How to perform Brown-Forsythe test in Python
The Brown-Forsythe test is a statistical test used to determine whether the variances of two or more groups are equal. Levene's test uses the absolute deviation from the mean, while the Brown-Forsythe test uses the deviation from the median.
The null hypothesis used in the test is as follows -
H0: The variances of the groups (population) are equal
The alternative hypothesis is that the variances are not equal -
H1: The variances of groups (populations) are not equal
To perform the test, we calculate the median of each group and the absolute deviation from the median. We then calculate the F statistic based on the variance of these deviations. Assume that the calculated F statistic is greater than the critical value in the F distribution table. In this case, we reject the null hypothesis and conclude that the variances of the groups are not equal.
In Python, the scipy and statsmodels libraries provide methods to perform Brown-Forsythe tests.
It is worth noting that the Brown-Forsythe test is sensitive to outliers but more robust to non-normality than the Levene test. If the data is abnormal, it is generally recommended to use the Brown-Forsythe test.
Brown ñ Forsythe Test in Python
grammar
levene(sample1, sample2, …sampleN, center=’median’, proportiontocut=0.05)
parameter
sample1, sample2, …sampleN - sample data, may have different lengths. Samples must have only one dimension to be accepted.
Center - Data function for testing. Median is the default value.
Proportiontocut - Indicates the number of data points removed from each end when the center is "trimmed".
illustrate
In the levene() function, the user must pass the one-dimensional sample data of different lengths and the parameter center as "Median". The function then returns the statistics and p_value for the provided sample.
algorithm
Import the levene function from scipy.
Create a data sample on which to perform the Brown-Forsythe test.
Pass sample data to the levene function to execute the test.
Return statistics and p_value from the function.
You can use statistics. The Levene method in the scipy library is used to perform the Brown-Forsythe test.
from scipy.stats import levene group1 = [1, 2, 3, 4, 5] group2 = [2, 3, 4, 5, 6] group3 = [3, 4, 5, 6, 7] statistic, pvalue = levene(group1, group2, group3) print("statistic: ", statistic) print("p-value: ", pvalue)
Output
statistic: 0.0 p-value: 1.0
Here you can see that the p-value is 1, which is greater than 0.05. This means we can accept the null hypothesis. Therefore, the variances of the two groups are the same. Therefore, the alternative hypothesis is rejected.
In addition to implementing the Brown-Forsythe problem, we also need to clarify a common confusion that machine learning engineers encounter. This is how the Brown-Forsythe and ANOVA tests are related to each other.
Brown ñ How are Forsythe’s test and ANOVA test related?
Brown-Forsythe and ANOVA (analysis of variance) tests are related because they test differences in group means. However, they test different hypotheses and have different applications.
Analysis of variance is a statistical method used to test whether there are significant differences between the means of two or more groups. It assumes that the variances of the groups are equal and that the data is normally distributed. Analysis of variance is used to determine whether the means of two or more groups are equal and to compare the variances of the groups.
The Brown-Forsythe test is a variation of Levene's test, which uses the absolute deviation from the mean, whereas the Brown-Forsythe test uses the deviation from the median. The Brown-Forsythe test, on the other hand, is a test of homogeneity of variances, which is a necessary assumption for analysis of variance. Used to determine whether the variances of two or more groups are equal.
In practice, the Brown-Forsythe test is usually performed before analysis of variance to check whether the assumption of equal variances is met. If the variances are not equal, it may be appropriate to use a nonparametric test (such as the Kruskal-Wallis test or Welch's ANOVA test) instead of the regular test.
Brown ñ Forsythe Test Case
Brown-Forsythe test is used in various fields such as biology, medicine, psychology, social sciences, and engineering to test for equal variances in different groups. Some common use cases include -
Comparing the variances of two or more samples - The Brown-Forsythe test determines whether the variances of two or more samples are equal. For example, in medical research, this test can be used to compare the variance of blood pressure measurements in different groups of patients.
Testing for homogeneity of variances before performing an ANOVA - Since the Brown-Forsythe test is a test for homogeneity of variances, it can be used to check whether the assumption of equal variances is met before performing an ANOVA. This ensures that the results of the ANOVA are valid.
Test for equal variances in non-normally distributed data - The Brown-Forsythe test is more robust to non-normality than the Levene test. It can be used to test for equal variances in non-normally distributed data.
Comparing Variances in Repeated Measures Designs - When conducting experiments using a repeated measures design, it is useful to use the Brown-Forsythe test to check for homogeneity of variance between groups.
Quality Control in Manufacturing - The Brown-Forsythe test can be used to check for equal variances in different production batches to ensure consistent product quality.
in conclusion
In summary, the Brown-Forsythe test is a useful statistical method for detecting the presence of heteroskedasticity in a data set. It can be easily implemented in Python using the scipy library. Test results can inform decisions about performing appropriate statistical analysis of the data. By understanding the hypotheses tested and interpreting the results, researchers can better understand the distribution of data and make informed decisions about their analysis.
The above is the detailed content of How to perform Brown-Forsythe test in Python. For more information, please follow other related articles on the PHP Chinese website!

