


Learn more about Python's innovative applications in natural language processing
In-depth understanding of Python’s innovative applications in natural language processing
Natural Language Processing (NLP) is an important technology in the field of artificial intelligence. It involves the ability of machines to understand and process human language. As a high-level programming language, Python has rich libraries and tools, providing strong support for innovative applications in the field of NLP. In this article, we will delve into the innovative applications of Python in the field of NLP and give some example codes.
- Text processing
In NLP, text preprocessing is a very important step. Python provides the nltk library (Natural Language Toolkit), which contains various text processing functions and algorithms. The following is an example that shows how to use the nltk library for text segmentation and word frequency statistics:
import nltk from nltk.corpus import stopwords from nltk.tokenize import word_tokenize from nltk.probability import FreqDist # 加载英文停用词 nltk.download('stopwords') # 加载文本数据 text = "The quick brown fox jumps over the lazy dog." # 文本分词 tokens = word_tokenize(text.lower()) # 去除停用词 stop_words = set(stopwords.words('english')) tokens = [word for word in tokens if word.isalpha() and word not in stop_words] # 词频统计 freq_dist = FreqDist(tokens) # 输出词频结果 for word, freq in freq_dist.items(): print(word, freq)
The above example first introduces the nltk library and downloads the required stop vocabulary library. Next, we define a piece of English text, and then use the word_tokenize
function to segment the text. After that, by removing stop words and non-alphabetic characters, we get the cleaned text. Finally, use the FreqDist
class to perform word frequency statistics on the cleaned text and output the results.
- Sentiment Analysis
Sentiment analysis is an important research direction in the field of NLP. It aims to determine the emotional tendency in a given text, such as positive, negative or neutral. Python provides a variety of methods and libraries to implement sentiment analysis, the most commonly used of which is classification using machine learning algorithms.
The following is an example that shows how to use the scikit-learn library for sentiment analysis:
from sklearn.feature_extraction.text import TfidfVectorizer from sklearn.model_selection import train_test_split from sklearn.svm import SVC from sklearn.metrics import accuracy_score # 加载情感分类数据集 # ... # 文本特征提取 vectorizer = TfidfVectorizer() X = vectorizer.fit_transform(text_list) # 划分训练集和测试集 X_train, X_test, y_train, y_test = train_test_split(X, labels, test_size=0.2) # 训练模型 model = SVC() model.fit(X_train, y_train) # 预测 y_pred = model.predict(X_test) # 计算准确率 accuracy = accuracy_score(y_test, y_pred) print("Accuracy:", accuracy)
In the above example, we first load the sentiment classification dataset and define a TfidfVectorizer
class to extract text features. Then, use the train_test_split
function to divide the data set into a training set and a test set. Next, we train the model using the support vector machine (SVM) algorithm and make predictions on the test set. Finally, use the accuracy_score
function to calculate the prediction accuracy.
- Machine Translation
Machine translation is an important application in the field of NLP, which aims to automatically convert text in one language into another language. Python provides a packaging library for the Google Translate APIgoogletrans
, which can facilitate machine translation.
Here is an example that shows how to use the googletrans
library for machine translation:
from googletrans import Translator # 创建翻译器对象 translator = Translator(service_urls=['translate.google.cn']) # 设置源语言和目标语言 src_lang = 'en' target_lang = 'zh-CN' # 待翻译的文本 text = "Hello, how are you?" # 机器翻译 result = translator.translate(text, src=src_lang, dest=target_lang) # 输出翻译结果 print(result.text)
In the above example, we first create a translator object , and set the source language and target language. Next, we define the text to be translated and then use the translate
method to translate it. Finally, output the translation results.
Through the above examples, we can see the innovative applications of Python in the field of natural language processing, including text processing, sentiment analysis, and machine translation. Python provides a wealth of libraries and tools to make these tasks easier and more efficient. I hope this article can help readers gain a deeper understanding of the application of Python in NLP and inspire innovative ideas.
The above is the detailed content of Learn more about Python's innovative applications in natural language processing. For more information, please follow other related articles on the PHP Chinese website!

Hot AI Tools

Undresser.AI Undress
AI-powered app for creating realistic nude photos

AI Clothes Remover
Online AI tool for removing clothes from photos.

Undress AI Tool
Undress images for free

Clothoff.io
AI clothes remover

AI Hentai Generator
Generate AI Hentai for free.

