


How to improve the data recommendation effect in C++ big data development?
How to improve the data recommendation effect in C big data development?
Abstract:
In today's big data era, data recommendation systems have become an important part of the Internet industry an important technology. In order to improve the data recommendation effect in C big data development, this article will introduce the data recommendation algorithm based on C and some methods to improve the recommendation effect, including data preprocessing, feature engineering, model selection and model evaluation.
1. Data preprocessing
Data preprocessing is the key to improving the effect of data recommendation. In the process of data preprocessing, we need to perform operations such as data cleaning, data filtering and data conversion.
- Data Cleaning
By cleaning the data, data that does not meet the requirements such as noise, outliers, and missing values can be removed. Commonly used data cleaning methods include deduplication, deleting outliers and filling missing values. - Data filtering
In the data filtering process, we can filter and filter data according to business needs and specific rules. For example, we can retain only data relevant to the user's interests based on the user's preferences. - Data transformation
Data transformation is the conversion of raw data into a form usable by machine learning algorithms. When performing data conversion, we can use methods such as one-hot encoding, numericization, and standardization to convert the original data into usable feature vectors.
2. Feature Engineering
Feature engineering is an important link to improve the effect of data recommendation. In feature engineering, we will perform feature extraction, feature selection, and feature combination on the original data.
- Feature extraction
Feature extraction is to extract the most informative features from the original data. Commonly used feature extraction methods include bag-of-words model, TF-IDF, Word2Vec, etc. - Feature selection
Feature selection is to select the most representative features from the extracted features. Commonly used feature selection methods include correlation analysis, chi-square test and mutual information. - Feature combination
Feature combination is to combine multiple features to form a new feature. Commonly used feature combination methods include polynomial feature combination, discretization, and cross features.
3. Model Selection
Model selection is to select the appropriate recommendation model. Commonly used recommendation models in C big data development include collaborative filtering, matrix decomposition, and deep learning. For different data problems, choosing different models can achieve better recommendation results.
4. Model Evaluation
Model evaluation is to evaluate and optimize the effect of the recommended model. In model evaluation, we can use indicators such as cross-validation, precision and recall to evaluate the performance of the model, and perform model tuning based on the evaluation results.
Code example:
The following is a simple example of a collaborative filtering recommendation algorithm implemented in C:
#include <iostream> #include <vector> // 定义用户物品矩阵 std::vector<std::vector<int>> userItemMatrix = { {5, 3, 0, 1}, {4, 0, 0, 1}, {1, 1, 0, 5}, {1, 0, 0, 4}, {0, 1, 5, 4} }; // 计算欧氏距离 double euclideanDistance(const std::vector<int>& vec1, const std::vector<int>& vec2) { double sum = 0.0; for (size_t i = 0; i < vec1.size(); ++i) { sum += (vec1[i] - vec2[i]) * (vec1[i] - vec2[i]); } return sqrt(sum); } // 计算相似度矩阵 std::vector<std::vector<double>> calculateSimilarityMatrix() { std::vector<std::vector<double>> similarityMatrix(userItemMatrix.size(), std::vector<double>(userItemMatrix.size(), 0.0)); for (size_t i = 0; i < userItemMatrix.size(); ++i) { for (size_t j = 0; j < userItemMatrix.size(); ++j) { if (i != j) { double distance = euclideanDistance(userItemMatrix[i], userItemMatrix[j]); similarityMatrix[i][j] = 1 / (1 + distance); } } } return similarityMatrix; } int main() { std::vector<std::vector<double>> similarityMatrix = calculateSimilarityMatrix(); // 输出相似度矩阵 for (size_t i = 0; i < similarityMatrix.size(); ++i) { for (size_t j = 0; j < similarityMatrix[i].size(); ++j) { std::cout << similarityMatrix[i][j] << " "; } std::cout << std::endl; } return 0; }
This example uses the collaborative filtering algorithm to calculate the similarity matrix of a user item matrix . By calculating the Euclidean distance between users and then converting it into similarity, a matrix representing the similarity between users is obtained.
Conclusion:
Through methods such as data preprocessing, feature engineering, model selection and model evaluation, we can improve the data recommendation effect in C big data development. At the same time, the code example shows how to use C to implement a simple collaborative filtering recommendation algorithm for readers' reference and learning.
The above is the detailed content of How to improve the data recommendation effect in C++ big data development?. For more information, please follow other related articles on the PHP Chinese website!

Hot AI Tools

Undresser.AI Undress
AI-powered app for creating realistic nude photos

AI Clothes Remover
Online AI tool for removing clothes from photos.

