How to deal with data clustering problems in C++ big data development?
How to deal with the data clustering problem in C big data development?
Data clustering is one of the commonly used technologies in big data analysis. It can combine a large number of Data is divided into different categories or groups to help us understand the similarities and differences between data and discover the rules and patterns hidden behind the data. In the development of C big data, it is very important to correctly handle the data clustering problem. This article will introduce a common data clustering algorithm-k-means algorithm, and provide C code examples to help readers understand and apply this algorithm in depth.
1. Principle of k-means algorithm
k-means algorithm is a simple and powerful clustering algorithm. It divides data into k non-overlapping clusters so that the data points in the clusters are similar. The degree is the highest, while the similarity of data points between clusters is the lowest. The specific implementation process is as follows:
- Initialization: randomly select k data points as the initial clustering center.
- Assignment: Assign each data point to the cluster with its nearest cluster center.
- Update: Calculate a new cluster center for each cluster, that is, move the cluster center to the average position of all data points in the cluster.
- Repeat steps 2 and 3 until the cluster center no longer moves or the predetermined number of iterations is reached.
2. C code example
The following is a simple C code example that demonstrates how to use the k-means algorithm to cluster a set of two-dimensional data points:
#include <iostream> #include <vector> #include <cmath> // 数据点结构体 struct Point { double x; double y; }; // 计算两个数据点之间的欧几里德距离 double euclideanDistance(const Point& p1, const Point& p2) { return std::sqrt(std::pow(p1.x - p2.x, 2) + std::pow(p1.y - p2.y, 2)); } // k均值算法 std::vector<std::vector<Point>> kMeansClustering(const std::vector<Point>& data, int k, int maxIterations) { std::vector<Point> centroids(k); // 聚类中心点 std::vector<std::vector<Point>> clusters(k); // 簇 // 随机选择k个数据点作为初始聚类中心 for (int i = 0; i < k; i++) { centroids[i] = data[rand() % data.size()]; } int iteration = 0; bool converged = false; while (!converged && iteration < maxIterations) { // 清空簇 for (int i = 0; i < k; i++) { clusters[i].clear(); } // 分配数据点到最近的聚类中心所在的簇 for (const auto& point : data) { double minDistance = std::numeric_limits<double>::max(); int closestCluster = -1; for (int i = 0; i < k; i++) { double distance = euclideanDistance(point, centroids[i]); if (distance < minDistance) { minDistance = distance; closestCluster = i; } } clusters[closestCluster].push_back(point); } // 更新聚类中心 converged = true; for (int i = 0; i < k; i++) { if (clusters[i].empty()) { continue; } Point newCentroid{ 0.0, 0.0 }; for (const auto& point : clusters[i]) { newCentroid.x += point.x; newCentroid.y += point.y; } newCentroid.x /= clusters[i].size(); newCentroid.y /= clusters[i].size(); if (newCentroid.x != centroids[i].x || newCentroid.y != centroids[i].y) { centroids[i] = newCentroid; converged = false; } } iteration++; } return clusters; } int main() { // 生成随机的二维数据点 std::vector<Point> data{ { 1.0, 1.0 }, { 1.5, 2.0 }, { 3.0, 4.0 }, { 5.0, 7.0 }, { 3.5, 5.0 }, { 4.5, 5.0 }, { 3.5, 4.5 } }; int k = 2; // 聚类数 int maxIterations = 100; // 最大迭代次数 // 运行k均值算法进行数据聚类 std::vector<std::vector<Point>> clusters = kMeansClustering(data, k, maxIterations); // 输出聚类结果 for (int i = 0; i < k; i++) { std::cout << "Cluster " << i + 1 << ":" << std::endl; for (const auto& point : clusters[i]) { std::cout << "(" << point.x << ", " << point.y << ")" << std::endl; } std::cout << std::endl; } return 0; }
The above code demonstrates how to use the k-means algorithm to cluster a set of two-dimensional data points and output the clustering results. Readers can modify the data and parameters according to actual needs and apply the algorithm to data clustering problems in big data development.
Summary:
This article introduces how to deal with data clustering problems in C big data development, focusing on the k-means algorithm and providing C code examples. Through this code example, readers can understand and apply the k-means algorithm to deal with big data clustering problems. In practical applications, other algorithms can also be combined, such as spectral clustering, hierarchical clustering, etc., to further improve the clustering effect. Data clustering is a very important link in data analysis and big data processing. It can solve the hidden information in the data, discover patterns, and support more accurate decision-making and optimization. I hope this article can provide some help to readers and solve the data clustering problem in big data development.
The above is the detailed content of How to deal with data clustering problems in C++ big data development?. For more information, please follow other related articles on the PHP Chinese website!

Hot AI Tools

Undresser.AI Undress
AI-powered app for creating realistic nude photos

AI Clothes Remover
Online AI tool for removing clothes from photos.

Undress AI Tool
Undress images for free

Clothoff.io
AI clothes remover

Video Face Swap
Swap faces in any video effortlessly with our completely free AI face swap tool!

