


How to conduct user purchasing behavior analysis and personalized recommendations for PHP flash sale system
How to conduct user purchasing behavior analysis and personalized recommendations of the PHP flash sale system requires specific code examples
With the in-depth development of the Internet, competition in the e-commerce field has changed. It's getting more and more intense. In order to attract users and encourage them to purchase, e-commerce platforms often launch flash sales activities. However, it is not easy for users to choose and purchase products that suit them. Therefore, it is very important to analyze users' purchasing behavior and recommend personalized products for them.
In the PHP flash sale system, we can conduct user purchasing behavior analysis and personalized recommendations through the following steps:
- Data collection: First, we need to collect data on user behavior collection. It can record the user's browsing, collection, adding shopping cart and purchasing behaviors in the system, as well as the user's personal information and preferences.
- Data storage: Store the collected data in the database. Relational databases such as MySQL can be used to store user data.
- Data analysis: Through data analysis, we can understand users’ purchasing habits, preferences and potential needs. By analyzing the user's purchase frequency, purchase time, purchase amount and other indicators, the user's purchasing behavior pattern can be derived. In addition, the user's preference characteristics can also be obtained by analyzing the user's historical purchase records and personal information, such as the user's preference for brand, color, size, etc.
- Recommendation algorithm: Based on the user's purchasing behavior and personal preferences, we can use the recommendation algorithm to recommend personalized products to the user. Commonly used recommendation algorithms include collaborative filtering recommendation algorithms, content-based recommendation algorithms and hybrid recommendation algorithms. The following takes the collaborative filtering recommendation algorithm as an example to introduce the specific implementation method.
The implementation steps of the collaborative filtering recommendation algorithm are as follows:
Step 1: Calculate the similarity between users. A user-based collaborative filtering algorithm can be used to measure the correlation between users by calculating the similarity matrix between users.
Step 2: Recommend products to users. When a user browses, collects, adds to a shopping cart, or purchases products, products that similar users like can be recommended to the user based on the user's purchasing behavior and the purchasing behavior of similar users.
The following is a simple sample code that demonstrates how to implement a user-based collaborative filtering recommendation algorithm:
// 获取用户ID $userID = $_SESSION['userID']; // 从数据库中获取用户与商品的交互行为数据 $interactions = fetch_interactions($userID); // 计算用户之间的相似度矩阵 $similarityMatrix = calculate_similarity_matrix($interactions); // 为用户推荐商品 $recommendedItems = recommend_items($similarityMatrix, $userID); // 展示推荐的商品 foreach ($recommendedItems as $itemID) { $item = fetch_item($itemID); echo "商品名称:{$item['name']}, 价格:{$item['price']}"; } // 从数据库中获取用户与商品的交互行为数据 function fetch_interactions($userID) { // 查询数据库获取用户与商品的交互行为数据 // 返回用户与商品的交互行为数据数组,数组的每个元素包含用户ID、商品ID和操作类型(浏览、收藏、加购物车、购买等) } // 计算用户之间的相似度矩阵 function calculate_similarity_matrix($interactions) { // 根据用户与商品的交互行为数据计算用户之间的相似度矩阵 // 返回用户之间的相似度矩阵 } // 为用户推荐商品 function recommend_items($similarityMatrix, $userID) { // 根据用户之间的相似度矩阵和用户ID推荐商品 // 返回推荐的商品ID数组 } // 从数据库中获取商品信息 function fetch_item($itemID) { // 查询数据库获取商品信息 // 返回商品信息数组,数组的每个元素包含商品ID、商品名称和商品价格等信息 }
Through the above code example, we can implement a user-based collaborative filtering recommendation algorithm and based on The user's purchasing behavior and the purchasing behavior of similar users recommend personalized products to the user.
To sum up, by analyzing the user’s purchasing behavior and using the recommendation algorithm to recommend personalized products to the user, the user’s purchasing experience can be improved and the user’s purchasing rate can be increased. For the PHP flash sale system, purchasing behavior analysis and personalized recommendations are very important functions, which can help the platform attract more users and improve user purchase satisfaction.
The above is the detailed content of How to conduct user purchasing behavior analysis and personalized recommendations for PHP flash sale system. For more information, please follow other related articles on the PHP Chinese website!

Hot AI Tools

Undresser.AI Undress
AI-powered app for creating realistic nude photos

AI Clothes Remover
Online AI tool for removing clothes from photos.

Undress AI Tool
Undress images for free

Clothoff.io
AI clothes remover

AI Hentai Generator
Generate AI Hentai for free.

Hot Article

Hot Tools

Notepad++7.3.1
Easy-to-use and free code editor

SublimeText3 Chinese version
Chinese version, very easy to use

Zend Studio 13.0.1
Powerful PHP integrated development environment

Dreamweaver CS6
Visual web development tools

SublimeText3 Mac version
God-level code editing software (SublimeText3)

