


How to use Python to perform gradient calculation on images
How to use Python to calculate the gradient of images
Gradient (Gradient) is one of the commonly used technical means in image processing. It calculates the gradient of each pixel in the image. Gradient values can help us understand the edge information of the image and perform other further processing. This article will introduce how to use Python to perform gradient calculation on images, and attach code examples.
1. Principle of gradient calculation
Gradient calculation is based on the brightness change of the image to measure the edge information of the image. In digital images, pixel values are represented by gray levels from 0 to 255. For each pixel, we can obtain the gradient value of that point by calculating the change in gray level of the surrounding pixels.
Common gradient operators include Sobel, Prewitt, Laplacian, etc. Among them, the Sobel operator is the most commonly used operator, which is divided into two directions: horizontal and vertical. By performing the Sobel operation on the image, we can get the gradient values of the image in the horizontal and vertical directions.
2. Steps of gradient calculation
For each pixel, we need to calculate its gradient value in the horizontal and vertical directions. The specific calculation steps are as follows:
- Convert the color image into a grayscale image to facilitate calculation.
- Perform Gaussian filtering on grayscale images to remove noise in the image.
- Calculate the gradient values of the image in the horizontal and vertical directions respectively.
- Combine the gradient values in the horizontal and vertical directions to obtain the gradient amplitude of the image.
3. Use Python for gradient calculation
The following is a code example for using Python for gradient calculation:
import cv2 import numpy as np def gradient(image): # 将彩色图像转换为灰度图像 gray = cv2.cvtColor(image, cv2.COLOR_BGR2GRAY) # 对灰度图像进行高斯滤波 blurred = cv2.GaussianBlur(gray, (3, 3), 0) # 计算水平和垂直方向上的梯度值 sobelx = cv2.Sobel(blurred, cv2.CV_64F, 1, 0, ksize=3) sobely = cv2.Sobel(blurred, cv2.CV_64F, 0, 1, ksize=3) # 合并水平和垂直方向上的梯度值 gradient = np.sqrt(sobelx**2 + sobely**2) # 对梯度幅值进行归一化处理 gradient = cv2.normalize(gradient, None, 0, 255, cv2.NORM_MINMAX, cv2.CV_8U) return gradient # 读取图片 image = cv2.imread('image.jpg') # 调用梯度计算函数 result = gradient(image) # 显示计算结果 cv2.imshow('Gradient Image', result) cv2.waitKey(0)
This code uses the OpenCV library, so it needs Install the corresponding libraries first. The gradient calculation of the image can be realized by calling the cv2.Sobel()
function. The ksize
in the parameter indicates the size of the Sobel operator, which is generally 3. Finally, we normalize the calculated gradient image and display it.
Conclusion
This article introduces how to use Python to perform gradient calculation on images and gives relevant code examples. Gradient calculation is a commonly used technical method in image processing. Mastering this skill can provide a deeper understanding of the edge information of the image and lay the foundation for subsequent image processing work. Hope this article is helpful to you!
The above is the detailed content of How to use Python to perform gradient calculation on images. For more information, please follow other related articles on the PHP Chinese website!

Hot AI Tools

Undresser.AI Undress
AI-powered app for creating realistic nude photos

AI Clothes Remover
Online AI tool for removing clothes from photos.

Undress AI Tool
Undress images for free

Clothoff.io
AI clothes remover

AI Hentai Generator
Generate AI Hentai for free.

Hot Article

Hot Tools

Notepad++7.3.1
Easy-to-use and free code editor

SublimeText3 Chinese version
Chinese version, very easy to use

Zend Studio 13.0.1
Powerful PHP integrated development environment

Dreamweaver CS6
Visual web development tools

SublimeText3 Mac version
God-level code editing software (SublimeText3)

