact operations on floating-point numbers: When performing floating-point number calculations, you should try to avoid continuous floating-point number operations, especially operations involving large and small values. You can consider converting floating-point numbers into integers for calculations, or use appropriate rounding methods, such as rounding, rounding up, etc., to ensure the accuracy of calculation results.
- Use scientific computing libraries: There are some very good scientific computing libraries in C, such as Eigen and Armadillo. These libraries provide efficient and accurate arithmetic functions for scientific computing and are optimized for floating point precision. Developers can use these libraries to ensure data accuracy when performing scientific calculations.
3. Case Analysis
The following is a simple example to illustrate how to deal with data accuracy issues in C development:
#include <iostream> #include <iomanip> #include <cmath> int main() { double x = 2.0; double y = sqrt(x); std::cout << std::setprecision(16) << y << std::endl; // 输出:1.4142135623730951 return 0; }
In the above example, we calculated The square root of 2, the desired result is 1.414213562373095. However, due to rounding errors in floating point numbers, the final calculation is 1.4142135623730951. If we have higher precision requirements for the results, we can use fixed-point numbers or high-precision numerical libraries instead of the built-in floating point number types.
Conclusion:
The issue of data accuracy is a very important issue in C development. For calculations with high precision requirements, developers should pay attention to selecting appropriate data types and calculation methods. By using high-precision numerical libraries, fixed-point numbers, and scientific computing libraries, you can solve data accuracy problems in C development and improve calculation accuracy. For some special needs, the impact of data accuracy issues can also be reduced through appropriate operation conventions.
The above is the detailed content of How to deal with data accuracy issues in C++ development. For more information, please follow other related articles on the PHP Chinese website!

Hot AI Tools

Undresser.AI Undress
AI-powered app for creating realistic nude photos

AI Clothes Remover
Online AI tool for removing clothes from photos.

Undress AI Tool
Undress images for free

Clothoff.io
AI clothes remover

Video Face Swap
Swap faces in any video effortlessly with our completely free AI face swap tool!

Hot Article

Hot Tools

Notepad++7.3.1
Easy-to-use and free code editor

SublimeText3 Chinese version
Chinese version, very easy to use

Zend Studio 13.0.1
Powerful PHP integrated development environment

Dreamweaver CS6
Visual web development tools

SublimeText3 Mac version
God-level code editing software (SublimeText3)

