How to develop distributed graph computing functions using Redis and R language
How to use Redis and R language to develop distributed graph computing functions
Introduction:
As the scale of data continues to increase, traditional data processing methods have Unable to meet demand. Distributed graph computing has become an effective way to process large-scale data. This article will introduce how to use Redis and R language to develop distributed graph computing functions, and give specific code examples.
1. What is distributed graph computing
Distributed graph computing refers to dividing a large-scale graph into multiple subgraphs and then assigning them to different computing nodes for parallel computing. This method can greatly reduce the time of graph calculation and can cope with the processing needs of big data.
2. Basic concepts of Redis
Redis is a high-performance in-memory database, often used in caching and distributed computing. The following are some basic concepts of Redis:
- Key-Value storage: Redis uses key-value pairs to store data, and can quickly locate values based on keys.
- Data type: Redis supports multiple data types, such as strings, hash tables, lists, etc.
- Persistence: Redis can persist data to disk to avoid data loss.
- Publish/subscribe mode: Redis can realize the transmission and interaction of information through the publish/subscribe mode.
3. Integration of R language and Redis
R language is a programming language for statistical analysis and data visualization, with rich data analysis libraries and functions. You can use the rredis
package to integrate the R language with Redis. The following are some commonly used Redis operation examples:
- Connect to Redis server
library(rredis) redisConnect(host = "localhost", port = 6379)
- Set key-value pairs
redisSet("name", "Jack")
- Get the value corresponding to the key
redisGet("name")
- Delete the key-value pair
redisDel("name")
4. The basic idea of distributed graph computing
In distributed graph computing , we divide the entire graph into multiple subgraphs and assign them to different computing nodes for calculation. We can use the key-value pair feature of Redis to represent the nodes and edges of the graph. The following are the basic distributed graph calculation steps:
- Split the entire graph into multiple subgraphs and store each subgraph in Redis.
- On each computing node, independently calculate the subgraph assigned to it, and store the calculation results in Redis.
- Continuously iterate the calculation until the final calculation result is obtained.
5. Sample code
The following is a sample code that uses Redis and R language to develop distributed graph calculation functions, which is used to calculate the PageRank value of nodes in the graph.
- Install rredis package
install.packages("rredis")
- Set Redis parameters
library(rredis) redisConnect(host = "localhost", port = 6379)
- Create graph data
nodes <- c("A", "B", "C", "D", "E") edges <- matrix(c("A", "B", "B", "C", "B", "D", "C", "D", "D", "E", "E", "D"), ncol = 2, byrow = TRUE)
- Storing graph data into Redis
redisMSet(nodes, rep(1, length(nodes))) for(i in 1:nrow(edges)) { redisDel(edges[i, 2]) redisLPush(edges[i, 2], edges[i, 1]) }
- Get the PageRank value through iterative calculation
for(i in 1:10) { result <- vector("list", length(nodes)) for(j in 1:length(nodes)) { neighbors <- redisList(nodes[j]) pagerank <- sum(sapply(neighbors, function(x) redisGet(x, type = "numeric"))) result[[j]] <- pagerank } names(result) <- nodes for(j in 1:length(nodes)) { redisSet(nodes[j], result[[j]]) } }
6. Summary
This article It introduces how to use Redis and R language to develop distributed graph computing functions, and gives specific code examples. Through distributed graph computing, the efficiency of large-scale data processing can be improved to meet practical needs. I hope this article can be helpful to readers in their learning and application of distributed graph computing.
The above is the detailed content of How to develop distributed graph computing functions using Redis and R language. For more information, please follow other related articles on the PHP Chinese website!

Hot AI Tools

Undresser.AI Undress
AI-powered app for creating realistic nude photos

AI Clothes Remover
Online AI tool for removing clothes from photos.

Undress AI Tool
Undress images for free

Clothoff.io
AI clothes remover

Video Face Swap
Swap faces in any video effortlessly with our completely free AI face swap tool!

