Several attack methods against large AI models
With the application and promotion of new technologies such as artificial intelligence and big data, large models have also become a popular technology. Of course, organizations and individuals will begin to use various technologies to attack them.
There are many types of attacks against models, among which several are often mentioned:
(1) Adversarial sample attack:
Adversarial sample attack is one of the most widely used machine learning attack methods. During the attack, the attacker generates adversarial samples by adding small perturbations to the original data samples (such as misclassification or prediction that can fool the model), and misleads the classifier output of the machine learning model while keeping the model function unchanged. .
(2) Data poisoning attack:
Data poisoning attack is to destroy or destroy the use of the model by adding erroneous or disturbing data to the training data.
Note: Adversarial sample attacks and data poisoning attacks are somewhat similar, but the focus is different.
(3) Model stealing attack:
This is a model reversal and stealing attack that uses black box detection to reconstruct the model or recover training data.
(4) Privacy leak attack:
Data is the core asset used to train the model. Attackers may illegally obtain this data from legitimate connections or malware, resulting in user privacy deprived. And use it to train your own machine learning model to leak the private information of the data set.
Of course, there are many security protection methods, and the following are just a few of them:
(1) Data enhancement
Data enhancement is a common data preprocessing method , which can increase the number and diversity of samples in the data set. This technology can help improve the robustness of the model, making it less susceptible to adversarial sample attacks.
(2) Adversarial training
Adversarial training is also a commonly used method to defend against adversarial sample attacks. It improves the model's resistance to attacks by allowing the model to learn how to resist adversarial sample attacks. Robustness allows the model to better adapt to adversarial examples.
(3) Model distillation
Model distillation technology can convert a complex model into a small model. Because small models are more tolerant of noise and disturbances.
(4) Model integration
Model integration uses multiple different models to make predictions, thereby reducing the risk of adversarial sample attacks.
(5) Data cleaning, filtering, and encryption
Cleaning, filtering, and encryption of data is also a common protection method.
(6) Model monitoring and auditing
Model monitoring and auditing is a method that can identify unusual behaviors in the training process and prediction tasks, thereby helping to detect and repair model vulnerabilities early.
Today, with the rapid development of technology, attackers will use various technical means to carry out attacks, and defenders need more technologies to improve security protection. Therefore, while ensuring data security, we need Continuously learn and adapt to new technologies and methods.
The above is the detailed content of Several attack methods against large AI models. For more information, please follow other related articles on the PHP Chinese website!

Hot AI Tools

Undresser.AI Undress
AI-powered app for creating realistic nude photos

AI Clothes Remover
Online AI tool for removing clothes from photos.

Undress AI Tool
Undress images for free

Clothoff.io
AI clothes remover

AI Hentai Generator
Generate AI Hentai for free.

Hot Article

Hot Tools

Notepad++7.3.1
Easy-to-use and free code editor

SublimeText3 Chinese version
Chinese version, very easy to use

Zend Studio 13.0.1
Powerful PHP integrated development environment

Dreamweaver CS6
Visual web development tools

SublimeText3 Mac version
God-level code editing software (SublimeText3)

Hot Topics
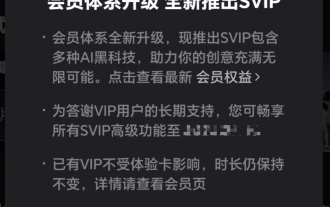
This site reported on June 27 that Jianying is a video editing software developed by FaceMeng Technology, a subsidiary of ByteDance. It relies on the Douyin platform and basically produces short video content for users of the platform. It is compatible with iOS, Android, and Windows. , MacOS and other operating systems. Jianying officially announced the upgrade of its membership system and launched a new SVIP, which includes a variety of AI black technologies, such as intelligent translation, intelligent highlighting, intelligent packaging, digital human synthesis, etc. In terms of price, the monthly fee for clipping SVIP is 79 yuan, the annual fee is 599 yuan (note on this site: equivalent to 49.9 yuan per month), the continuous monthly subscription is 59 yuan per month, and the continuous annual subscription is 499 yuan per year (equivalent to 41.6 yuan per month) . In addition, the cut official also stated that in order to improve the user experience, those who have subscribed to the original VIP
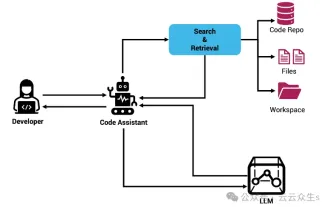
Improve developer productivity, efficiency, and accuracy by incorporating retrieval-enhanced generation and semantic memory into AI coding assistants. Translated from EnhancingAICodingAssistantswithContextUsingRAGandSEM-RAG, author JanakiramMSV. While basic AI programming assistants are naturally helpful, they often fail to provide the most relevant and correct code suggestions because they rely on a general understanding of the software language and the most common patterns of writing software. The code generated by these coding assistants is suitable for solving the problems they are responsible for solving, but often does not conform to the coding standards, conventions and styles of the individual teams. This often results in suggestions that need to be modified or refined in order for the code to be accepted into the application

