Golang: a powerful tool for building efficient AI applications
Golang: A powerful tool for creating efficient AI applications
Artificial Intelligence (AI) has become one of the hottest topics in today’s technology field, and it covers Many fields, such as machine learning, deep learning, natural language processing, etc. In the development process of AI applications, choosing the appropriate programming language is crucial. This article will introduce how Golang, as an efficient programming language, can become a powerful tool for creating efficient AI applications.
1. Advantages of Golang
Golang is a statically typed, compiled programming language developed by Google. It has the following advantages:
- Efficient concurrency: Golang has a built-in lightweight coroutine mechanism called goroutine. Through goroutine, concurrent programming can be easily implemented and the performance of multi-core processors can be effectively utilized.
- Powerful standard library: Golang's standard library is very rich, providing a wealth of APIs and tools, such as http, json, crypto, etc., which can greatly reduce the workload of developers.
- Excellent performance: Golang has high execution efficiency by optimizing the compiler and runtime. It is a memory-safe language that automatically manages memory through a garbage collection mechanism and avoids the problem of memory leaks.
- Easy to learn: Golang’s syntax is concise and clear, easy to understand and get started. It doesn't have too many complicated concepts and grammatical rules, making it ideal for rapid development.
2. Golang’s application in AI applications
- Machine learning: Machine learning is one of the most common fields in AI applications. Golang provides a wealth of machine learning libraries, such as tensorflow, golearn, etc. The following is a simple example code for image classification using tensorflow:
package main import ( "github.com/tensorflow/tensorflow/tensorflow/go" "github.com/tensorflow/tensorflow/tensorflow/go/op" "image" _ "image/jpeg" _ "image/png" "io/ioutil" "log" "os" ) func main() { // 读取模型和图片 model, err := ioutil.ReadFile("model.pb") if err != nil { log.Fatal("Error reading model:", err) } imageData, err := ioutil.ReadFile("image.jpg") if err != nil { log.Fatal("Error reading image:", err) } // 创建图和session graph := tensorflow.NewGraph() err = graph.Import(model, "") if err != nil { log.Fatal("Error importing model:", err) } session, err := tensorflow.NewSession(graph, nil) if err != nil { log.Fatal("Error creating session:", err) } defer session.Close() // 图像预处理 img, _, err := image.Decode(bytes.NewReader(imageData)) if err != nil { log.Fatal("Error decoding image:", err) } tensor, err := loadImage(img) if err != nil { log.Fatal("Error creating tensor:", err) } // 运行分类模型 output, err := session.Run( map[tensorflow.Output]*tensorflow.Tensor{ graph.Operation("input").Output(0): tensor, }, []tensorflow.Output{ graph.Operation("output").Output(0), }, nil, ) if err != nil { log.Fatal("Error running model:", err) } // 处理输出结果 result := output[0].Value().([][]float32)[0] log.Println("Result:", result) } func loadImage(img image.Image) (*tensorflow.Tensor, error) { bounds := img.Bounds() width, height := bounds.Max.X, bounds.Max.Y rgba := image.NewRGBA(bounds) draw.Draw(rgba, bounds, img, bounds.Min, draw.Src) tensor, err := tensorflow.NewTensor(rgba.Pix, tensorflow.Uint8, []int{1, height, width, 3}) if err != nil { return nil, err } return tensor, nil }
- Natural Language Processing: Natural language processing is another common AI application area. Golang provides multiple natural language processing libraries, such as go-nlp, go-nlp-tools, etc. The following is a simple sample code using go-nlp for text classification:
package main import ( "fmt" "github.com/nu7hatch/gouuid" "github.com/nu7hatch/gouuid" "github.com/nu7hatch/gouuid" "github.com/nu7hatch/gouuid" "github.com/nu7hatch/gouuid" "github.com/nu7hatch/gouuid" ) func main() { // 创建分类器 classifier := nlp.NewClassifier(nlp.NaiveBayes) // 添加训练数据 classifier.Train("I love Golang", "positive") classifier.Train("Golang is awesome", "positive") classifier.Train("I hate Golang", "negative") classifier.Train("Golang is terrible", "negative") // 对测试数据进行分类 fmt.Println(classifier.Classify("I like Golang")) // Output: positive fmt.Println(classifier.Classify("I dislike Golang")) // Output: negative }
The above two sample codes show a simple method to implement machine learning and natural language processing under Golang. Golang's ease of learning and excellent performance make it an ideal choice for AI application development.
Summary:
As an efficient programming language, Golang has the advantages of concurrency capabilities, rich standard libraries, excellent performance, and ease of learning, making it a powerful tool for creating efficient AI applications. Through Golang, we can easily implement various AI applications, such as machine learning, natural language processing, etc. I hope this article has provided some help for you to understand the application of Golang in AI applications.
The above is the detailed content of Golang: a powerful tool for building efficient AI applications. For more information, please follow other related articles on the PHP Chinese website!

Hot AI Tools

Undresser.AI Undress
AI-powered app for creating realistic nude photos

AI Clothes Remover
Online AI tool for removing clothes from photos.

Undress AI Tool
Undress images for free

Clothoff.io
AI clothes remover

Video Face Swap
Swap faces in any video effortlessly with our completely free AI face swap tool!

