


Training with 7,500 trajectory data, CMU and Meta allow the robot to reach the level of all-round living room and kitchen
Just by training using 7500 trajectory data, this robot can demonstrate 12 different operating skills in 38 tasks, not just limited to picking and pushing, but also including joint object manipulation and object repositioning . Furthermore, these skills can be applied to hundreds of different unknown situations, including unknown objects, unknown tasks, and even completely unknown kitchen environments. This kind of robot is really cool!
For decades, creating a robot capable of manipulating arbitrary objects in diverse environments has been an elusive goal. One of the reasons is the lack of diverse robotics datasets to train such agents, as well as the lack of general-purpose agents capable of generating such datasets
To overcome this problem, from The authors from Carnegie Mellon University and Meta AI spent two years developing a universal RoboAgent. Their main goal is to develop an efficient paradigm that can train a general agent capable of multiple skills with limited data and generalize these skills to various unknown situations
RoboAgent is modularly composed of:
- RoboPen - a distributed robotics infrastructure built with general-purpose hardware that enables Long term non-stop operation;
- RoboHive - a unified framework for robot learning in simulations and real-world operations;
- RoboSet - A high-quality dataset representing multiple skills using everyday objects in various scenarios;
- MT-ACT - An efficient language-conditional multi-task offline imitation learning framework , by creating diverse semantically enhanced collections based on existing robot experience, thereby expanding the offline data set, and adopting a novel policy architecture and efficient action representation method to recover good performance under limited data budgets strategy.
RoboSet: Multi-skill, multi-task, multi-modal data set
Build one that can be generalized in many different situations Robot agents first need a data set with broad coverage. Given that scaling-up efforts are often helpful (e.g., RT-1 demonstrated results on ~130,000 robot trajectories), there is a need to understand the efficiency and generalization principles of learning systems in the context of limited data sets, often low-data situations. Will lead to overfitting. Therefore, the authors' main goal is to develop a powerful paradigm that can learn generalizable general strategies in low-data situations while avoiding overfitting problems.
The skill and data panorama in robot learning is an important field. In robot learning, skills refer to the abilities that a robot acquires through learning and training and can be used to perform specific tasks. The development of these skills cannot be separated from the support of large amounts of data. Data is the basis for robot learning. By analyzing and processing data, robots can learn from it and improve their skills. Therefore, skills and data are two indispensable aspects of robot learning. Only by continuously learning and acquiring new data can robots continue to improve their skill levels and demonstrate higher intelligence and efficiency in various tasks
The dataset RoboSet (MT-ACT) used to train RoboAgent includes only 7,500 trajectories (18 times less than the data for RT-1). This data set is collected in advance and remains frozen. The dataset consists of high-quality trajectories collected during human teleoperation using commodity robotic hardware (Franka-Emika robot equipped with Robotiq gripper) across multiple tasks and scenarios. RoboSet (MT-ACT) sparsely covers 12 unique skills in several different contexts. Data were collected by dividing daily kitchen activities (e.g. making tea, baking) into different subtasks, each representing a unique skill. The dataset includes common pick-and-place skills, but also contact-rich skills such as wiping, lidding, and skills involving articulated objects. Rewritten content: The dataset used to train RoboAgent, RoboSet (MT-ACT), includes only 7,500 trajectories (18 times less than the data for RT-1). This data set is collected in advance and remains frozen. The dataset consists of high-quality trajectories collected during human teleoperation using commodity robotic hardware (Franka-Emika robot equipped with Robotiq gripper) across multiple tasks and scenarios. RoboSet (MT-ACT) sparsely covers 12 unique skills in several different contexts. Data were collected by dividing daily kitchen activities (e.g. making tea, baking) into different subtasks, each representing a unique skill. The dataset includes common pick-and-place skills, but also contact-rich skills such as wiping, capping, and skills involving articulated objects
## MT-ACT: Multi-task Action Chunking Transformer
RoboAgent learns a common policy in low-data situations based on two key insights. It leverages the underlying model's prior knowledge of the world to avoid mode collapse, and adopts a novel efficient representation strategy capable of ingesting highly multimodal data.
#What needs to be rewritten They are: 1. Semantic enhancement: RoboAgent injects world prior knowledge from the existing basic model into RoboSet (MT-ACT) by semantically enhancing it. The resulting dataset combines the robot's experience with prior knowledge of the world without additional human/robot costs. Use SAM to segment target objects and semantically enhance them in terms of shape, color, and texture changes. Rewritten content: 1. Semantic enhancement: RoboAgent injects world prior knowledge from the existing basic model into RoboSet (MT-ACT) by semantically enhancing it. In this way, the robot's experience and prior knowledge of the world can be combined without additional human/robot costs. Use SAM to segment target objects and perform semantic enhancement in terms of shape, color, and texture changes
2. Efficient strategy representation: The resulting dataset is severely multi-modal , containing a rich variety of skills, tasks, and scenarios. We apply the action chunking method to a multi-task setting and develop a novel and efficient policy representation—MT-ACT—that is able to acquire highly multimodal datasets with small amounts of data while avoiding overfitting. Question
Experimental results
RoboAgent’s sample efficiency is higher than existing methods
The following figure compares the MT-ACT strategy representation proposed by the author with several imitation learning architectures. The author only uses environment changes including object pose changes and partial illumination changes. Similar to previous studies, the authors attribute this to L1 generalization. From the results of RoboAgent, it is clear that using action chunking to model sub-trajectories significantly outperforms all baseline methods, thus further proving the effectiveness of the author's proposed strategy representation in sample-efficient learning
RoboAgent excels at multiple levels of abstraction
The following figure shows the author's results of testing methods at different levels of generalization. At the same time, the generalization level is also demonstrated through visualization, where L1 represents object pose changes, L2 represents diverse desktop backgrounds and interference factors, and L3 represents novel skill-object combinations. Next, the authors show how each method performs at these levels of generalization. In rigorous evaluation studies, MT-ACT performed significantly better than other methods, especially at the more difficult level of generalization (L3)
RoboAgent is highly scalable
The authors evaluated the performance of RoboAgent at increasing levels of semantic enhancement and presented it in a Five skills are assessed in the activity. As can be seen from the figure below, as the data increases (i.e. the number of enhancements per frame increases), the performance improves significantly at all levels of generalization. It is especially worth noting that in the more difficult task (L3 generalization), the performance improvement is more obvious
RoboAgent is able to demonstrate His skills in a variety of different activities
##
The above is the detailed content of Training with 7,500 trajectory data, CMU and Meta allow the robot to reach the level of all-round living room and kitchen. For more information, please follow other related articles on the PHP Chinese website!

