How to use artificial intelligence to improve work efficiency
Productivity has always been a major focus for individuals and organizations, and with the advent of artificial intelligence, the rules of the game are changing. This guide explores how to leverage AI tools and technology to increase productivity, optimize workflows, and streamline communications. You can expect to find insights into different types of AI technologies, including machine learning, natural language processing, and computer vision, and their applications in productivity.
Identifying Productivity Gaps
Before deeply deploying artificial intelligence technology to improve productivity, a thorough assessment must be conducted to identify specific areas that require improvement. This initial step requires a rigorous data collection process to gain insight into all aspects of operational processes. You'll want to analyze workflow efficiency, identify potential bottlenecks that could impact performance, and scrutinize tasks that are repetitive and might benefit from automation. By collecting this multifaceted data, you not only gain a comprehensive understanding of your current productivity state, but you also build a strong evidence base. This data-driven approach will allow you to more precisely tailor your AI solutions, ensuring they meet your unique challenges and goals in the most effective way.
Types of AI technologies that improve productivity
Natural Language Processing (NLP): This subset of artificial intelligence focuses on the interaction between computers and human language. Wide range of applications. NLP technology powers chatbots that can handle customer service inquiries, enabling highly accurate transcription services, converting spoken words into written text, and facilitating real-time language translation solutions. These capabilities are invaluable for automating communication processes, reducing human error in transcription, and breaking down language barriers in global organizations.
Machine LearningAlgorithms: These are specialized computational algorithms that allow a system to learn from data and make decisions or predictions. In the context of productivity, machine learning algorithms are widely deployed in various forms of data analysis, from identifying trends in large data sets to predictive analytics that can predict future outcomes. They are also critical for automating complex decision-making processes, reducing the time and resources required for manual assessment.
Computer Vision: This technology enables machines to interpret and act on visual information from the world, replicating the capabilities of human vision but often exceeding it in speed and accuracy. In the area of productivity, computer vision applications are particularly useful for tasks involving image recognition, such as automated quality inspections in production lines or barcode scanning in retail environments. Additionally, they can be used to automate manual inspection processes in industries such as construction and agriculture, freeing up human resources to perform more complex tasks.
AI Tools and Technologies to Improve Productivity
-
Automate Repetitive Tasks
- Robotic Process Automation (RPA): The Technology is specifically designed to automate rule-based and repetitive tasks, essentially acting as a digital workforce. It seamlessly handles chores like data entry, extracting information from documents, and organizing emails. This enables human employees to focus on more detailed and creative tasks, thereby adding higher value to the organization.
- Natural Language Bots: These bots employ natural language processing to perform a variety of tasks that typically require human interaction. They can manage customer service inquiries, autoresponders, and even organize your schedule by integrating with your calendar. These robots are particularly useful for handling routine but essential tasks, freeing up time for more complex activities.
-
Data Analysis and Decision-Making
- Predictive Analytics: Leveraging machine learning algorithms, Predictive AnalyticsScreen large data sets to identify Patterns and trends. These insights are invaluable for making informed, data-driven decisions and strategic initiatives. By predicting potential future events or behaviors, organizations can optimize operations and identify opportunities or risks.
- Recommendation Systems: These algorithms are designed to personalize user experience on various digital platforms, such as mobile apps and e-commerce websites. By analyzing user behavior and preferences, they recommend products, services or content, thereby increasing user engagement and potential revenue streams.
-
Enhanced Communication
- Smart Reply Features: These features are integrated into email services and instant messaging platforms, using Natural language processing to analyze incoming messages and suggest contextually appropriate responses. By doing this, they significantly reduce the time required to respond to letters, making communication quick and efficient.
- LanguageTranslation Tool: In today’s globalized work environment, language is often a barrier to effective communication. Translation tools powered by artificial intelligence can not only translate text, but also translate text in real time, promoting smoother interaction and collaboration between different language backgrounds.
-
Knowledge Management
- Document Classification: AI algorithm can automatically sort and classify incoming documents or information and management. Whether it's invoices, emails, or other forms of content, these algorithms organize the data in a way that makes retrieval and use more efficient.
- Information Retrieval: Leveraging natural language processing, AI-driven search capabilities can scan huge databases or document collections to retrieve relevant information. Unlike simple keyword searches, these systems understand the context and can provide results that are more relevant to the user's actual needs.
Implementing AI Solutions
Feasibility Study: Before implementing any AI solution, conduct a comprehensive Feasibility Study Crucially, the study delves into the expected return on investment (ROI) and technical prerequisites required for successful deployment. This involves a detailed cost-benefit analysis that considers not only upfront and operating costs, but also long-term gains in efficiency and productivity. The technology assessment should review hardware and software requirements, as well as the skills needed to effectively manage and maintain the AI solution.
Select tools: After identifying productivity gaps and assessing feasibility, the next step involves carefully selecting the AI tools that are best suited to address these specific challenges. This requires comparing various platforms and technologies to assess their functionality, scalability, and compatibility with existing systems. The goal is to choose tools that not only solve the immediate problem, but also adapt to changing needs.
Deployment: The deployment phase involves integrating the selected AI tool into the existing technology framework. This is a multi-step process that may include customizing tools to meet unique organizational needs, setting up the necessary infrastructure, and training employees for optimal utilization. A phased rollout strategy must be developed, starting with a pilot program to validate the effectiveness of the solution before full implementation.
Monitoring and Tuning: Once an AI system is operational, ongoing monitoring is critical to tracking its effectiveness in real time. This includes regular assessments using predefined performance indicators and potentially using other artificial intelligence or analytics tools for deeper analysis. Based on these assessments, adjustments may need to be made – whether that’s fine-tuning the algorithm, scaling the solution, or reverting to an alternative tool if the existing tool doesn’t live up to expectations.
The above is the detailed content of How to use artificial intelligence to improve work efficiency. For more information, please follow other related articles on the PHP Chinese website!

