How does DSA overtake NVIDIA GPU in a corner?
You may have heard the following sharp opinions:
1. If you follow NVIDIA’s technical route, you may never be able to catch up with NVIDIA.
2. DSA may have a chance to catch up with NVIDIA, but the current situation is that DSA is on the verge of extinction and there is no hope.
On the other hand, we all know that large models are now at the forefront. , many people in the industry want to make large-model chips, and many people want to invest in large-model chips.
But what is the key to the design of large model chips? Everyone seems to know the importance of large bandwidth and large memory, but how is the chip made different from NVIDIA?
With questions, this article tries to give you some inspiration.
Articles that are purely based on opinions often appear formalistic. We can illustrate this through an architectural example
SambaNova Systems is known as one of the top ten unicorn companies in the United States. In April 2021, the company received US$678 million in Series D investment led by SoftBank, with a valuation reaching US$5 billion, making it a super unicorn company. Previously, SambaNova’s investors included the world’s top venture capital funds such as Google Ventures, Intel Capital, SK and Samsung Catalytic Fund. So, what disruptive things is this super unicorn company that has attracted the favor of the world's top investment institutions doing? By observing their early promotional materials, we can find that SambaNova has chosen a different development path from the AI giant NVIDIA
Is it a bit shocking? A 1024 V100 cluster built with unprecedented power on the NVIDIA platform is actually equivalent to a single machine from SambaNova? ! This is the first generation product, a stand-alone 8-card machine based on SN10 RDU.
Some people may say that this comparison is unfair. Doesn’t NVIDIA have DGX A100? Maybe SambaNova itself has realized it, and the second-generation product SN30 has been changed to this:
DGX A100 has a computing power of 5 petaFLOPS, and SambaNova’s second-generation DataScale also has a computing power of 5 petaFLOPS. Memory comparison of 320GB HBM vs 8TB DDR4 (the editor guesses that he may have written the article wrong, it should be 3TB * 8).
The second generation chip is actually the Die-to-Die version of the SN10 RDU. The architectural indicators of SN10 RDU are: 320TFLOPS@BF16, 320M SRAM, 1.5T DDR4. The SN30 RDU is doubled on this basis, as described below:
“This chip had 640 pattern compute units with more than 320 teraflops of compute at BF16 floating point precision and also had 640 pattern memory units with 320 MB of on-chip SRAM and 150 TB/sec of on-chip memory bandwidth. Each SN10 processor was also able to address 1.5 TB of DDR4 auxiliary memory." "With the Cardinal SN30 RDU, the capacity of the RDU is doubled, and the reason it is doubled is that SambaNova designed its architecture to make use of multi-die packaging from the get-go, and in this case SambaNova is doubling up the capacity of its DataScale machines by cramming two new RDU – what we surmise are two tweaked SN10s with microarchitectures changes to better support large foundation models – into a single complex called the SN30. Each socket in a DataScale system now has twice the compute capacity, twice the local memory capacity, and twice the memory bandwidth of the first generations of machines."
Key points extraction:There is only one choice between large bandwidth and large capacity. NVIDIA chose large bandwidth HBM, while SambaNova chose Large capacity DDR4. In terms of performance results, SambaNova wins.
If you switch to DGX H100, even if you switch to low-precision technologies such as FP8, you can only narrow the gap.
“And even if the DGX-H100 offers 3X the performance at 16-bit floating point calculation than the DGX-A100, it will not close the gap with the SambaNova system. However, with lower precision FP8 data, Nvidia might be able to close the performance gap; it is unclear how much precision will be sacrificed by shifting to lower precision data and processing."
If someone can achieve such an effect, wouldn't it be a perfect large-scale Chip solution? And it can also directly face the competition from NVIDIA!
(Maybe you will say that Grace CPU can also be connected to LPDDR, which is helpful for increasing capacity. On the other hand, how does SambaNova view this matter: Grace is just a large memory controller, but it can only Hopper brings 512GB of DRAM, while an SN30 has 3TB of DRAM.
We used to joke that Nvidia's "Grace" Arm CPU was just an overhyped memory controller for a Hopper GPU. In many cases, It's really just a memory controller, and each Hopper GPU in the Grace-Hopper super chip set only has up to 512GB of memory. That's still a lot less than the 3TB of memory the SambaNova offers per socket
History tells us that no matter how prosperous the empire is, it may still have to be careful of that inconspicuous crack!
Xia He, the master of Huawei, recently speculated that a weakness of the NVIDIA empire may lie in the cost per GB from a cost perspective. He suggested crazy stacking of cheap DDR memory for large-scale internal input/output. This may have a revolutionary impact on NVIDIA
(Extension:https://www.php.cn/link/617974172720b96de92525536de581fa)
Mackler, another Zhihu master who studies DSA, gave his opinion. From the perspective of $/GBps (data migration), HBM is more cost-effective, because although LLM has a relatively large demand for memory capacity, it has There is also a huge demand for bandwidth, and training requires a large number of parameters that need to be exchanged in DRAM.
(Extension:https://www.php.cn/link/a56ee48e5c142c26cf645b2cc23d78fc)
From the SambaNova architecture example Look, large-capacity and cheap DDR can solve the problem of LLM, which confirms Xia Core’s judgment! But the huge bandwidth requirement for data migration is also a problem in Mackler's point of view. So how does SambaNova solve it?
You need to further understand the characteristics of the RDU architecture. In fact, it is easy to understand:
A is the paradigm of data exchange in the traditional GPU architecture. Each operator They all need to exchange data with off-chip DRAM. This back-and-forth exchange occupies a large amount of DDR bandwidth and should be easier to understand. B is what SambaNova's architecture can achieve. During the model calculation process, a large part of the data movement is kept on-chip, and there is no need to go back and forth to DRAM for exchange.
Therefore, If we can achieve the effect like B, if we have to choose between large bandwidth and large capacity, we can safely choose large capacity. This is as the following passage says:
“The question we have is this: What is more important in a hybrid memory architecture supporting foundation models, memory capacity or memory bandwidth? You can't have both based on a single memory technology in any architecture, and even when you have a mix of fast and skinny and slow and fat memories, where Nvidia and SambaNova draw the lines are different."
Facing the powerful NVIDIA , we are not without hope! However, following NVIDIA's GPGPU strategy may not be feasible. It seems that the correct idea for large chips is to use lower-cost DRAM. With the same computing power specifications, the performance can reach more than 6 times that of NVIDIA!
How does SambaNova's RDU/DataFlow architecture achieve the effect of B? Or are there other ways to achieve an effect similar to B? We will share it with you next time. Friends who are interested, please continue to pay attention to our updates
Extended reading materials:
[1]https:/ /sambanova.ai/blog/a-new-state-of-the-art-in-nlp-beyond-gpus/
[2]https://www.nextplatform. com/2022/09/17/sambanova-doubles-up-chips-to-chase-ai-foundation-models/
[3]https://hc33.hotchips.org /assets/program/conference/day2/SambaNova HotChips 2021 Aug 23 v1.pdf
[4]《TRAINING LARGE LANGUAGE MODELS EFFICIENTLY WITH SPARSITY AND DATAFLOW》
[5]https://www.php.cn/link/617974172720b96de92525536de581fa
##The content that needs to be rewritten is: [6]https://www.php .cn/link/a56ee48e5c142c26cf645b2cc23d78fc
The above is the detailed content of How does DSA overtake NVIDIA GPU in a corner?. For more information, please follow other related articles on the PHP Chinese website!