Hot AI Tools

Undresser.AI Undress
AI-powered app for creating realistic nude photos

AI Clothes Remover
Online AI tool for removing clothes from photos.

Undress AI Tool
Undress images for free

Clothoff.io
AI clothes remover

Video Face Swap
Swap faces in any video effortlessly with our completely free AI face swap tool!

Hot Article

Hot Tools

Notepad++7.3.1
Easy-to-use and free code editor

SublimeText3 Chinese version
Chinese version, very easy to use

Zend Studio 13.0.1
Powerful PHP integrated development environment

Dreamweaver CS6
Visual web development tools

SublimeText3 Mac version
God-level code editing software (SublimeText3)

Hot Topics




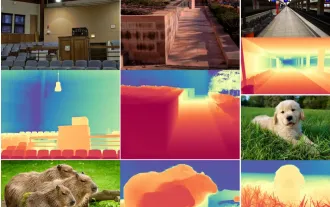
0.What does this article do? We propose DepthFM: a versatile and fast state-of-the-art generative monocular depth estimation model. In addition to traditional depth estimation tasks, DepthFM also demonstrates state-of-the-art capabilities in downstream tasks such as depth inpainting. DepthFM is efficient and can synthesize depth maps within a few inference steps. Let’s read about this work together ~ 1. Paper information title: DepthFM: FastMonocularDepthEstimationwithFlowMatching Author: MingGui, JohannesS.Fischer, UlrichPrestel, PingchuanMa, Dmytr
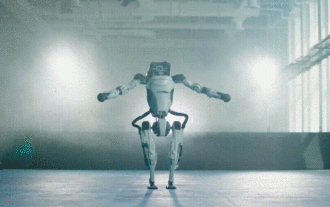
Boston Dynamics Atlas officially enters the era of electric robots! Yesterday, the hydraulic Atlas just "tearfully" withdrew from the stage of history. Today, Boston Dynamics announced that the electric Atlas is on the job. It seems that in the field of commercial humanoid robots, Boston Dynamics is determined to compete with Tesla. After the new video was released, it had already been viewed by more than one million people in just ten hours. The old people leave and new roles appear. This is a historical necessity. There is no doubt that this year is the explosive year of humanoid robots. Netizens commented: The advancement of robots has made this year's opening ceremony look like a human, and the degree of freedom is far greater than that of humans. But is this really not a horror movie? At the beginning of the video, Atlas is lying calmly on the ground, seemingly on his back. What follows is jaw-dropping
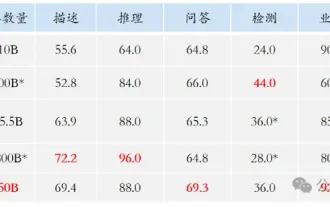
I cry to death. The world is madly building big models. The data on the Internet is not enough. It is not enough at all. The training model looks like "The Hunger Games", and AI researchers around the world are worrying about how to feed these data voracious eaters. This problem is particularly prominent in multi-modal tasks. At a time when nothing could be done, a start-up team from the Department of Renmin University of China used its own new model to become the first in China to make "model-generated data feed itself" a reality. Moreover, it is a two-pronged approach on the understanding side and the generation side. Both sides can generate high-quality, multi-modal new data and provide data feedback to the model itself. What is a model? Awaker 1.0, a large multi-modal model that just appeared on the Zhongguancun Forum. Who is the team? Sophon engine. Founded by Gao Yizhao, a doctoral student at Renmin University’s Hillhouse School of Artificial Intelligence.
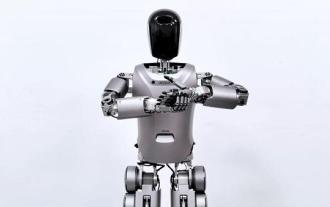
Editor of Machine Power Report: Wu Xin The domestic version of the humanoid robot + large model team completed the operation task of complex flexible materials such as folding clothes for the first time. With the unveiling of Figure01, which integrates OpenAI's multi-modal large model, the related progress of domestic peers has been attracting attention. Just yesterday, UBTECH, China's "number one humanoid robot stock", released the first demo of the humanoid robot WalkerS that is deeply integrated with Baidu Wenxin's large model, showing some interesting new features. Now, WalkerS, blessed by Baidu Wenxin’s large model capabilities, looks like this. Like Figure01, WalkerS does not move around, but stands behind a desk to complete a series of tasks. It can follow human commands and fold clothes