Hot AI Tools

Undresser.AI Undress
AI-powered app for creating realistic nude photos

AI Clothes Remover
Online AI tool for removing clothes from photos.

Undress AI Tool
Undress images for free

Clothoff.io
AI clothes remover

AI Hentai Generator
Generate AI Hentai for free.

Hot Article

Hot Tools

Notepad++7.3.1
Easy-to-use and free code editor

SublimeText3 Chinese version
Chinese version, very easy to use

Zend Studio 13.0.1
Powerful PHP integrated development environment

Dreamweaver CS6
Visual web development tools

SublimeText3 Mac version
God-level code editing software (SublimeText3)

Hot Topics


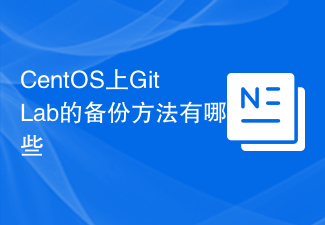
Backup and Recovery Policy of GitLab under CentOS System In order to ensure data security and recoverability, GitLab on CentOS provides a variety of backup methods. This article will introduce several common backup methods, configuration parameters and recovery processes in detail to help you establish a complete GitLab backup and recovery strategy. 1. Manual backup Use the gitlab-rakegitlab:backup:create command to execute manual backup. This command backs up key information such as GitLab repository, database, users, user groups, keys, and permissions. The default backup file is stored in the /var/opt/gitlab/backups directory. You can modify /etc/gitlab
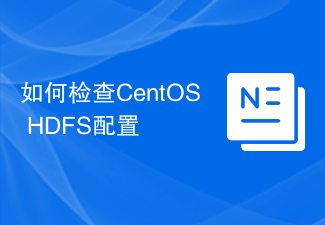
Complete Guide to Checking HDFS Configuration in CentOS Systems This article will guide you how to effectively check the configuration and running status of HDFS on CentOS systems. The following steps will help you fully understand the setup and operation of HDFS. Verify Hadoop environment variable: First, make sure the Hadoop environment variable is set correctly. In the terminal, execute the following command to verify that Hadoop is installed and configured correctly: hadoopversion Check HDFS configuration file: The core configuration file of HDFS is located in the /etc/hadoop/conf/ directory, where core-site.xml and hdfs-site.xml are crucial. use
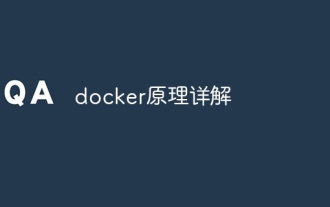
Docker uses Linux kernel features to provide an efficient and isolated application running environment. Its working principle is as follows: 1. The mirror is used as a read-only template, which contains everything you need to run the application; 2. The Union File System (UnionFS) stacks multiple file systems, only storing the differences, saving space and speeding up; 3. The daemon manages the mirrors and containers, and the client uses them for interaction; 4. Namespaces and cgroups implement container isolation and resource limitations; 5. Multiple network modes support container interconnection. Only by understanding these core concepts can you better utilize Docker.
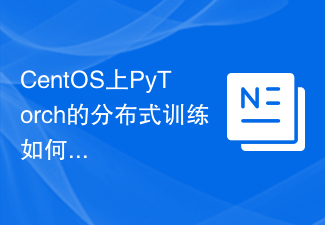