To learn more about AIGC, please visit: 51CTOAI.x Community https://www.51cto.com/aigc/Translator|Jingyan Reviewer|Chonglou is different from the traditional question bank that can be seen everywhere on the Internet. These questions It requires thinking outside the box. Large Language Models (LLMs) are increasingly important in the fields of data science, generative artificial intelligence (GenAI), and artificial intelligence. These complex algorithms enhance human skills and drive efficiency and innovation in many industries, becoming the key for companies to remain competitive. LLM has a wide range of applications. It can be used in fields such as natural language processing, text generation, speech recognition and recommendation systems. By learning from large amounts of data, LLM is able to generate text
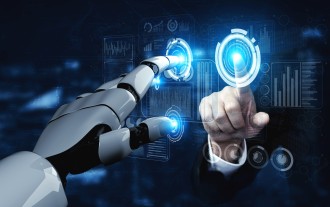
Large Language Models (LLMs) are trained on huge text databases, where they acquire large amounts of real-world knowledge. This knowledge is embedded into their parameters and can then be used when needed. The knowledge of these models is "reified" at the end of training. At the end of pre-training, the model actually stops learning. Align or fine-tune the model to learn how to leverage this knowledge and respond more naturally to user questions. But sometimes model knowledge is not enough, and although the model can access external content through RAG, it is considered beneficial to adapt the model to new domains through fine-tuning. This fine-tuning is performed using input from human annotators or other LLM creations, where the model encounters additional real-world knowledge and integrates it
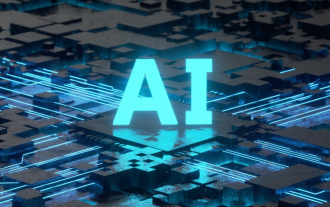
Editor |ScienceAI Question Answering (QA) data set plays a vital role in promoting natural language processing (NLP) research. High-quality QA data sets can not only be used to fine-tune models, but also effectively evaluate the capabilities of large language models (LLM), especially the ability to understand and reason about scientific knowledge. Although there are currently many scientific QA data sets covering medicine, chemistry, biology and other fields, these data sets still have some shortcomings. First, the data form is relatively simple, most of which are multiple-choice questions. They are easy to evaluate, but limit the model's answer selection range and cannot fully test the model's ability to answer scientific questions. In contrast, open-ended Q&A
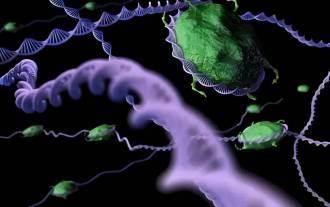
Editor | KX In the field of drug research and development, accurately and effectively predicting the binding affinity of proteins and ligands is crucial for drug screening and optimization. However, current studies do not take into account the important role of molecular surface information in protein-ligand interactions. Based on this, researchers from Xiamen University proposed a novel multi-modal feature extraction (MFE) framework, which for the first time combines information on protein surface, 3D structure and sequence, and uses a cross-attention mechanism to compare different modalities. feature alignment. Experimental results demonstrate that this method achieves state-of-the-art performance in predicting protein-ligand binding affinities. Furthermore, ablation studies demonstrate the effectiveness and necessity of protein surface information and multimodal feature alignment within this framework. Related research begins with "S
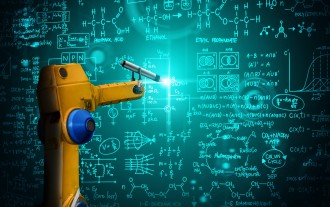
Machine learning is an important branch of artificial intelligence that gives computers the ability to learn from data and improve their capabilities without being explicitly programmed. Machine learning has a wide range of applications in various fields, from image recognition and natural language processing to recommendation systems and fraud detection, and it is changing the way we live. There are many different methods and theories in the field of machine learning, among which the five most influential methods are called the "Five Schools of Machine Learning". The five major schools are the symbolic school, the connectionist school, the evolutionary school, the Bayesian school and the analogy school. 1. Symbolism, also known as symbolism, emphasizes the use of symbols for logical reasoning and expression of knowledge. This school of thought believes that learning is a process of reverse deduction, through existing
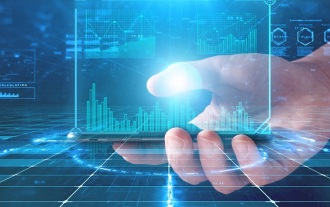
In the world of front-end development, VSCode has become the tool of choice for countless developers with its powerful functions and rich plug-in ecosystem. In recent years, with the rapid development of artificial intelligence technology, AI code assistants on VSCode have sprung up, greatly improving developers' coding efficiency. AI code assistants on VSCode have sprung up like mushrooms after a rain, greatly improving developers' coding efficiency. It uses artificial intelligence technology to intelligently analyze code and provide precise code completion, automatic error correction, grammar checking and other functions, which greatly reduces developers' errors and tedious manual work during the coding process. Today, I will recommend 12 VSCode front-end development AI code assistants to help you in your programming journey.