Hot Topics
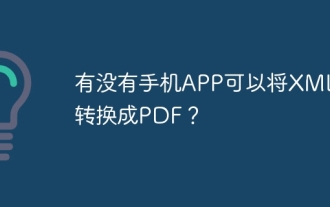
There is no APP that can convert all XML files into PDFs because the XML structure is flexible and diverse. The core of XML to PDF is to convert the data structure into a page layout, which requires parsing XML and generating PDF. Common methods include parsing XML using Python libraries such as ElementTree and generating PDFs using ReportLab library. For complex XML, it may be necessary to use XSLT transformation structures. When optimizing performance, consider using multithreaded or multiprocesses and select the appropriate library.
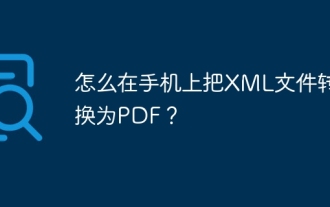
It is impossible to complete XML to PDF conversion directly on your phone with a single application. It is necessary to use cloud services, which can be achieved through two steps: 1. Convert XML to PDF in the cloud, 2. Access or download the converted PDF file on the mobile phone.
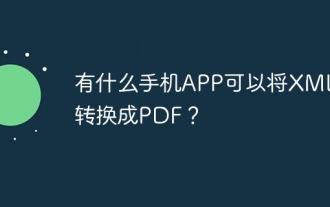
An application that converts XML directly to PDF cannot be found because they are two fundamentally different formats. XML is used to store data, while PDF is used to display documents. To complete the transformation, you can use programming languages and libraries such as Python and ReportLab to parse XML data and generate PDF documents.
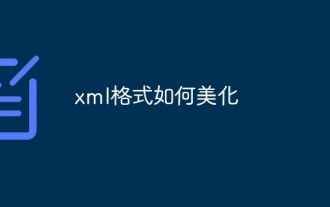
XML beautification is essentially improving its readability, including reasonable indentation, line breaks and tag organization. The principle is to traverse the XML tree, add indentation according to the level, and handle empty tags and tags containing text. Python's xml.etree.ElementTree library provides a convenient pretty_xml() function that can implement the above beautification process.
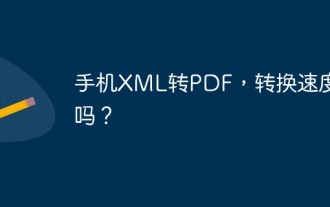
The speed of mobile XML to PDF depends on the following factors: the complexity of XML structure. Mobile hardware configuration conversion method (library, algorithm) code quality optimization methods (select efficient libraries, optimize algorithms, cache data, and utilize multi-threading). Overall, there is no absolute answer and it needs to be optimized according to the specific situation.
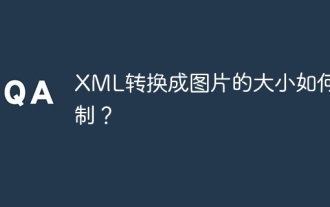
To generate images through XML, you need to use graph libraries (such as Pillow and JFreeChart) as bridges to generate images based on metadata (size, color) in XML. The key to controlling the size of the image is to adjust the values of the <width> and <height> tags in XML. However, in practical applications, the complexity of XML structure, the fineness of graph drawing, the speed of image generation and memory consumption, and the selection of image formats all have an impact on the generated image size. Therefore, it is necessary to have a deep understanding of XML structure, proficient in the graphics library, and consider factors such as optimization algorithms and image format selection.
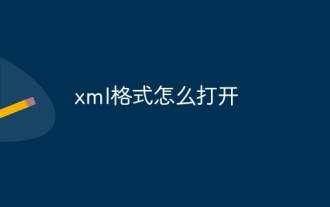
Use most text editors to open XML files; if you need a more intuitive tree display, you can use an XML editor, such as Oxygen XML Editor or XMLSpy; if you process XML data in a program, you need to use a programming language (such as Python) and XML libraries (such as xml.etree.ElementTree) to parse.
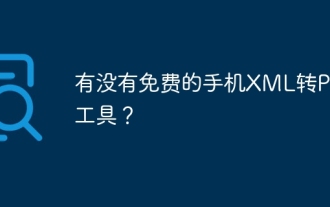
There is no simple and direct free XML to PDF tool on mobile. The required data visualization process involves complex data understanding and rendering, and most of the so-called "free" tools on the market have poor experience. It is recommended to use computer-side tools or use cloud services, or develop apps yourself to obtain more reliable conversion effects.
