In the year when large models have exploded, the most important development bottleneck in the generative AI industry has appeared on the computing power side. On September 20, Sequoia America mentioned in the article "The Second Act of Generative AI" that many generative AI companies quickly discovered in the past year that their development bottleneck was not customer demand, but GPU tension. Long GPU wait times became so normal that a simple business model emerged: pay a subscription fee to skip the queue and get better models. In the training of large models, the exponential increase in parameter size has brought about a sharp increase in training costs. For tight GPU resources, it has become more important to maximize the performance of the hardware and improve training efficiency. AI development computing platform is an important solution. Using the AI development computing platform, a large model developer can complete the entire AI development process covering data preparation, model development, model training and model deployment in one stop. In addition to lowering the threshold for large model development, the AI computing platform makes computing resources more efficient by providing training optimization and inference management services. On September 26, according to JD Cloud’s introduction of the Yanxi AI development computing platform at the Xi’an City Conference, the Yanxi AI development computing platform launched by JD Cloud was used , the entire process from data preparation, model training, to model deployment can be completed in less than a week; it previously required a team of more than 10 scientists to work, but now only requires 1-2 algorithmic staff; through platform model acceleration tool optimization, use The team was able to save 90% of inference costs. More importantly, at a time when large models are rapidly entering thousands of industries, the Yanxi AI development computing platform simultaneously supports large model algorithm developers and application developers. Empowerment, for application developers can also use low-code form to achieve large-model product development. The threshold for developing large industrial models has become lower, and it will be easier to utilize and transform large models. 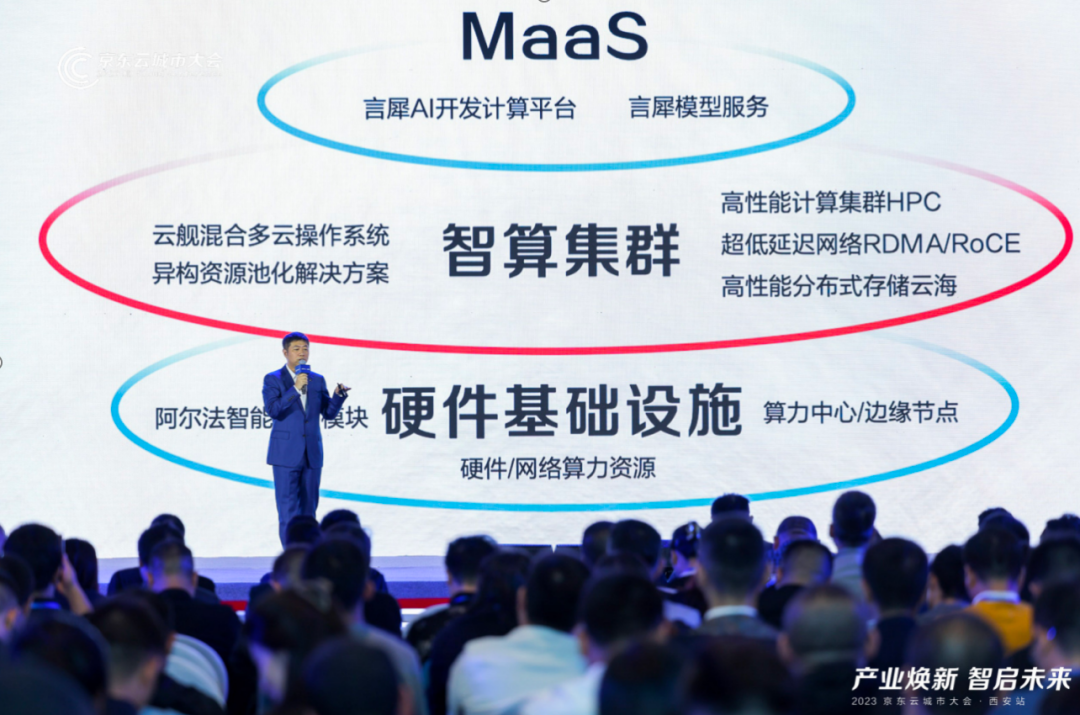
The era of large models requires new digital infrastructureFor a large model developer, if there is no AI development computing platform, This means: During the development process of algorithms and applications, you need to build a series of systems such as scheduling of underlying GPU computing resources, storage network, and model management and control. The overall development process will be very primitive and the threshold is very high. For a company that is promoting large model industry applications internally, this means a rapid increase in costs and difficulty in ensuring training efficiency. One year has passed, and industries such as finance, marketing, automobiles, content, law, and office are actively integrating with large models. The powerful potential of large models has become an important factor in restructuring the competitive landscape in many industries. Finding scenarios that combine your business with large models faster and implementing them efficiently has become the key to competition. But developing an industry model is not a smooth process. Today we still face a series of challenges and opportunities: In terms of data, data from different industries show different concentrations and dispersion states, and data preparation cycles and processing difficulties vary; how to efficiently load massive multi-modal data during training is a problem that must be solved. Secondly, the stability of the environment during large model training, the recovery of fault breakpoints and the processing of continued training have a great impact on the training efficiency; in the training and deployment links, How to efficiently schedule computing power and improve the utilization of computing power resources is also a cost issue that enterprises must consider. JD Cloud shared at the Xi'an City Conference that in the past period of practice, JD Cloud found that the challenges of large industrial models are not just technical In itself, how to combine technology with industry application scenarios and how to balance cost, efficiency, and experience are the real challenges for the implementation of large model industries. Returning to the most basic development level and balancing cost, efficiency, and experience means that some problems need to be re-solved and optimized. Gong Yicheng, head of the IaaS product research and development department of Jingdong Cloud, further explained in the interview that the requirements for development infrastructure in the era