Hot Topics


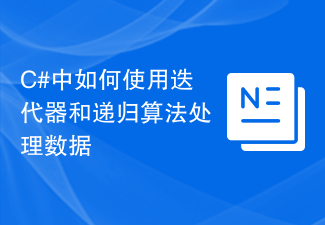
How to use iterators and recursive algorithms to process data in C# requires specific code examples. In C#, iterators and recursive algorithms are two commonly used data processing methods. Iterators can help us traverse the elements in a collection, and recursive algorithms can handle complex problems efficiently. This article details how to use iterators and recursive algorithms to process data, and provides specific code examples. Using Iterators to Process Data In C#, we can use iterators to iterate over the elements in a collection without knowing the size of the collection in advance. Through the iterator, I
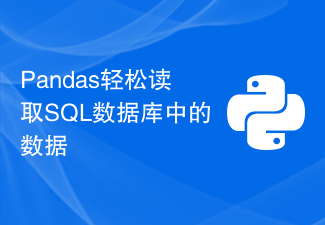
Data processing tool: Pandas reads data in SQL databases and requires specific code examples. As the amount of data continues to grow and its complexity increases, data processing has become an important part of modern society. In the data processing process, Pandas has become one of the preferred tools for many data analysts and scientists. This article will introduce how to use the Pandas library to read data from a SQL database and provide some specific code examples. Pandas is a powerful data processing and analysis tool based on Python
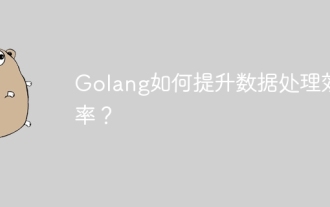
Golang improves data processing efficiency through concurrency, efficient memory management, native data structures and rich third-party libraries. Specific advantages include: Parallel processing: Coroutines support the execution of multiple tasks at the same time. Efficient memory management: The garbage collection mechanism automatically manages memory. Efficient data structures: Data structures such as slices, maps, and channels quickly access and process data. Third-party libraries: covering various data processing libraries such as fasthttp and x/text.
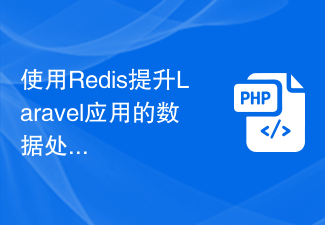
Use Redis to improve the data processing efficiency of Laravel applications. With the continuous development of Internet applications, data processing efficiency has become one of the focuses of developers. When developing applications based on the Laravel framework, we can use Redis to improve data processing efficiency and achieve fast access and caching of data. This article will introduce how to use Redis for data processing in Laravel applications and provide specific code examples. 1. Introduction to Redis Redis is a high-performance memory data
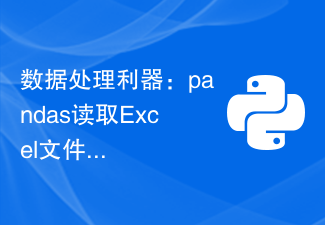
With the increasing popularity of data processing, more and more people are paying attention to how to use data efficiently and make the data work for themselves. In daily data processing, Excel tables are undoubtedly the most common data format. However, when a large amount of data needs to be processed, manually operating Excel will obviously become very time-consuming and laborious. Therefore, this article will introduce an efficient data processing tool - pandas, and how to use this tool to quickly read Excel files and perform data processing. 1. Introduction to pandas pandas
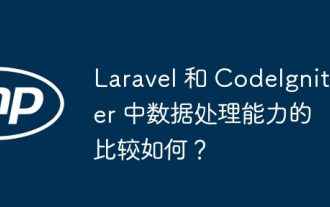
Compare the data processing capabilities of Laravel and CodeIgniter: ORM: Laravel uses EloquentORM, which provides class-object relational mapping, while CodeIgniter uses ActiveRecord to represent the database model as a subclass of PHP classes. Query builder: Laravel has a flexible chained query API, while CodeIgniter’s query builder is simpler and array-based. Data validation: Laravel provides a Validator class that supports custom validation rules, while CodeIgniter has less built-in validation functions and requires manual coding of custom rules. Practical case: User registration example shows Lar
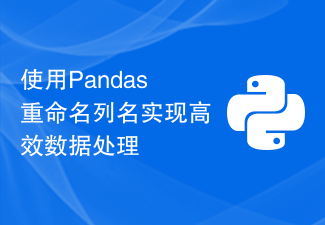
Efficient data processing: Using Pandas to modify column names requires specific code examples. Data processing is a very important part of data analysis, and during the data processing process, it is often necessary to modify the column names of the data. Pandas is a powerful data processing library that provides a wealth of methods and functions to help us process data quickly and efficiently. This article will introduce how to use Pandas to modify column names and provide specific code examples. In actual data analysis, the column names of the original data may have inconsistent naming standards and are difficult to understand.
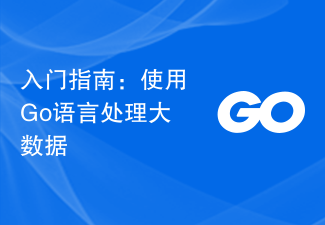
As an open source programming language, Go language has gradually received widespread attention and use in recent years. It is favored by programmers for its simplicity, efficiency, and powerful concurrent processing capabilities. In the field of big data processing, the Go language also has strong potential. It can be used to process massive data, optimize performance, and can be well integrated with various big data processing tools and frameworks. In this article, we will introduce some basic concepts and techniques of big data processing in Go language, and show how to use Go language through specific code examples.