Hot AI Tools

Undresser.AI Undress
AI-powered app for creating realistic nude photos

AI Clothes Remover
Online AI tool for removing clothes from photos.

Undress AI Tool
Undress images for free

Clothoff.io
AI clothes remover

AI Hentai Generator
Generate AI Hentai for free.

Hot Article

Hot Tools

Notepad++7.3.1
Easy-to-use and free code editor

SublimeText3 Chinese version
Chinese version, very easy to use

Zend Studio 13.0.1
Powerful PHP integrated development environment

Dreamweaver CS6
Visual web development tools

SublimeText3 Mac version
God-level code editing software (SublimeText3)

Hot Topics


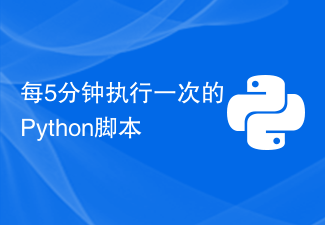
Automation and task scheduling play a vital role in streamlining repetitive tasks in software development. Imagine there is a Python script that needs to be executed every 5 minutes, such as getting data from an API, performing data processing, or sending periodic updates. Running scripts manually so frequently can be time-consuming and error-prone. This is where task scheduling comes in. In this blog post, we will explore how to schedule a Python script to execute every 5 minutes, ensuring it runs automatically without manual intervention. We will discuss different methods and libraries that can be used to achieve this goal, allowing you to automate tasks efficiently. An easy way to run a Python script every 5 minutes using the time.sleep() function is to utilize tim
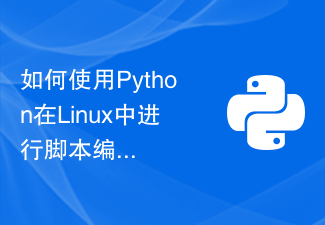
How to use Python to write and execute scripts in Linux In the Linux operating system, we can use Python to write and execute various scripts. Python is a concise and powerful programming language that provides a wealth of libraries and tools to make scripting easier and more efficient. Below we will introduce the basic steps of how to use Python for script writing and execution in Linux, and provide some specific code examples to help you better understand and use it. Install Python
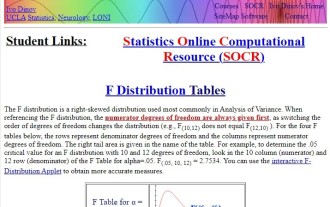
The Brown-Forsythe test is a statistical test used to determine whether the variances of two or more groups are equal. Levene's test uses the absolute deviation from the mean, while the Brown-Forsythe test uses the deviation from the median. The null hypothesis used in the test is as follows - H0: The variances of the groups (population) are equal. The alternative hypothesis is that the variances of the groups (population) are not equal. - H1: The variances of the groups (population) are not equal. To perform the test, we calculate the median of each group and its correlation The absolute deviation of the number of digits. We then calculate the F-statistic based on the variance of these deviations. Assume that the calculated F statistic is greater than the critical value in the F distribution table. In this case, we reject the null hypothesis and conclude that the variances of the groups are not equal. In Python, sc
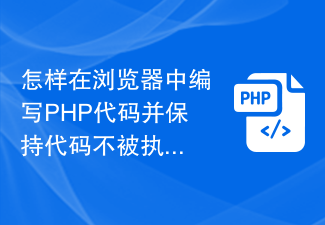