of large models have been hugely different from traditional ones. In terms of efficiency, in the past AI development process, even relatively low-cost GPUs could complete a lot of related work. However, in large-model scenarios, AI development has relied heavily on high-cost GPUs such as A100 and A800. Power and performance requirements are becoming higher, and costs are rising rapidly. "Therefore, under the high cost, how to squeeze the performance of these hardware to the extreme has become particularly important for the cost efficiency of large model development." In the past development of AI, the concurrency of data throughput was not as large as that of large models, because it required many GPUs to work at the same time. Therefore, even if the amount of data itself is not large, the Concurrent reading of the model and the possible delay issues have put forward new requirements for high-performance storage, which are usually unable to be met by previous storage mechanisms. Gong Yicheng also mentioned that if the delay is lower during the data access process, the efficiency of the entire model will be higher. If you use self-developed smart chips, you can completely use low-latency networks, which can help improve the efficiency of the entire model training. In addition, at the scale level, large model training with more than 100 billion parameters basically requires more than 1,000 calories for training. Gong Yicheng shared that this was extremely rare in previous AI development, so it put forward high and new experience requirements for development, and the corresponding development infrastructure was completely different. For companies that want to improve the efficiency of large model development and help large models to be better implemented in the industry, a new set of infrastructure has become necessary. JD Cloud releases Yanxi AI computing platform September 26, JD.com Yanxi AI development and computing platform was officially released at the Xi'an City Conference. The product covers the entire AI development process of data preparation, model development, model training and model deployment, and is preset with mainstream open source large models and some commercial large models. , as well as more than one hundred inference tools and frameworks, can effectively reduce the threshold and cost of large model development. 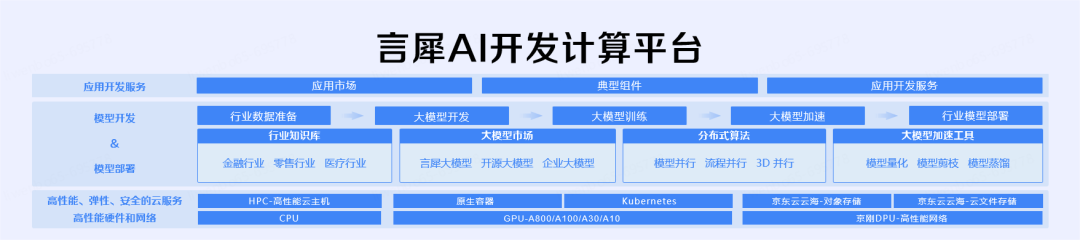
In terms of performance improvement, Yanxi AI development computing platform has made many technological breakthroughs in computing power and storage. At the bottom level, the platform can further overall schedule and coordinate GPU computing power to improve the scheduling efficiency of the platform's underlying resource usage. According to JD Cloud’s sharing, in terms of computing power, JD Cloud will provide fifth-generation cloud hosts and various high-performance product forms in the platform, It can provide computing power and support hundreds of thousands of GPU node scales. At the network level, the self-developed RDMA congestion algorithm is used to globally control the RDMA network traffic path. Different GPU nodes support a maximum of 3.2 Tbps RDMA network bandwidth, and the transmission delay is as low as about 2 us as basic capability support. In terms of storage, JD Cloud’s Yunhai distributed storage can support large model massive data and high concurrency cluster requirements for large model training data throughput. Achieve tens of millions of IOPS, with latency as low as 100 microseconds. With the new storage and computing separation architecture, Yunhai can save customers more than 30% of the overall infrastructure cost. It is now widely used in emerging scenarios such as high-performance computing and AI training, as well as traditional scenarios such as audio and video storage and data reporting. In addition to optimizing underlying resources, the Yanxi AI computing platform can help large model developers improve the efficiency of the entire link and efficiently implement data processing, model development, Training, deployment, evaluation, training inference optimization, model security, etc.:
- In the data management process, Yan Xi can Through intelligent annotation models, data enhancement models, and data conversion toolsets, it helps model developers implement all aspects of data import, cleaning, annotation, and enhancement. It supports data import and intelligent analysis in multiple file formats, and provides automatic and semi-automatic data annotation capabilities. . Help solve problems such as scattered data storage, different data formats, uneven data quality, and low efficiency of manual data annotation.
