How to improve query performance in C++ big data development?
How to improve the query performance in C big data development?
In recent years, with the increasing amount of data and the continuous improvement of processing requirements, C big data development Plays an important role in various fields. However, when processing huge amounts of data, improving query performance becomes a very critical issue. In this article, we will explore some practical tips for improving query performance in C big data development and illustrate them with code examples.
1. Optimize data structure
In big data query, the selection and optimization of data structure are very important. An efficient data structure can reduce query time and improve query performance. The following are some commonly used optimization techniques:
- Use a hash table: A hash table is a fast search data structure that can achieve constant time complexity search operations. When working with large data collections, using hash tables can significantly speed up queries.
- Use index: Index is a data structure that sorts data and can speed up query operations. When processing large data collections, using indexes can reduce the number of data scans, thereby improving query performance.
- Use tree structure: Tree structure is a self-balancing data structure that can quickly locate data. When processing large data collections, using a tree structure can achieve fast range queries and maintain the orderliness of the data.
2. Reasonable use of parallel computing
In big data queries, parallel computing is an important means to improve performance. Proper use of multi-core processors and parallel programming technology can achieve parallel decomposition and parallel execution of query tasks. The following are some commonly used parallel computing techniques:
- Use multi-threading: Multi-threading is a common parallel computing technology that can perform multiple query tasks at the same time and improve query performance. In C, you can use multi-thread libraries such as std::thread or OpenMP to implement multi-thread parallel computing.
- Use a distributed computing framework: For the processing of massive data, single-machine computing may not be able to meet the needs. At this time, a distributed computing framework can be used to distribute the data on multiple machines for processing. Commonly used distributed computing frameworks include Hadoop, Spark, etc.
3. Optimizing query algorithm
In big data query, the optimization of query algorithm is very important. An efficient query algorithm can reduce unnecessary data scanning and calculations, thereby improving query performance. The following are some commonly used query algorithm optimization techniques:
- Binary search: For ordered data collections, you can use the binary search algorithm to quickly locate data. The time complexity of the binary search algorithm is O(logN), which is much lower than the complexity of linear search.
- Filtering and pruning: During the query process, data can be filtered through filter conditions to reduce unnecessary data scanning. For example, you can filter by date range, numerical range, etc. to reduce the amount of data that needs to be scanned when querying.
- Use the divide-and-conquer algorithm: The divide-and-conquer algorithm is an algorithm that decomposes a large problem into multiple small problems and solves them separately. In big data queries, the query task can be decomposed into multiple subtasks, queried separately and finally merged results, thereby reducing query time.
The following is a sample code that uses indexes to optimize queries:
#include <iostream> #include <vector> #include <algorithm> // 定义数据结构 struct Data { int id; std::string name; // 其他字段... }; // 定义索引 struct Index { int id; int index; }; // 查询函数 std::vector<Data> query(int queryId, const std::vector<Data>& data, const std::vector<Index>& index) { std::vector<Data> result; // 使用二分查找定位查询的数据 auto it = std::lower_bound(index.begin(), index.end(), queryId, [](const Index& index, int id) { return index.id < id; }); // 循环查询数据并存入结果 while (it != index.end() && it->id == queryId) { result.push_back(data[it->index]); it++; } return result; } int main() { // 构造测试数据 std::vector<Data> data = { {1, "Alice"}, {2, "Bob"}, {2, "Tom"}, // 其他数据... }; // 构造索引 std::vector<Index> index; for (int i = 0; i < data.size(); i++) { index.push_back({data[i].id, i}); } std::sort(index.begin(), index.end(), [](const Index& a, const Index& b) { return a.id < b.id; }); // 执行查询 int queryId = 2; std::vector<Data> result = query(queryId, data, index); // 输出查询结果 for (const auto& data : result) { std::cout << data.id << " " << data.name << std::endl; } return 0; }
By using indexes for queries, the number of data scans can be greatly reduced and query performance improved.
Summary: In C big data development, optimizing query performance is very important. By optimizing data structures, rationally utilizing parallel computing and optimizing query algorithms, query performance can be improved and program efficiency improved. I hope the introduction and sample code of this article will be helpful to you in improving query performance in C big data development.
The above is the detailed content of How to improve query performance in C++ big data development?. For more information, please follow other related articles on the PHP Chinese website!

Hot AI Tools

Undresser.AI Undress
AI-powered app for creating realistic nude photos

AI Clothes Remover
Online AI tool for removing clothes from photos.

Undress AI Tool
Undress images for free

Clothoff.io
AI clothes remover

AI Hentai Generator
Generate AI Hentai for free.

