How to optimize performance issues in C++ big data development?
How to optimize the performance issues in C big data development?
With the advent of the big data era, C, as an efficient and high-performance programming language, is widely used in Big data development field. However, when processing large-scale data, performance issues often become a bottleneck restricting system efficiency. Therefore, optimizing performance issues in C big data development has become crucial. This article will introduce several performance optimization methods and illustrate them through code examples.
- Use basic data types instead of complex data types
When processing large amounts of data, using basic data types and simple data structures can improve performance than using complex data types. For example, use integers instead of floating point types, use character arrays instead of strings, etc. The following is a sample code:
// 使用基本数据类型替代复杂数据类型 float sum = 0; for (int i = 0; i < size; ++i) { sum += array[i]; // 假设array为一个浮点型数组 }
- Use efficient data structures and algorithms
Choosing appropriate data structures and algorithms can significantly improve the performance of your program. For example, for scenarios that require frequent insertion and deletion operations, using a linked list instead of an array can better meet the needs. The following is a sample code:
// 使用高效的数据结构和算法 std::unordered_map<int, std::string> map; // 使用哈希表来存储键值对 for (int i = 0; i < size; ++i) { map[i] = "value"; // 假设需要频繁地插入键值对 }
- Reasonable use of memory management
Reasonable use of memory management is one of the keys to optimizing performance. Avoiding frequent allocation and freeing of large chunks of memory can improve program efficiency. The following is a sample code:
// 合理使用内存管理 const int size = 10000; int* array = new int[size]; // 使用静态数组代替动态数组 for (int i = 0; i < size; ++i) { array[i] = 0; } delete[] array; // 释放内存
- Parallel processing
For large-scale data processing, you can consider using parallelization to improve performance. Using multi-threading or parallel algorithms can make full use of CPU resources. The following is a sample code:
// 并行化处理 std::vector<int> data = {1, 2, 3, 4, 5, 6, 7, 8, 9, 10}; std::vector<int> result(data.size()); #pragma omp parallel for for (int i = 0; i < data.size(); ++i) { result[i] = data[i] * data[i]; // 假设需要对数据进行平方操作 }
- Using library functions and compilation optimization
C provides a rich standard library and third-party libraries. By using these library functions, you can save development time and Improve program performance. In addition, compiler optimization is also an important means to improve performance. The following is a sample code:
// 使用库函数和编译优化 #include <algorithm> std::vector<int> data = {5, 4, 3, 2, 1}; std::sort(data.begin(), data.end()); // 使用标准库中的排序函数
Through the above methods, the performance issues in C big data development can be significantly improved. Of course, in actual development, performance optimization is a complex process that requires analysis and tuning based on specific problems. Only by continuous learning and practice can we better improve the performance of C big data development.
The above is the detailed content of How to optimize performance issues in C++ big data development?. For more information, please follow other related articles on the PHP Chinese website!

Hot AI Tools

Undresser.AI Undress
AI-powered app for creating realistic nude photos

AI Clothes Remover
Online AI tool for removing clothes from photos.

Undress AI Tool
Undress images for free

Clothoff.io
AI clothes remover

AI Hentai Generator
Generate AI Hentai for free.

Hot Article

Hot Tools

Notepad++7.3.1
Easy-to-use and free code editor

SublimeText3 Chinese version
Chinese version, very easy to use

Zend Studio 13.0.1
Powerful PHP integrated development environment

Dreamweaver CS6
Visual web development tools

SublimeText3 Mac version
God-level code editing software (SublimeText3)