- In the distributed training process, Yanxi platform adapts to domestic hardware, supports HPC, and integrates high-performance file systems; it provides resource allocation and scheduling strategies to ensure hardware Resources are fully utilized; a unified interactive interface is provided to simplify the management of training tasks. Help solve the problem of rapid growth in network and algorithm complexity, resulting in scarcity and waste of computing resources; difficulties in the use and adaptation of HPC, high-performance computing, high-performance file systems, and heterogeneous hardware; diversity of model training, and training and learning costs Improvement and other issues.
- In terms of code-free development capabilities, further simplifies the development process of large-scale models. Users can directly select the large model built into the platform, upload data, and then continue to select a training method. After specifying one of the two codeless training methods of hyperparameters and AutoML, a fine-tuned model or application will be obtained.
- In the application layer, the Yanxi platform has built-in no-code development tools for common application scenarios such as question and answer development, document analysis development, and plug-in development. After selecting the model, knowledge base, prompt template and development platform, deploy it with one click. And can support monitoring, tracking testing and test evaluation.
Overall, Yanxi AI development computing platform can meet the needs of users with different professional levels. For large model algorithm developers, it can support the entire process from data preparation, model selection, code tuning, deployment and release, etc. For application layer developers, they can use a codeless approach to visually select models, upload data, and configure parameters. They can trigger tasks without writing code and start training model tasks, thus lowering the threshold. In terms of introducing models, the platform currently has built-in commercial models such as Yanxi, Spark, and LLama2, as well as open source models. Gong Yicheng said that Yanxi’s approach to model selection tends to focus more on quality than quantity: it chooses relatively outstanding business models in various technical fields, as well as some industry models built around basic models, to avoid users falling into choice anxiety. Moreover, Yanxi will focus on introducing industry model applications built by JD.com based on basic models, such as retail, health scenarios, and industry applications that have actually achieved large-scale implementation. The model is put on the platform to help platform developers promote the implementation of related businesses. Currently Yanxi has three delivery methods: One is the MaaS service form, which developers can explore and use through the API in a pay-as-you-go, cost-effective way. The second is the public cloud SaaS version. Users can use the one-stop model development, training and deployment capabilities provided by the platform. Based on the advantages of elastic supply of public cloud resources, users can start the development and development of large industrial models at minimized costs. deploy. The third is a privatized delivery version to meet customers who have more special requirements for data security, and the data is completely localized. In the future, Yanxi will continue to upgrade its platform capabilities, including domestic hardware coverage, model ecological cooperation, plug-in development, application evaluation services, all-in-one machine delivery, Agent development services, etc. Continue to improve in this aspect, systematically helping to solve the difficulties in the development and implementation of large industrial models, the difficulty in developing large model applications, the high cost of model training and inference, the difficulty in obtaining models and applications, the use of high-performance computing, high-performance files, and heterogeneous hardware. and adaptation difficulties. Promote the implementation of large models in thousands of industriesAt the Xi'an City Conference, Cao Peng, chairman of JD Group’s technical committee and president of JD Cloud Division, mentioned in his speech that in the process of gradually implementing large models into the industry, he hopes to improve better industrial efficiency, generate greater industrial value, and be able to operate in greater Copying in multiple scenarios essentially puts forward higher requirements for the model training process and infrastructure: the model needs to be easier to use, have a lower threshold and lower cost, and can flexibly use computing power. AI development computing platform is one of the important solutions to solve these problems. A high-performance and easy-to-use AI development computing platform can allow more industry parties to participate at a low cost Come to