Hot Article

Hot Tools

Notepad++7.3.1
Easy-to-use and free code editor

SublimeText3 Chinese version
Chinese version, very easy to use

Zend Studio 13.0.1
Powerful PHP integrated development environment

Dreamweaver CS6
Visual web development tools

SublimeText3 Mac version
God-level code editing software (SublimeText3)

Hot Topics


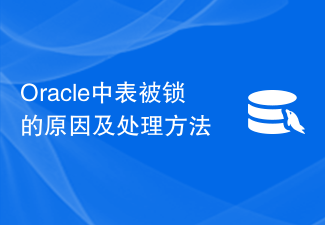
Reasons for table locking in Oracle and how to deal with it In Oracle database, table locking is a common phenomenon, and there are many reasons for table locking. This article will explore some common reasons why tables are locked, and provide some processing methods and related code examples. 1. Types of locks In the Oracle database, locks are mainly divided into shared locks (SharedLock) and exclusive locks (ExclusiveLock). Shared locks are used for read operations, allowing multiple sessions to read the same resource at the same time.
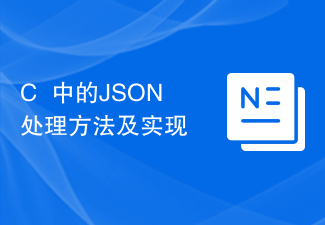
JSON is a lightweight data exchange format that is easy to read and write, as well as easy for machines to parse and generate. Using JSON format makes it easy to transfer data between various systems. In C++, there are many open source JSON libraries for JSON processing. This article will introduce some commonly used JSON processing methods and implementations in C++. JSON processing methods in C++ RapidJSON RapidJSON is a fast C++ JSON parser/generator that provides DOM, SAX and
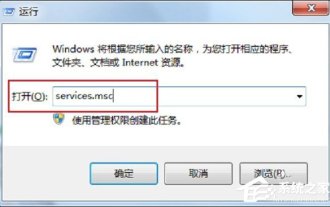
In the process of using computers, we often encounter some problems, some of which can make people overwhelmed. Some users encounter this problem. When they turn on the computer and use the printer, a message that the RPC server is unavailable pops up. What happened? what do I do? In response to this problem, let us share the solution to Win7rpc server being unavailable. 1. Press the Win+R keys to open Run, and enter services.msc in the Run input box. 2. After entering the service list, find the RemoteProcedureCall(RPC)Locator service. 3. Select the service and double-click. The default state is as shown below: 4. Change the startup type of the RPCLoader service to automatic
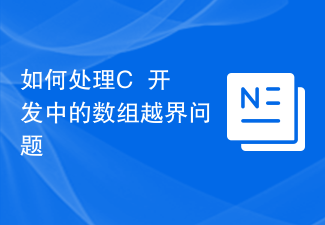
How to deal with the array out-of-bounds problem in C++ development In C++ development, array out-of-bounds is a common error, which can lead to program crashes, data corruption and even security vulnerabilities. Therefore, correctly handling array out-of-bounds problems is an important part of ensuring program quality. This article will introduce some common processing methods and suggestions to help developers avoid array out-of-bounds problems. First, it is key to understand the cause of the array out-of-bounds problem. Array out-of-bounds refers to an index that exceeds its definition range when accessing an array. This usually happens in the following scenario: Negative numbers are used when accessing the array
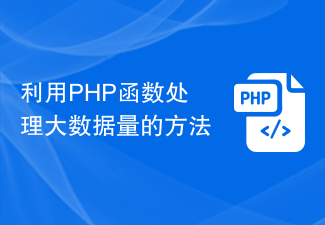
With the development of the Internet, we are exposed to large amounts of data every day, which needs to be stored, processed and analyzed. PHP is a server-side scripting language that is widely used today and is also used for large-scale data processing. When processing large-scale data, it is easy to face memory overflow and performance bottlenecks. This article will introduce how to use PHP functions to process large amounts of data. 1. Turn on memory limit By default, PHP’s memory limit size is 128M, which may become a problem when processing large amounts of data. To handle larger
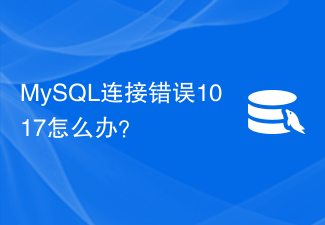
How to deal with MySQL connection error 1017? MySQL is an open source relational database management system that is widely used in website development and data storage. However, when using MySQL, you may encounter a variety of errors. One of the common errors is connection error 1017 (MySQL error code 1017). Connection error 1017 indicates a database connection failure, usually caused by an incorrect username or password. When MySQL fails to authenticate using the provided username and password
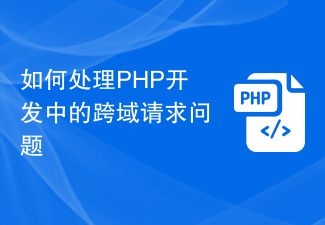
How to deal with cross-domain request issues in PHP development In web development, cross-domain requests are a common problem. When the Javascript code in a web page initiates an HTTP request to access resources under different domain names, a cross-domain request occurs. Cross-domain requests are restricted by the browser's Same-Origin Policy, so in PHP development, we need to take some measures to deal with cross-domain request issues. Using a proxy server to forward requests is a common way to handle cross-domain
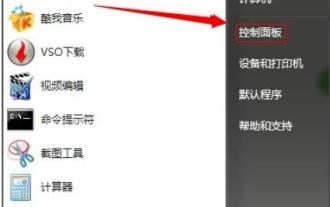
The memory space of the computer depends on the smoothness of the computer's operation. Over time, the memory will become full and the usage will be too high, which will cause the computer to become delayed. So how to solve it? Let’s take a look at the solutions below. What to do if Windows 7 memory usage is too high: Method 1. Disable automatic updates 1. Click "Start" to open "Control Panel" 2. Click "Windows Update" 3. Click "Change Settings" on the left 4. Select the "Never Check for Updates" method 2. Software deletion: Uninstall all useless software. Method 3: Close processes and end all useless processes, otherwise there will be many advertisements in the background filling up the memory. Method 4: Disable services. Many useless services in the system are also closed, which not only ensures security but also saves space.