Hot AI Tools

Undresser.AI Undress
AI-powered app for creating realistic nude photos

AI Clothes Remover
Online AI tool for removing clothes from photos.

Undress AI Tool
Undress images for free

Clothoff.io
AI clothes remover

AI Hentai Generator
Generate AI Hentai for free.

Hot Article

Hot Tools

Notepad++7.3.1
Easy-to-use and free code editor

SublimeText3 Chinese version
Chinese version, very easy to use

Zend Studio 13.0.1
Powerful PHP integrated development environment

Dreamweaver CS6
Visual web development tools

SublimeText3 Mac version
God-level code editing software (SublimeText3)

Hot Topics


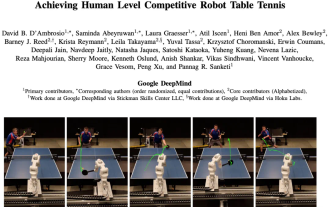
But maybe he can’t defeat the old man in the park? The Paris Olympic Games are in full swing, and table tennis has attracted much attention. At the same time, robots have also made new breakthroughs in playing table tennis. Just now, DeepMind proposed the first learning robot agent that can reach the level of human amateur players in competitive table tennis. Paper address: https://arxiv.org/pdf/2408.03906 How good is the DeepMind robot at playing table tennis? Probably on par with human amateur players: both forehand and backhand: the opponent uses a variety of playing styles, and the robot can also withstand: receiving serves with different spins: However, the intensity of the game does not seem to be as intense as the old man in the park. For robots, table tennis
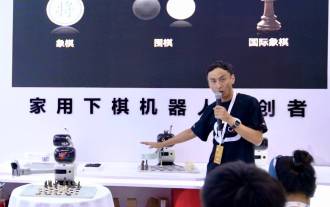
On August 21, the 2024 World Robot Conference was grandly held in Beijing. SenseTime's home robot brand "Yuanluobot SenseRobot" has unveiled its entire family of products, and recently released the Yuanluobot AI chess-playing robot - Chess Professional Edition (hereinafter referred to as "Yuanluobot SenseRobot"), becoming the world's first A chess robot for the home. As the third chess-playing robot product of Yuanluobo, the new Guoxiang robot has undergone a large number of special technical upgrades and innovations in AI and engineering machinery. For the first time, it has realized the ability to pick up three-dimensional chess pieces through mechanical claws on a home robot, and perform human-machine Functions such as chess playing, everyone playing chess, notation review, etc.
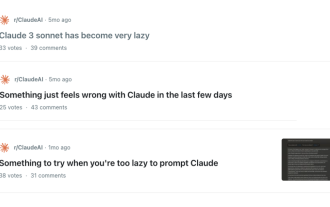
The start of school is about to begin, and it’s not just the students who are about to start the new semester who should take care of themselves, but also the large AI models. Some time ago, Reddit was filled with netizens complaining that Claude was getting lazy. "Its level has dropped a lot, it often pauses, and even the output becomes very short. In the first week of release, it could translate a full 4-page document at once, but now it can't even output half a page!" https:// www.reddit.com/r/ClaudeAI/comments/1by8rw8/something_just_feels_wrong_with_claude_in_the/ in a post titled "Totally disappointed with Claude", full of
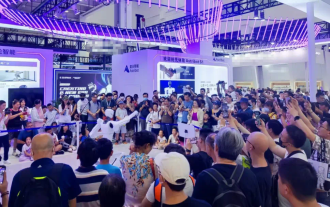
At the World Robot Conference being held in Beijing, the display of humanoid robots has become the absolute focus of the scene. At the Stardust Intelligent booth, the AI robot assistant S1 performed three major performances of dulcimer, martial arts, and calligraphy in one exhibition area, capable of both literary and martial arts. , attracted a large number of professional audiences and media. The elegant playing on the elastic strings allows the S1 to demonstrate fine operation and absolute control with speed, strength and precision. CCTV News conducted a special report on the imitation learning and intelligent control behind "Calligraphy". Company founder Lai Jie explained that behind the silky movements, the hardware side pursues the best force control and the most human-like body indicators (speed, load) etc.), but on the AI side, the real movement data of people is collected, allowing the robot to become stronger when it encounters a strong situation and learn to evolve quickly. And agile