the construction of the large model industry to stimulate the emergence of more industrial large models and accelerate the implementation of large models in thousands of industries. In the actual market, Gong Yicheng said that when industry customers choose an AI computing platform, the main highlights they will consider are: industry understanding and platform efficiency. Compared with other AI computing platforms, Yanxi AI development computing platform can not only improve the ultimate performance, but also combine JD.com’s long-term experience in advantageous scenarios such as retail, finance, logistics, and health, and have a more professional industrial giant. Model selection. In the model ecosystem of Yanxi AI computing platform, in addition to built-in excellent business models and open source models, in order to further lower the threshold,
Yanxi AI computing platform will also Supplement these large models with further enhanced capabilities, such as Chinese ability, math ability, etc., allowing users to choose large models that are easier to use and more professional.
More importantly, since the Yanxi AI development and computing platform is also aimed at large model application developers, it supports the construction of proprietary models in a codeless manner,In addition to the above In addition to the basic models, the Yanxi platform will also provide users with more proprietary models
for application scenarios, allowing users to quickly implement them in their own industries.
Currently, the application scenario-specific models provided by Yanxi Platform mainly include mature high-frequency scenarios such as question and answer development and document analysis and development. These applications Jingdong has been verified many times in its own advantageous areas, and combined with large models can quickly improve efficiency.
Taking conversation tools as an example, starting from 2021, Miniso and JD Cloud have reached a cooperation to apply a series of technical products of Yanxi under JD Cloud in customer service. In MINISO, it covers the MINISO store customer service team, user operation team, and IT service operation and maintenance team. In April 2022, the Yanxi series of products were launched one after another, including a series of intelligent products such as online customer service robots, voice response robots, voice outbound call robots, intelligent quality inspection, and intelligent knowledge bases, which have brought significant results.
Feedback data shows that the current average daily consultation service volume of Yanxi series products is nearly 10,000 times, among which the online customer service robot response accuracy rate exceeds 97%, and the independent reception rate exceeds 70% , reducing service costs by 40%; the response accuracy of the voice response robot exceeds 93%, and independently handles 46.1% of customer problems; intelligent quality inspections have been completed hundreds of thousands of times, and nearly 3,000 service risk issues have been discovered and dealt with, making users satisfied degree increased by 20%; the content of the intelligent knowledge base covers approximately 8,800 core SKUs under the "MINISO" brand and approximately 4,600 SKUs under the "TOP TOY" brand. #The practical process of implementing large models has reached the stage of spreading from a single point to outwards.
In the industry, there are many industrial companies similar to Miniso, and conversational robot scenarios can bring greater value to them. In terms of the launch of the Xi AI development and computing platform, it will empower industrial companies from the bottom-level computing power, data management, code-free applications and other full links, and will provide these companies with a lower threshold, lower cost, and shorter training cycle. large-scale model industrialization plan. It is foreseeable that cases similar to Miniso will appear more frequently in the future.
In addition, JD Cloud emphasized that compared with other competing products, the low-code aspect adopted by JD Yanxi AI computing platform further slows down the development of application developers. threshold, and it is completely independent in terms of high-performance storage, the overall technical system has complete adaptability and high performance efficiency.
With the popularization of new digital infrastructure, the implementation of large models in thousands of industries will become faster, and the impossible triangle of cost efficiency and innovation will become wider. imagination space. The above is the detailed content of Bringing large-scale models within easy reach, Yanxi AI development and computing platform is officially launched. For more information, please follow other related articles on the PHP Chinese website!