


Enhancing instant insights: The synergy of computer vision and edge computing
In today’s fast-paced world, the seamless integration of cutting-edge technologies has become the cornerstone of innovation.
Rewritten content: Across industries, computer vision and edge computing stand out as two key pillars. Computer vision is a technology driven by artificial intelligence that enables machines to interpret, analyze and understand visual information from the world. Edge computing supports real-time data processing and analysis at the edge of the network, getting closer to the data source, reducing delays and improving efficiency
Benefits of integrating computer vision and edge computing
Computer vision and edge computing Integration opens up a new realm of possibilities, especially in areas where real-time data analysis and low latency are critical. By bringing intelligence closer to the data source, businesses can now make faster, more informed decisions. This synergy is revolutionizing the following areas:
1. Intelligent surveillance systems
Traditional surveillance systems are rapidly being replaced by intelligent and proactive solutions driven by computer vision and edge computing replaced. These solutions are capable of processing and analyzing video from multiple cameras in real time, detecting anomalies, predicting potential threats, and promptly alerting authorities. Therefore, security personnel can respond to incidents more efficiently and improve the safety of public places
2. Industrial automation
By integrating computer vision and edge computing, the level of industrial automation has been greatly improved. In manufacturing units, cameras installed next to production lines can accurately identify defective products. By analyzing edge data, the system can take immediate corrective action to prevent defective products from spreading further in the production process. This optimization minimizes downtime, reduces waste, and improves overall productivity Understand customer behavior and preferences. Smart cameras strategically placed inside stores can analyze shoppers’ movements, product interactions, and even facial expressions while protecting data privacy. This data-driven approach can help retailers optimize store layouts, provide personalized recommendations, and ultimately improve the overall shopping experience
4. Self-driving cars
With the emergence of self-driving cars, The automotive industry is undergoing a transformation. Computer vision algorithms deployed at the edge allow these cars to quickly interpret their surroundings and react accordingly. By processing data from multiple sensors in real time, autonomous vehicles can detect pedestrians, road signs, obstacles and other vehicles to ensure safe and reliable navigation on the road
Challenges and Opportunities of Computer Vision and Edge Computing
Although the synergy of computer vision and edge computing presents great potential, it also brings a series of challenges
1. Bandwidth limitations
Edge devices usually operate under limited bandwidth operating bandwidth, compared to centralized cloud servers. To ensure that the network is not overloaded, computer vision models and data transfer need to be optimized for efficient processing
2, security and privacy
As data is processed closer to the data source Processing, ensuring the security and privacy of sensitive information becomes critical. Strong encryption and authentication mechanisms must be in place to protect data from unauthorized access or tampering
3. Scalability
In terms of deploying, managing and scaling edge devices at scale Complex challenges may be faced. We need to design a flexible architecture that can handle growing computing demands and maintain seamless operations
Summary
It is undeniable that the seamless integration of computer vision and edge computing is reshaping Technology landscape across industries. From real-time surveillance and industrial automation to revolutionary retail experiences and autonomous vehicles, this synergy provides unprecedented opportunities for innovation and growth. As organizations continue to explore this convergence, addressing challenges such as bandwidth constraints, security and scalability will pave the way for a future where actionable insights are instantly available to drive new heights of efficiency, security and productivity
The above is the detailed content of Enhancing instant insights: The synergy of computer vision and edge computing. For more information, please follow other related articles on the PHP Chinese website!

Hot AI Tools

Undresser.AI Undress
AI-powered app for creating realistic nude photos

AI Clothes Remover
Online AI tool for removing clothes from photos.

Undress AI Tool
Undress images for free

Clothoff.io
AI clothes remover

AI Hentai Generator
Generate AI Hentai for free.

Hot Article

Hot Tools

Notepad++7.3.1
Easy-to-use and free code editor

SublimeText3 Chinese version
Chinese version, very easy to use

Zend Studio 13.0.1
Powerful PHP integrated development environment

Dreamweaver CS6
Visual web development tools

SublimeText3 Mac version
God-level code editing software (SublimeText3)