Hot Article

Hot Tools

Notepad++7.3.1
Easy-to-use and free code editor

SublimeText3 Chinese version
Chinese version, very easy to use

Zend Studio 13.0.1
Powerful PHP integrated development environment

Dreamweaver CS6
Visual web development tools

SublimeText3 Mac version
God-level code editing software (SublimeText3)

Hot Topics


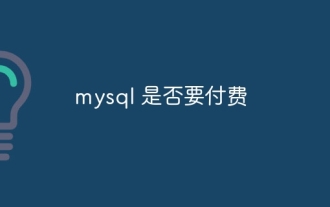
MySQL has a free community version and a paid enterprise version. The community version can be used and modified for free, but the support is limited and is suitable for applications with low stability requirements and strong technical capabilities. The Enterprise Edition provides comprehensive commercial support for applications that require a stable, reliable, high-performance database and willing to pay for support. Factors considered when choosing a version include application criticality, budgeting, and technical skills. There is no perfect option, only the most suitable option, and you need to choose carefully according to the specific situation.

HadiDB: A lightweight, high-level scalable Python database HadiDB (hadidb) is a lightweight database written in Python, with a high level of scalability. Install HadiDB using pip installation: pipinstallhadidb User Management Create user: createuser() method to create a new user. The authentication() method authenticates the user's identity. fromhadidb.operationimportuseruser_obj=user("admin","admin")user_obj.
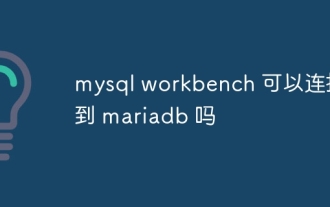
MySQL Workbench can connect to MariaDB, provided that the configuration is correct. First select "MariaDB" as the connector type. In the connection configuration, set HOST, PORT, USER, PASSWORD, and DATABASE correctly. When testing the connection, check that the MariaDB service is started, whether the username and password are correct, whether the port number is correct, whether the firewall allows connections, and whether the database exists. In advanced usage, use connection pooling technology to optimize performance. Common errors include insufficient permissions, network connection problems, etc. When debugging errors, carefully analyze error information and use debugging tools. Optimizing network configuration can improve performance
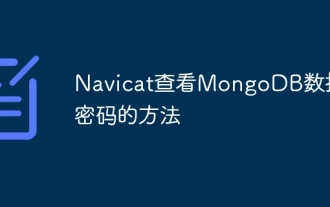
It is impossible to view MongoDB password directly through Navicat because it is stored as hash values. How to retrieve lost passwords: 1. Reset passwords; 2. Check configuration files (may contain hash values); 3. Check codes (may hardcode passwords).
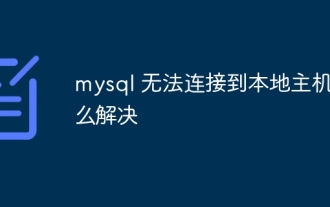
The MySQL connection may be due to the following reasons: MySQL service is not started, the firewall intercepts the connection, the port number is incorrect, the user name or password is incorrect, the listening address in my.cnf is improperly configured, etc. The troubleshooting steps include: 1. Check whether the MySQL service is running; 2. Adjust the firewall settings to allow MySQL to listen to port 3306; 3. Confirm that the port number is consistent with the actual port number; 4. Check whether the user name and password are correct; 5. Make sure the bind-address settings in my.cnf are correct.
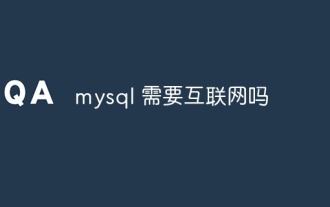
MySQL can run without network connections for basic data storage and management. However, network connection is required for interaction with other systems, remote access, or using advanced features such as replication and clustering. Additionally, security measures (such as firewalls), performance optimization (choose the right network connection), and data backup are critical to connecting to the Internet.
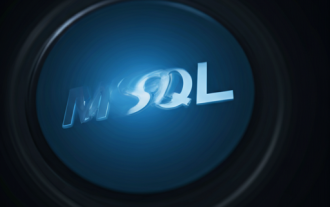
MySQL database performance optimization guide In resource-intensive applications, MySQL database plays a crucial role and is responsible for managing massive transactions. However, as the scale of application expands, database performance bottlenecks often become a constraint. This article will explore a series of effective MySQL performance optimization strategies to ensure that your application remains efficient and responsive under high loads. We will combine actual cases to explain in-depth key technologies such as indexing, query optimization, database design and caching. 1. Database architecture design and optimized database architecture is the cornerstone of MySQL performance optimization. Here are some core principles: Selecting the right data type and selecting the smallest data type that meets the needs can not only save storage space, but also improve data processing speed.
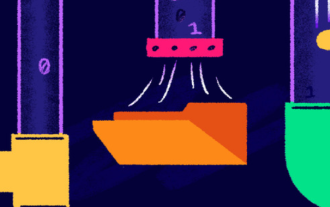
As a data professional, you need to process large amounts of data from various sources. This can pose challenges to data management and analysis. Fortunately, two AWS services can help: AWS Glue and Amazon Athena.