Hot Topics


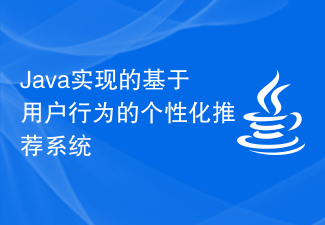
With the development of Internet technology and the era of information explosion, how to find content that meets one's needs from massive data has become a topic of public concern. The personalized recommendation system exudes endless light at this time. This article will introduce a personalized recommendation system based on user behavior implemented in Java. 1. Introduction to the Personalized Recommendation System The personalized recommendation system provides users with personalized recommendation services based on the user’s historical behavior, preferences, as well as multi-dimensional related factors such as item information, time and space in the system. Through a personalized recommendation system,
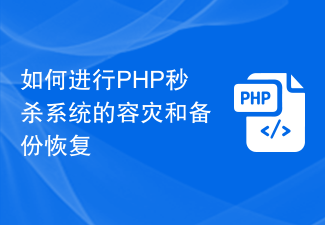
How to perform disaster recovery and backup recovery of PHP flash sale system 1. Background introduction With the rise of e-commerce and the advancement of Internet technology, flash sale activities are widely used in the e-commerce industry. However, in flash sale activities where a large number of users participate at the same time, system disaster recovery and backup and recovery have become important links to ensure user experience. This article will introduce how to use PHP to implement disaster recovery and backup recovery of the flash sale system, and provide relevant code examples. 2. Distributed architecture of disaster recovery design: split the system into multiple subsystems, and each subsystem is independently deployed on a different server and interacts with each other.
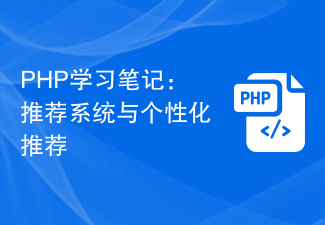
PHP study notes: Recommendation system and personalized recommendations, specific code examples are required Introduction: In today's Internet era, recommendation systems have become one of the important functions of many websites and applications. By using machine learning and data mining technologies, recommendation systems can recommend the most relevant content and products to users based on their behavior and interests, improving user experience and website interactivity. Personalized recommendation is an important algorithm of the recommendation system, which can customize personalized recommendation results based on the user's preferences and historical behavior. The basic principles of recommendation system
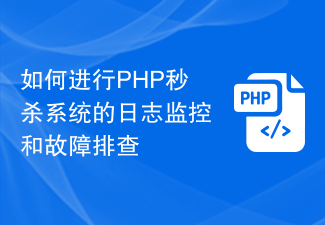
How to carry out log monitoring and troubleshooting of PHP flash sale system Introduction: With the rapid development of the e-commerce industry, flash sale activities have become an important way to attract consumers. In flash sale activities, system stability and high concurrency processing capabilities are crucial. In order to ensure the normal operation of the flash sale system, log monitoring and troubleshooting are required. This article will introduce how to use PHP for log monitoring and troubleshooting of the flash sale system, and provide some code examples. 1. Log monitoring setting log level In the configuration file of the flash sale system, we can set
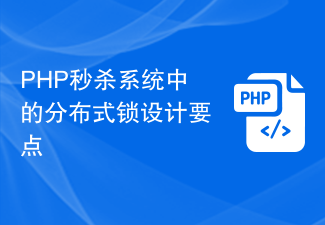
Key points of distributed lock design in PHP flash sale system With the development of the Internet, rush buying activities on e-commerce platforms are becoming more and more common. In high-concurrency scenarios, the implementation of flash sale activities faces many challenges. One of them is how to ensure that each user can only purchase once before the product is sold out. To solve this problem, distributed locks have become a common solution. In PHP development, we can implement distributed locks through the following design points. 1. Choose appropriate storage media and technology. Before choosing a distributed lock implementation solution, we need to
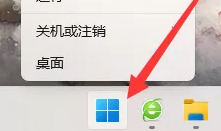
How to turn off personalized recommendations in win11? Users can directly select Settings under the Start menu, then select the Personalization option on the window that opens, and then click the Start option on the right to perform the operation. Let this site carefully introduce to users how to turn off Win11 personalized recommendations. How to turn off Windows 11 Personalization Recommendation 1. Right-click Start in the taskbar in the lower left corner. 3. In the window that opens, click the Personalization option in the left column. 5. Finally, turn off the switch buttons on the right side of Show Recently Added Applications and Show Most Commonly Used Applications.
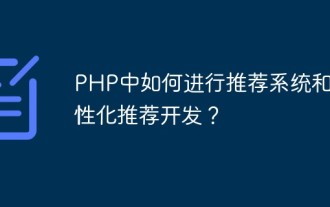
With the continuous development of e-commerce and social media, recommendation systems and personalized recommendations have attracted more and more attention. They have played an important role in improving user experience and increasing user retention. So how to develop recommendation systems and personalized recommendations in PHP? here we come to find out. The concept of recommendation system and personalized recommendation A recommendation system is a system that analyzes user behavior, interests, needs and other information to mine content or products that users may be interested in from massive data and make personalized recommendations. Recommendation systems can roughly
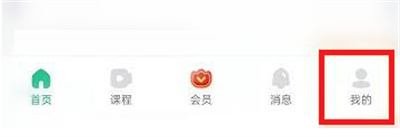
When we use Baidu Wenku, we can set up personalized recommendation content. Here we will introduce the operation method. Interested friends can take a look with me. 1. Click to open the Baidu Wenku app on your mobile phone and click "My" in the lower right corner of the page to switch to it. 2. Find the "Settings" function on my page and click to select it. 3. Next, there is a "Privacy Settings" in the settings page you enter. Click on it when you see it. 4. Click the "Recommended Settings" item on the privacy settings page to enter. 5. Finally, in the recommended setting interface, you will see a switch button behind "Personalized Recommendation". Click the circular slider on it and set it to green to turn it on. The software will be based on our interests and hobbies.