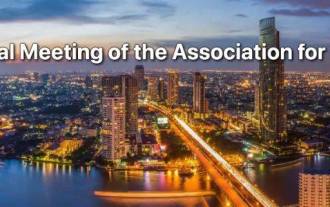
At this ACL conference, contributors have gained a lot. The six-day ACL2024 is being held in Bangkok, Thailand. ACL is the top international conference in the field of computational linguistics and natural language processing. It is organized by the International Association for Computational Linguistics and is held annually. ACL has always ranked first in academic influence in the field of NLP, and it is also a CCF-A recommended conference. This year's ACL conference is the 62nd and has received more than 400 cutting-edge works in the field of NLP. Yesterday afternoon, the conference announced the best paper and other awards. This time, there are 7 Best Paper Awards (two unpublished), 1 Best Theme Paper Award, and 35 Outstanding Paper Awards. The conference also awarded 3 Resource Paper Awards (ResourceAward) and Social Impact Award (
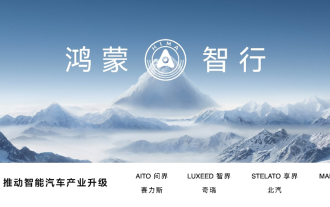
This afternoon, Hongmeng Zhixing officially welcomed new brands and new cars. On August 6, Huawei held the Hongmeng Smart Xingxing S9 and Huawei full-scenario new product launch conference, bringing the panoramic smart flagship sedan Xiangjie S9, the new M7Pro and Huawei novaFlip, MatePad Pro 12.2 inches, the new MatePad Air, Huawei Bisheng With many new all-scenario smart products including the laser printer X1 series, FreeBuds6i, WATCHFIT3 and smart screen S5Pro, from smart travel, smart office to smart wear, Huawei continues to build a full-scenario smart ecosystem to bring consumers a smart experience of the Internet of Everything. Hongmeng Zhixing: In-depth empowerment to promote the upgrading of the smart car industry Huawei joins hands with Chinese automotive industry partners to provide

Deep integration of vision and robot learning. When two robot hands work together smoothly to fold clothes, pour tea, and pack shoes, coupled with the 1X humanoid robot NEO that has been making headlines recently, you may have a feeling: we seem to be entering the age of robots. In fact, these silky movements are the product of advanced robotic technology + exquisite frame design + multi-modal large models. We know that useful robots often require complex and exquisite interactions with the environment, and the environment can be represented as constraints in the spatial and temporal domains. For example, if you want a robot to pour tea, the robot first needs to grasp the handle of the teapot and keep it upright without spilling the tea, then move it smoothly until the mouth of the pot is aligned with the mouth of the cup, and then tilt the teapot at a certain angle. . this
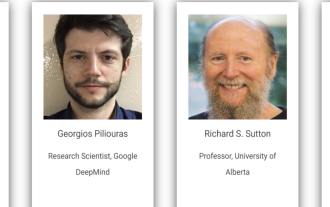
Conference Introduction With the rapid development of science and technology, artificial intelligence has become an important force in promoting social progress. In this era, we are fortunate to witness and participate in the innovation and application of Distributed Artificial Intelligence (DAI). Distributed artificial intelligence is an important branch of the field of artificial intelligence, which has attracted more and more attention in recent years. Agents based on large language models (LLM) have suddenly emerged. By combining the powerful language understanding and generation capabilities of large models, they have shown great potential in natural language interaction, knowledge reasoning, task planning, etc. AIAgent is taking over the big language model and has become a hot topic in the current AI circle. Au