Hot Topics


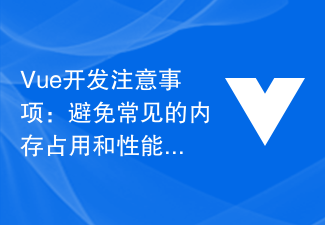
As Vue becomes more and more widely used, Vue developers also need to consider how to optimize the performance and memory usage of Vue applications. This article will discuss some precautions for Vue development to help developers avoid common memory usage and performance problems. Avoid infinite loops When a component continuously updates its own state, or a component continuously renders its own child components, an infinite loop may result. In this case, Vue will run out of memory and make the application very slow. To avoid this situation, Vue provides a
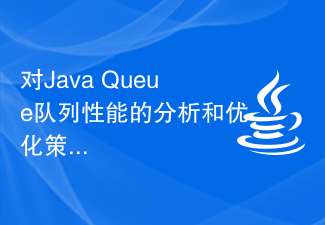
Performance Analysis and Optimization Strategy of JavaQueue Queue Summary: Queue (Queue) is one of the commonly used data structures in Java and is widely used in various scenarios. This article will discuss the performance issues of JavaQueue queues from two aspects: performance analysis and optimization strategies, and give specific code examples. Introduction Queue is a first-in-first-out (FIFO) data structure that can be used to implement producer-consumer mode, thread pool task queue and other scenarios. Java provides a variety of queue implementations, such as Arr
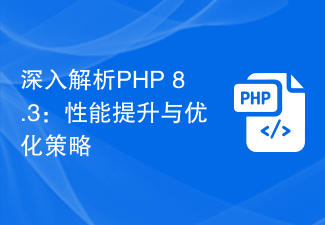
In-depth analysis of PHP8.3: Performance improvement and optimization strategies With the rapid development of Internet technology, PHP, as a very popular server-side programming language, is also constantly evolving and optimizing. The recently released PHP 8.3 version introduces a series of new features and performance optimizations, making PHP even better in terms of execution efficiency and resource utilization. This article will provide an in-depth analysis of the performance improvement and optimization strategies of PHP8.3. First of all, PHP8.3 has made great improvements in performance. The most striking of these is JIT (JIT
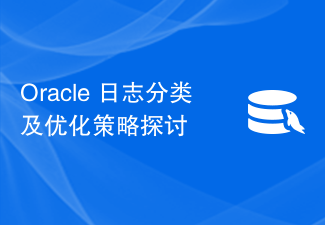
"Discussion on Oracle Log Classification and Optimization Strategies" In the Oracle database, log files are a very important component. They record the activities and changes of the database and ensure the integrity and consistency of the data. For database administrators, it is very critical to effectively manage and optimize database logs to improve database performance and stability. This article will discuss the classification and optimization strategies of logs in Oracle database, and give relevant code examples. 1. Classification of Oracle logs in Oracle data
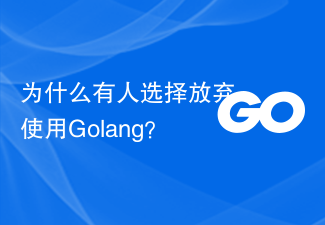
Why do some people choose to give up using Golang? In recent years, with the continuous development of the field of computer science, more and more programming languages have been developed. Among them, Golang, as a programming language with efficient performance and concurrency characteristics, has been widely loved in a certain range. However, despite Golang's many advantages, some developers choose not to use it. So why does this happen? This article will explain it in detail for you from several aspects. First of all, Golang’s design is different from traditional
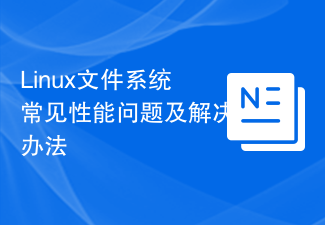
As a common operating system, Linux is widely used in servers, embedded devices and personal computers. However, when using a Linux system, we may encounter some file system performance problems, such as slow response speed, slow file reading and writing, etc. This article will introduce some common file system performance problems and provide corresponding solutions. Disk Fragmentation Disk fragmentation is a common file system performance problem. When files in the file system are frequently created, modified, and deleted, the files on the disk will be scattered.
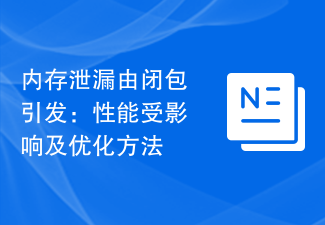
Overview of the impact of memory leaks caused by closures on performance and optimization strategies: Closures are a powerful feature in JavaScript that allow the creation of an independent scope within a function and access to variables and parameters of external functions. However, when using closures, memory leaks are often encountered. This article will discuss the performance impact of memory leaks caused by closures and provide some optimization strategies and specific code examples. Memory leaks caused by closures: In JavaScript, when a function is defined internally
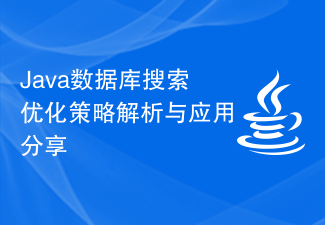
Java database search optimization strategy analysis and application sharing Preface: In development, database search is a very common requirement. However, when the amount of data is large, the search operation may become very time-consuming, seriously affecting the performance of the system. In order to solve this problem, we need to optimize the database search strategy and illustrate it with specific code examples. 1. Use indexes Indexes are a data structure used in databases to speed up searches. By creating indexes on key columns, you can reduce the amount of data your database needs to scan, thereby improving searches