Hot Topics


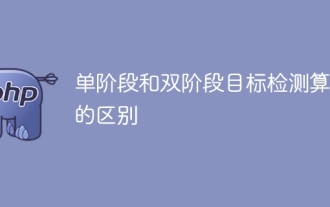
Object detection is an important task in the field of computer vision, used to identify objects in images or videos and locate their locations. This task is usually divided into two categories of algorithms, single-stage and two-stage, which differ in terms of accuracy and robustness. Single-stage target detection algorithm The single-stage target detection algorithm converts target detection into a classification problem. Its advantage is that it is fast and can complete the detection in just one step. However, due to oversimplification, the accuracy is usually not as good as the two-stage object detection algorithm. Common single-stage target detection algorithms include YOLO, SSD and FasterR-CNN. These algorithms generally take the entire image as input and run a classifier to identify the target object. Unlike traditional two-stage target detection algorithms, they do not need to define areas in advance, but directly predict
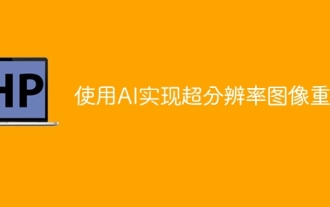
Super-resolution image reconstruction is the process of generating high-resolution images from low-resolution images using deep learning techniques, such as convolutional neural networks (CNN) and generative adversarial networks (GAN). The goal of this method is to improve the quality and detail of images by converting low-resolution images into high-resolution images. This technology has wide applications in many fields, such as medical imaging, surveillance cameras, satellite images, etc. Through super-resolution image reconstruction, we can obtain clearer and more detailed images, which helps to more accurately analyze and identify targets and features in images. Reconstruction methods Super-resolution image reconstruction methods can generally be divided into two categories: interpolation-based methods and deep learning-based methods. 1) Interpolation-based method Super-resolution image reconstruction based on interpolation
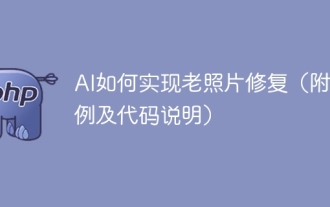
Old photo restoration is a method of using artificial intelligence technology to repair, enhance and improve old photos. Using computer vision and machine learning algorithms, the technology can automatically identify and repair damage and flaws in old photos, making them look clearer, more natural and more realistic. The technical principles of old photo restoration mainly include the following aspects: 1. Image denoising and enhancement. When restoring old photos, they need to be denoised and enhanced first. Image processing algorithms and filters, such as mean filtering, Gaussian filtering, bilateral filtering, etc., can be used to solve noise and color spots problems, thereby improving the quality of photos. 2. Image restoration and repair In old photos, there may be some defects and damage, such as scratches, cracks, fading, etc. These problems can be solved by image restoration and repair algorithms
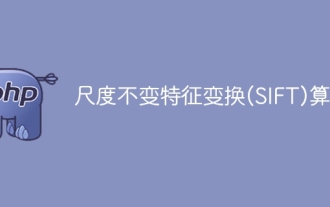
The Scale Invariant Feature Transform (SIFT) algorithm is a feature extraction algorithm used in the fields of image processing and computer vision. This algorithm was proposed in 1999 to improve object recognition and matching performance in computer vision systems. The SIFT algorithm is robust and accurate and is widely used in image recognition, three-dimensional reconstruction, target detection, video tracking and other fields. It achieves scale invariance by detecting key points in multiple scale spaces and extracting local feature descriptors around the key points. The main steps of the SIFT algorithm include scale space construction, key point detection, key point positioning, direction assignment and feature descriptor generation. Through these steps, the SIFT algorithm can extract robust and unique features, thereby achieving efficient image processing.
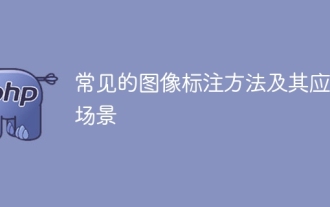
In the fields of machine learning and computer vision, image annotation is the process of applying human annotations to image data sets. Image annotation methods can be mainly divided into two categories: manual annotation and automatic annotation. Manual annotation means that human annotators annotate images through manual operations. This method requires human annotators to have professional knowledge and experience and be able to accurately identify and annotate target objects, scenes, or features in images. The advantage of manual annotation is that the annotation results are reliable and accurate, but the disadvantage is that it is time-consuming and costly. Automatic annotation refers to the method of using computer programs to automatically annotate images. This method uses machine learning and computer vision technology to achieve automatic annotation by training models. The advantages of automatic labeling are fast speed and low cost, but the disadvantage is that the labeling results may not be accurate.
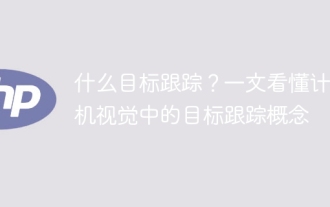
Object tracking is an important task in computer vision and is widely used in traffic monitoring, robotics, medical imaging, automatic vehicle tracking and other fields. It uses deep learning methods to predict or estimate the position of the target object in each consecutive frame in the video after determining the initial position of the target object. Object tracking has a wide range of applications in real life and is of great significance in the field of computer vision. Object tracking usually involves the process of object detection. The following is a brief overview of the object tracking steps: 1. Object detection, where the algorithm classifies and detects objects by creating bounding boxes around them. 2. Assign a unique identification (ID) to each object. 3. Track the movement of detected objects in frames while storing relevant information. Types of Target Tracking Targets
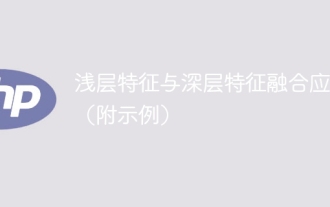
Deep learning has achieved great success in the field of computer vision, and one of the important advances is the use of deep convolutional neural networks (CNN) for image classification. However, deep CNNs usually require large amounts of labeled data and computing resources. In order to reduce the demand for computational resources and labeled data, researchers began to study how to fuse shallow features and deep features to improve image classification performance. This fusion method can take advantage of the high computational efficiency of shallow features and the strong representation ability of deep features. By combining the two, computational costs and data labeling requirements can be reduced while maintaining high classification accuracy. This method is particularly important for application scenarios where the amount of data is small or computing resources are limited. By in-depth study of the fusion methods of shallow features and deep features, we can further
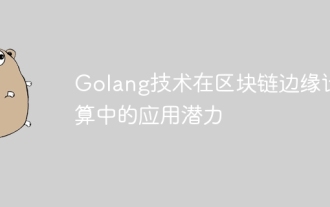
The Go language is ideal for developing blockchain edge computing applications because of its concurrency, high performance, and rich ecosystem. Use cases include smart contract execution, data collection and analysis, and identity verification. Go code examples demonstrate executing smart contracts and collecting and analyzing data on edge devices.