PyTorch distributed training on CentOS system requires the following steps: PyTorch installation: The premise is that Python and pip are installed in CentOS system. Depending on your CUDA version, get the appropriate installation command from the PyTorch official website. For CPU-only training, you can use the following command: pipinstalltorchtorchvisiontorchaudio If you need GPU support, make sure that the corresponding version of CUDA and cuDNN are installed and use the corresponding PyTorch version for installation. Distributed environment configuration: Distributed training usually requires multiple machines or single-machine multiple GPUs. Place
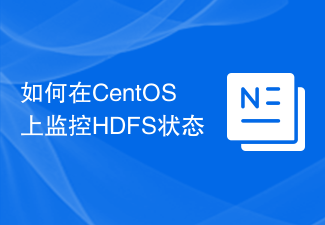
There are many ways to monitor the status of HDFS (Hadoop Distributed File System) on CentOS systems. This article will introduce several commonly used methods to help you choose the most suitable solution. 1. Use Hadoop’s own WebUI, Hadoop’s own Web interface to provide cluster status monitoring function. Steps: Make sure the Hadoop cluster is up and running. Access the WebUI: Enter http://:50070 (Hadoop2.x) or http://:9870 (Hadoop3.x) in your browser. The default username and password are usually hdfs/hdfs. 2. Command line tool monitoring Hadoop provides a series of command line tools to facilitate monitoring
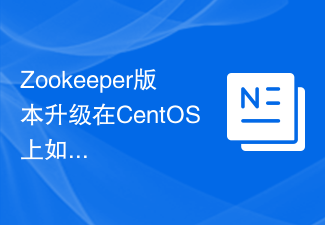
ZooKeeper version upgrade guide under CentOS system, be sure to be fully prepared before upgrading ZooKeeper version. The following steps will guide you through the upgrade of ZooKeeper version on CentOS: Backup the existing version: Before starting the upgrade, please back up the current ZooKeeper data directory and configuration files in case of accidents. Download the new version: Download the target version from the official ApacheZooKeeper website. Unzip the new version: Unzip the downloaded ZooKeeper compressed package to the directory of your choice. Configure the new version: Copy the zoo_sample.cfg file and rename it to zoo.cfg. Edit z
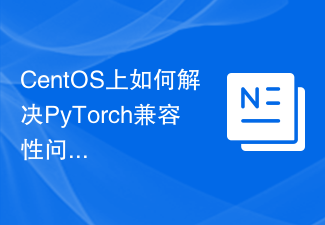
Installing and configuring PyTorch on CentOS system and making full use of GPU to accelerate deep learning tasks, you can follow the following steps: Step 1: Installing Anaconda3 First, use Anaconda3 as a Python environment management tool to facilitate the installation and management of PyTorch and its dependent libraries. Download the Anaconda3 installation script and execute: wgethttps://repo.anaconda.com/archive/Anaconda3-2024.05-Linux-x86_64.shbashAnaconda3-2024.05-Linux-x86_64.shStep 2: Create virtual
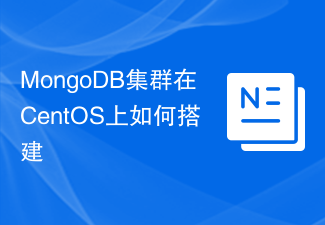
To build a MongoDB cluster on the CentOS system, you need to complete MongoDB installation, instance configuration, replica set settings, and sharding steps. The following steps will guide you through this process in detail: 1. Preparation work to ensure that the CentOS system has been updated and install the necessary tools: sudoyumupdate-ysudoyuminstall-ywgetvim 2. Install MongoDB Add MongoDBYUM source: Create mongodb.repo file and add MongoDB repository information (please adjust the version number according to the actual situation): echo"[mongodb-org-4.4]n