Hot Article

Hot Tools

Notepad++7.3.1
Easy-to-use and free code editor

SublimeText3 Chinese version
Chinese version, very easy to use

Zend Studio 13.0.1
Powerful PHP integrated development environment

Dreamweaver CS6
Visual web development tools

SublimeText3 Mac version
God-level code editing software (SublimeText3)

Hot Topics


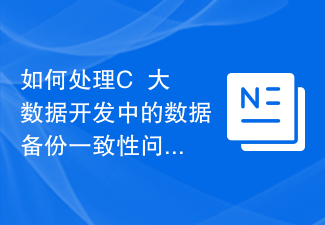
How to deal with the data backup consistency problem in C++ big data development? In C++ big data development, data backup is a very important part. In order to ensure the consistency of data backup, we need to take a series of measures to solve this problem. This article will discuss how to deal with data backup consistency issues in C++ big data development and provide corresponding code examples. Using transactions for data backup Transactions are a mechanism to ensure the consistency of data operations. In C++, we can use the transaction concept in the database to implement data backup.
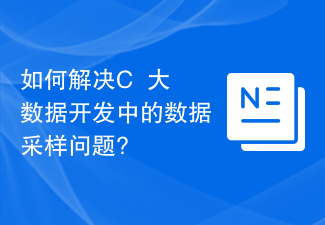
How to solve the data sampling problem in C++ big data development? In C++ big data development, the amount of data is often very large. In the process of processing these big data, a very common problem is how to sample the big data. Sampling is to select a part of sample data from a big data collection for analysis and processing, which can greatly reduce the amount of calculation and increase the processing speed. Below we will introduce several methods to solve the data sampling problem in C++ big data development, and attach code examples. 1. Simple random sampling Simple random sampling is the most common
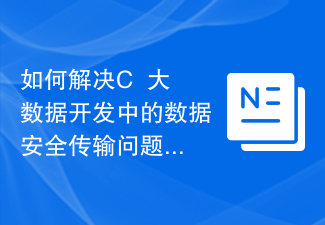
How to solve the problem of data security transmission in C++ big data development? With the rapid development of big data, data security transmission has become an issue that cannot be ignored during the development process. In C++ development, we can ensure the security of data during transmission through encryption algorithms and transmission protocols. This article will introduce how to solve the problem of data security transmission in C++ big data development and provide sample code. 1. Data encryption algorithm C++ provides a rich encryption algorithm library, such as OpenSSL, Crypto++, etc. These libraries can be used
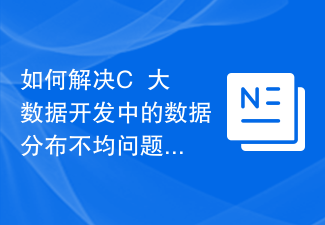
How to solve the problem of uneven data distribution in C++ big data development? In the C++ big data development process, uneven data distribution is a common problem. When the distribution of data is uneven, it will lead to inefficient data processing or even failure to complete the task. Therefore, solving the problem of uneven data distribution is the key to improving big data processing capabilities. So, how to solve the problem of uneven data distribution in C++ big data development? Some solutions are provided below, along with code examples to help readers understand and practice. Data Sharding Algorithm Data Sharding Algorithm is
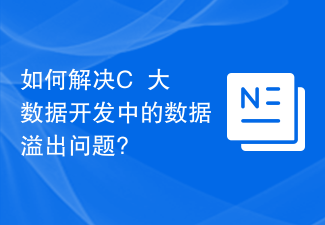
How to solve the data overflow problem in C++ big data development? In the process of C++ big data development, we often encounter the problem of data overflow. Data overflow means that when the value of data exceeds the range that its variable type can represent, it will lead to erroneous results or unpredictable program behavior. In order to solve this problem, we need to take some measures to ensure that the data does not overflow during the calculation process. 1. Choose the appropriate data type In C++, the choice of data type is very important to avoid data overflow problems. According to actual needs, we should
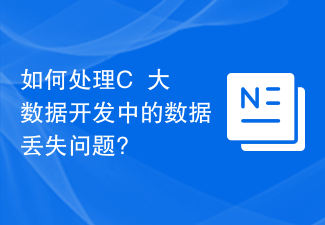
How to deal with the data loss problem in C++ big data development? With the advent of the big data era, more and more companies and developers are beginning to pay attention to big data development. As an efficient and widely used programming language, C++ has also begun to play an important role in big data processing. However, in C++ big data development, the problem of data loss often causes headaches. This article will introduce some common data loss problems and solutions, and provide relevant code examples. Sources of Data Loss Issues Data loss issues can arise from many sources, here are a few
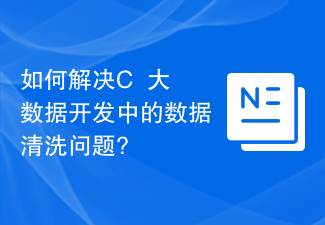
How to solve the data cleaning problem in C++ big data development? Introduction: In big data development, data cleaning is a very important step. Correct, complete, and structured data are the basis for algorithm analysis and model training. This article will introduce how to use C++ to solve data cleaning problems in big data development, and give specific implementation methods through code examples. 1. The concept of data cleaning Data cleaning refers to the preprocessing of original data to make it suitable for subsequent analysis and processing. Mainly includes the following aspects: Missing value processing: deleting or filling missing values
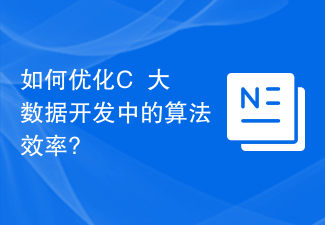
How to optimize algorithm efficiency in C++ big data development? With the continuous development of big data technology, more and more companies and organizations are beginning to pay attention to the efficiency of big data processing. In big data development, the efficiency of algorithms has become an important research direction. In the C++ language, how to optimize algorithm efficiency is a key issue. This article will introduce some methods to optimize algorithm efficiency in C++ big data development and illustrate it through code examples. 1. Selection of data structure In big data processing, the selection of data structure plays an important role in algorithm efficiency.