Hot Article

Hot Tools

Notepad++7.3.1
Easy-to-use and free code editor

SublimeText3 Chinese version
Chinese version, very easy to use

Zend Studio 13.0.1
Powerful PHP integrated development environment

Dreamweaver CS6
Visual web development tools

SublimeText3 Mac version
God-level code editing software (SublimeText3)

Hot Topics


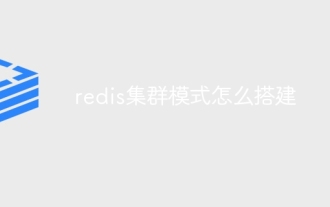
Redis cluster mode deploys Redis instances to multiple servers through sharding, improving scalability and availability. The construction steps are as follows: Create odd Redis instances with different ports; Create 3 sentinel instances, monitor Redis instances and failover; configure sentinel configuration files, add monitoring Redis instance information and failover settings; configure Redis instance configuration files, enable cluster mode and specify the cluster information file path; create nodes.conf file, containing information of each Redis instance; start the cluster, execute the create command to create a cluster and specify the number of replicas; log in to the cluster to execute the CLUSTER INFO command to verify the cluster status; make
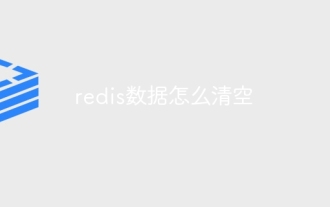
How to clear Redis data: Use the FLUSHALL command to clear all key values. Use the FLUSHDB command to clear the key value of the currently selected database. Use SELECT to switch databases, and then use FLUSHDB to clear multiple databases. Use the DEL command to delete a specific key. Use the redis-cli tool to clear the data.
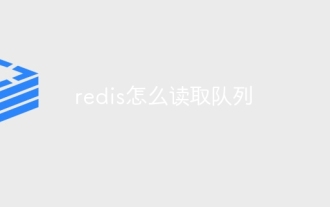
To read a queue from Redis, you need to get the queue name, read the elements using the LPOP command, and process the empty queue. The specific steps are as follows: Get the queue name: name it with the prefix of "queue:" such as "queue:my-queue". Use the LPOP command: Eject the element from the head of the queue and return its value, such as LPOP queue:my-queue. Processing empty queues: If the queue is empty, LPOP returns nil, and you can check whether the queue exists before reading the element.
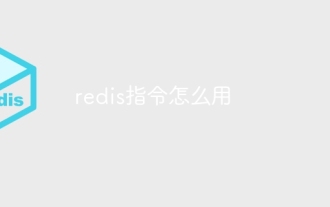
Using the Redis directive requires the following steps: Open the Redis client. Enter the command (verb key value). Provides the required parameters (varies from instruction to instruction). Press Enter to execute the command. Redis returns a response indicating the result of the operation (usually OK or -ERR).
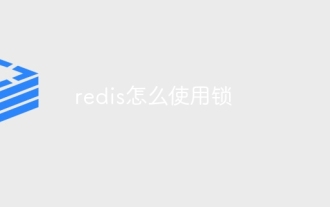
Using Redis to lock operations requires obtaining the lock through the SETNX command, and then using the EXPIRE command to set the expiration time. The specific steps are: (1) Use the SETNX command to try to set a key-value pair; (2) Use the EXPIRE command to set the expiration time for the lock; (3) Use the DEL command to delete the lock when the lock is no longer needed.
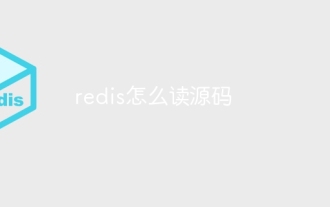
The best way to understand Redis source code is to go step by step: get familiar with the basics of Redis. Select a specific module or function as the starting point. Start with the entry point of the module or function and view the code line by line. View the code through the function call chain. Be familiar with the underlying data structures used by Redis. Identify the algorithm used by Redis.
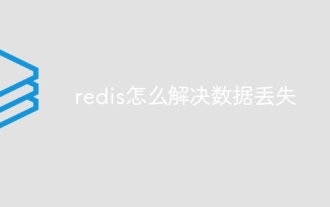
Redis data loss causes include memory failures, power outages, human errors, and hardware failures. The solutions are: 1. Store data to disk with RDB or AOF persistence; 2. Copy to multiple servers for high availability; 3. HA with Redis Sentinel or Redis Cluster; 4. Create snapshots to back up data; 5. Implement best practices such as persistence, replication, snapshots, monitoring, and security measures.
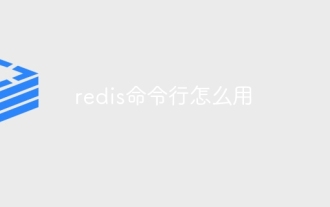
Use the Redis command line tool (redis-cli) to manage and operate Redis through the following steps: Connect to the server, specify the address and port. Send commands to the server using the command name and parameters. Use the HELP command to view help information for a specific command. Use the QUIT command to exit the command line tool.