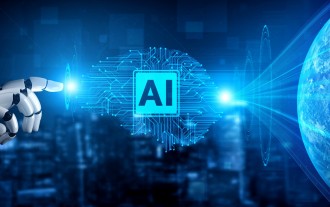
In the field of industrial automation technology, there are two recent hot spots that are difficult to ignore: artificial intelligence (AI) and Nvidia. Don’t change the meaning of the original content, fine-tune the content, rewrite the content, don’t continue: “Not only that, the two are closely related, because Nvidia is expanding beyond just its original graphics processing units (GPUs). The technology extends to the field of digital twins and is closely connected to emerging AI technologies. "Recently, NVIDIA has reached cooperation with many industrial companies, including leading industrial automation companies such as Aveva, Rockwell Automation, Siemens and Schneider Electric, as well as Teradyne Robotics and its MiR and Universal Robots companies. Recently,Nvidiahascoll
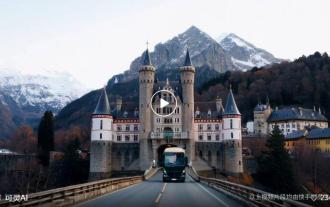
What? Is Zootopia brought into reality by domestic AI? Exposed together with the video is a new large-scale domestic video generation model called "Keling". Sora uses a similar technical route and combines a number of self-developed technological innovations to produce videos that not only have large and reasonable movements, but also simulate the characteristics of the physical world and have strong conceptual combination capabilities and imagination. According to the data, Keling supports the generation of ultra-long videos of up to 2 minutes at 30fps, with resolutions up to 1080p, and supports multiple aspect ratios. Another important point is that Keling is not a demo or video result demonstration released by the laboratory, but a product-level application launched by Kuaishou, a leading player in the short video field. Moreover, the main focus is to be pragmatic, not to write blank checks, and to go online as soon as it is released. The large model of Ke Ling is already available in Kuaiying.
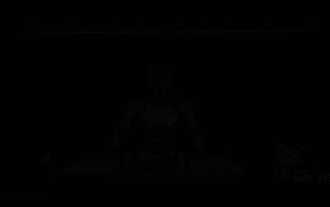
This week, FigureAI, a robotics company invested by OpenAI, Microsoft, Bezos, and Nvidia, announced that it has received nearly $700 million in financing and plans to develop a humanoid robot that can walk independently within the next year. And Tesla’s Optimus Prime has repeatedly received good news. No one doubts that this year will be the year when humanoid robots explode. SanctuaryAI, a Canadian-based robotics company, recently released a new humanoid robot, Phoenix. Officials claim that it can complete many tasks autonomously at the same speed as humans. Pheonix, the world's first robot that can autonomously complete tasks at human speeds, can gently grab, move and elegantly place each object to its left and right sides. It can autonomously identify objects
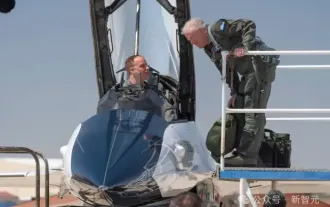
Recently, the military circle has been overwhelmed by the news: US military fighter jets can now complete fully automatic air combat using AI. Yes, just recently, the US military’s AI fighter jet was made public for the first time and the mystery was unveiled. The full name of this fighter is the Variable Stability Simulator Test Aircraft (VISTA). It was personally flown by the Secretary of the US Air Force to simulate a one-on-one air battle. On May 2, U.S. Air Force Secretary Frank Kendall took off in an X-62AVISTA at Edwards Air Force Base. Note that during the one-hour flight, all flight actions were completed autonomously by AI! Kendall said - "For the past few decades, we have been thinking about the unlimited potential of autonomous air-to-air combat, but it has always seemed out of reach." However now,