How to write PHP code in the browser and keep the code from being executed? With the popularization of the Internet, more and more people have begun to come into contact with web development, and learning PHP has also attracted more and more attention. PHP is a scripting language that runs on the server side and is often used to write dynamic web pages. However, during the exercise phase, we want to be able to write PHP code in the browser and see the results, but we don't want the code to be executed. So, how to write PHP code in the browser and keep it from being executed? This will be described in detail below. first,
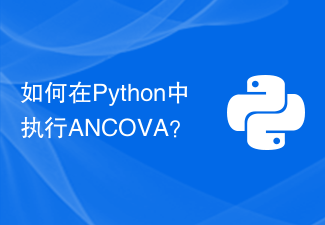
ANCOVA (analysis of covariance) is a useful statistical method because it allows the inclusion of covariates in the analysis, which can help adjust for auxiliary variables and increase the precision of comparisons between groups. These additional factors or covariates can be included in the study by using ANCOVA. To ensure that observed differences between groups are caused by the treatment or intervention in the study and not by extraneous factors, ANCOVA can be used to adjust for the effect of covariates on the group means. This allows for more accurate comparisons between groups and gives more reliable conclusions about the relationships between variables. In this article, we will take a closer look at ANCOVA and implement it in Python. What is ANCOVA? Analysis of covariance (ANCOVA) method compares two or more groups
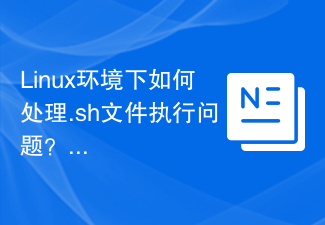
In the Linux environment, we often encounter situations where we need to execute .sh files. The .sh file is a script file in the Linux system, usually with .sh as the suffix, used to execute a series of commands. But sometimes we may encounter the problem that the .sh file cannot be executed. This may be due to permission issues or encoding format and other reasons. This article will introduce how to deal with .sh file execution issues in a Linux environment and provide specific code examples. 1. Add execution permissions in Linux system if a.
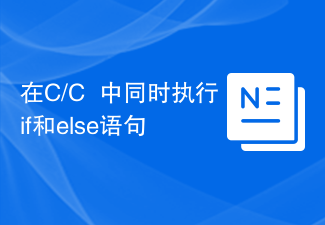
In this section, we will see how to execute both if and else parts in C or C++ code. This solution is a bit tricky. When if and else are executed one after another, it is as if the statement without if-else was executed. But here we will see how to execute them sequentially if they exist. Sample code #include<iostream>usingnamespacestd;intmain(){ intx=10; if(x>5) { &
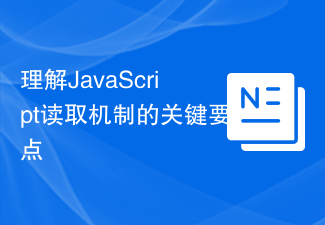
JavaScript is a commonly used programming language that is widely used in web development and front-end technology. In the process of learning JavaScript, understanding its reading mechanism is a very important part. The reading mechanism of JavaScript involves concepts such as variable declaration, scope chain, and closure. Illustrating these key points through specific code examples will help deepen the understanding of the JavaScript reading mechanism. Variable declaration In JavaScript, variable declaration can use var, l