Undress AI Tool
Undress images for free

Clothoff.io
AI clothes remover

AI Hentai Generator
Generate AI Hentai for free.

Hot Article

Hot Tools

Notepad++7.3.1
Easy-to-use and free code editor

SublimeText3 Chinese version
Chinese version, very easy to use

Zend Studio 13.0.1
Powerful PHP integrated development environment

Dreamweaver CS6
Visual web development tools

SublimeText3 Mac version
God-level code editing software (SublimeText3)

Hot Topics


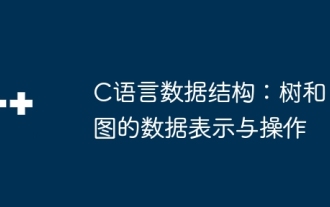
C language data structure: The data representation of the tree and graph is a hierarchical data structure consisting of nodes. Each node contains a data element and a pointer to its child nodes. The binary tree is a special type of tree. Each node has at most two child nodes. The data represents structTreeNode{intdata;structTreeNode*left;structTreeNode*right;}; Operation creates a tree traversal tree (predecision, in-order, and later order) search tree insertion node deletes node graph is a collection of data structures, where elements are vertices, and they can be connected together through edges with right or unrighted data representing neighbors.
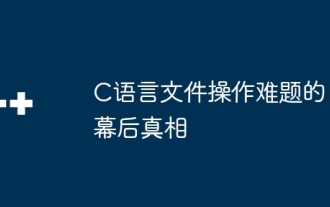
The truth about file operation problems: file opening failed: insufficient permissions, wrong paths, and file occupied. Data writing failed: the buffer is full, the file is not writable, and the disk space is insufficient. Other FAQs: slow file traversal, incorrect text file encoding, and binary file reading errors.
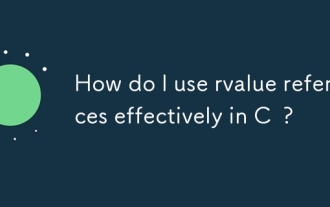
Article discusses effective use of rvalue references in C for move semantics, perfect forwarding, and resource management, highlighting best practices and performance improvements.(159 characters)
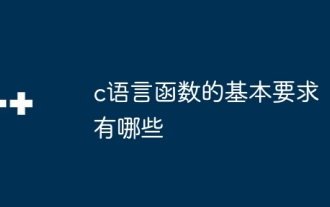
C language functions are the basis for code modularization and program building. They consist of declarations (function headers) and definitions (function bodies). C language uses values to pass parameters by default, but external variables can also be modified using address pass. Functions can have or have no return value, and the return value type must be consistent with the declaration. Function naming should be clear and easy to understand, using camel or underscore nomenclature. Follow the single responsibility principle and keep the function simplicity to improve maintainability and readability.
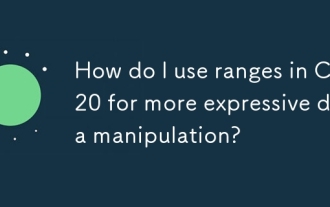
C 20 ranges enhance data manipulation with expressiveness, composability, and efficiency. They simplify complex transformations and integrate into existing codebases for better performance and maintainability.
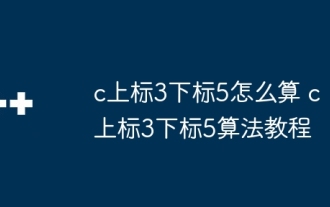
The calculation of C35 is essentially combinatorial mathematics, representing the number of combinations selected from 3 of 5 elements. The calculation formula is C53 = 5! / (3! * 2!), which can be directly calculated by loops to improve efficiency and avoid overflow. In addition, understanding the nature of combinations and mastering efficient calculation methods is crucial to solving many problems in the fields of probability statistics, cryptography, algorithm design, etc.
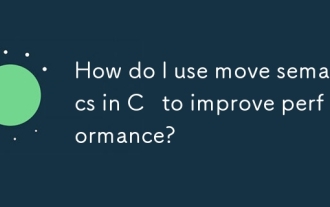
The article discusses using move semantics in C to enhance performance by avoiding unnecessary copying. It covers implementing move constructors and assignment operators, using std::move, and identifies key scenarios and pitfalls for effective appl
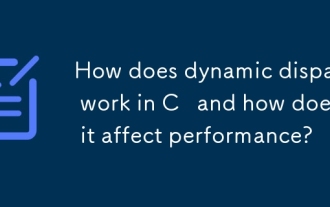
The article discusses dynamic dispatch in C , its performance costs, and optimization strategies. It highlights scenarios where dynamic dispatch impacts performance and compares it with static dispatch, emphasizing trade-offs between performance and