Hot Article

Hot Tools

Notepad++7.3.1
Easy-to-use and free code editor

SublimeText3 Chinese version
Chinese version, very easy to use

Zend Studio 13.0.1
Powerful PHP integrated development environment

Dreamweaver CS6
Visual web development tools

SublimeText3 Mac version
God-level code editing software (SublimeText3)

Hot Topics


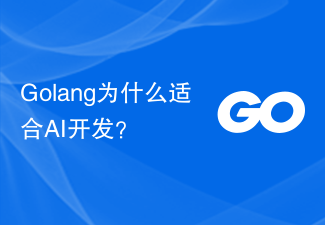
Why is Golang suitable for AI development? With the rapid development of artificial intelligence (AI) technology, more and more developers and researchers have begun to pay attention to the potential of using the Golang programming language in the field of AI. Golang (also known as Go) is an open source programming language developed by Google. It is loved by developers for its high performance, high concurrency and simplicity and ease of use. This article will explore why Golang is suitable for AI development and provide some sample code to demonstrate Golang's advantages in the AI field. High sex

Golang Development: Building a Distributed File Storage System In recent years, with the rapid development of cloud computing and big data, the demand for data storage has continued to increase. In order to cope with this trend, distributed file storage systems have become an important technical direction. This article will introduce how to build a distributed file storage system using the Golang programming language and provide specific code examples. 1. Design of distributed file storage system A distributed file storage system is a system that stores file data dispersedly on multiple machines. It divides the data into multiple blocks.
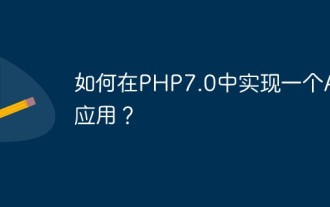
With the rapid development of artificial intelligence, more and more developers have begun to research and develop AI applications. As a language widely used in web development, PHP also has its own tools and frameworks related to AI application development. This article will introduce how to implement an AI application based on machine learning in PHP7.0. Step 1: Choose a suitable machine learning library Machine learning is one of the most mainstream applications in the current development of AI technology. In PHP7.0, we can choose to use scikit-learn or php-m
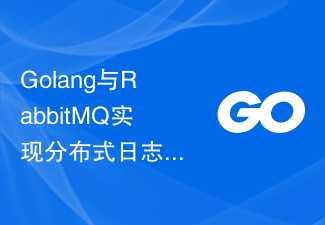
Details, techniques, and best practices for implementing distributed log collection and analysis with Golang and RabbitMQ. In recent years, with the popularity of microservice architecture and the complexity of large-scale systems, log collection and analysis have become more and more important. In a distributed system, the logs of each microservice are often scattered in different places. How to efficiently collect and analyze these logs becomes a challenge. This article will introduce the details, techniques, and best practices on how to use Golang and RabbitMQ to implement distributed log collection and analysis. Ra
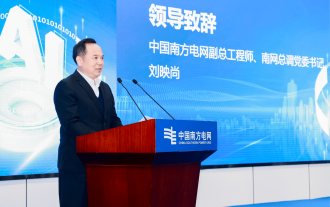
The power market clearing speed has set a new record. On December 27, China Southern Power Grid’s fifth power dispatching AI application competition concluded successfully in Guangzhou. The Tsinghua University team won the championship with a perfect score. Relying on "cloud + AI" technology, the overall clearing process was controlled within 600 seconds, improving efficiency by nearly 50%. When supply and demand are in equilibrium, it is called clearing. With the further advancement of power market transactions, new entities such as photovoltaics, energy storage and virtual power plants have joined in. How to quickly clear electricity as a commodity has attracted much attention in the industry. In July 2022, the southern regional power market led by China Southern Power Grid was officially launched, becoming the first regional market in the country to be put into trial operation. Currently, 160,000 trading entities conduct electricity spot transactions on the platform, achieving free and real-time transactions.

It’s a stormy holiday season, the busiest travel season of the year, and you’ve just received a notification you least expected to see: a serious flight delay. When you want to contact airline customer service, it usually means you’ll have to spend Waited for half an hour for customer service to get through. However, the communication process seemed a little different this time. You discover that the airline uses an AI chatbot and you have a brief text exchange. The bot is able to quickly assess your situation and put you in a priority queue. Soon, a human agent takes over the conversation, verifies the details, and rebookes you on an earlier flight. That way, you can catch your next flight and get home to your family in time. Generative AI is becoming a critical component of business operations and customer service interactions. According to S
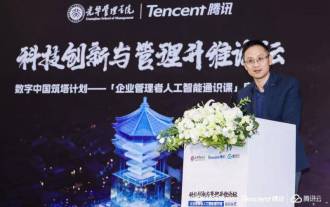
On June 21, Peking University Guanghua School of Management and Tencent announced the upgrade of the "Digital China Tower Building Plan" and jointly launched a series of courses "Artificial Intelligence General Course for Business Managers". In the first lesson, Tang Daosheng, Senior Executive Vice President of Tencent Group and CEO of Cloud and Smart Industry Group, reviewed the history of AI development and said that the superposition of three major factors, namely algorithm innovation, increased computing power, and open source co-creation, constituted the development of AI. "Growth flywheel". The rapid progress of large models has pushed us into an era reshaped by AI. Tang Daosheng said that the large model is only the starting point. In the future, the industrial transformation of application implementation will be a bigger picture. In the past, enterprises relied on people to judge, coordinate and communicate in R&D, production, sales, service and other links. Today, it is worth taking a look at which ones
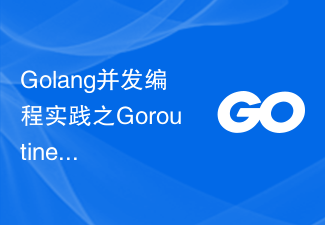
Introduction to the application scenario analysis of Goroutines in Golang concurrent programming practice: With the continuous improvement of computer performance, multi-core processors have become mainstream. In order to make full use of the advantages of multi-core processors, we need to use concurrent programming technology to implement multi-threaded operations. In the Go language, Goroutines (coroutines) are a very powerful concurrent programming mechanism that can be used to achieve efficient concurrent operations. In this article, we will explore the application scenarios of Goroutines and give some examples.
