


Analyzing the interpretability of large models: a review reveals the truth and answers doubts
Paper link: https://arxiv.org/abs/2309.01029 Github link: https://github.com/hy-zhao23/Explainability-for-Large-Language-Models
High model complexity. Different from deep learning models or traditional statistical machine learning models before the LLM era, LLMs models are huge in scale and contain billions of parameters. Their internal representation and reasoning processes are very complex, and it is difficult to explain their specific outputs. Strong data dependence. LLMs rely on large-scale text corpus during the training process. Bias, errors, etc. in these training data may affect the model, but it is difficult to completely judge the impact of the quality of the training data on the model. Black box nature. We usually think of LLMs as black box models, even for open source models such as Llama-2. It is difficult for us to explicitly judge its internal reasoning chain and decision-making process. We can only analyze it based on input and output, which makes interpretability difficult. Output uncertainty. The output of LLMs is often uncertain, and different outputs may be produced for the same input, which also increases the difficulty of interpretability. Insufficient evaluation indicators. The current automatic evaluation indicators of dialogue systems are not enough to fully reflect the interpretability of the model, and more evaluation indicators that consider human understanding are needed.
#1. The purpose of feature attribution is to measure the correlation between each input feature (e.g. word, phrase, text range) and the model prediction. Feature attribution methods can be divided into:
Perturbation-based interpretation, observing the impact on the output results by modifying specific input features
According to the interpretation of the gradient, the partial differential of the output to the input is used as the importance index of the corresponding input
Alternative model, use a simple human-understandable model to fit a single component of the complex model Output to obtain the importance of each input;
Decomposition-based technology aims to linearly decompose feature correlation scores.
Attention visualization technology to intuitively observe changes in attention scores on different scales; Function-based interpretation, such as outputting the partial differential of attention. However, the use of attention as a research perspective remains controversial in the academic community.
Adversarial examples are data generated for the characteristics of the model that are very sensitive to small changes. In natural language processing, they are usually obtained by modifying the text, which is difficult for humans to Different text transformations often lead to different predictions from the model. Counterfactual samples are obtained by deforming the text such as negation, which is usually a test of the model's causal inference ability.
- Probe-based interpretation Probe interpretation technology is mainly based on classifier detection, by training a shallow layer on a pre-trained model or a fine-tuned model The classifier is then evaluated on a holdout dataset, enabling the classifier to identify language features or reasoning abilities.
- Neuron activation Traditional neuron activation analysis only considers a part of important neurons, and then learns the relationship between neurons and semantic features. Recently, GPT-4 has also been used to explain neurons. Instead of selecting some neurons for explanation, GPT-4 can be used to explain all neurons.
- Concept-based interpretation The input is first mapped to a set of concepts and then the model is interpreted by measuring the importance of the concepts to the prediction.
- # #The benefits of explanation for model learning Explore whether explanation is helpful for model learning in the case of few-shot learning.
- Situational Learning Explore the mechanism of situational learning in large models, and distinguish the difference between situational learning in large models and medium models.
- Thinking chain prompting Explore the thinking chain prompting to improve the performance of the model.
- Fine-tuning's role assistant model is usually pre-trained to obtain general semantic knowledge, and then acquires domain knowledge through supervised learning and reinforcement learning. The stage at which the knowledge of the assistant model mainly comes from remains to be studied.
- Illusion and Uncertainty The accuracy and credibility of large model predictions are still important topics of current research. Despite the powerful inference capabilities of large models, their results often suffer from misinformation and hallucinations. This uncertainty in prediction brings huge challenges to its widespread application.
##The evaluation indicators explained by the model include plausibility, faithfulness, stability, robustness, etc. The paper mainly talks about two widely concerned dimensions: 1) rationality to humans; 2) fidelity to the internal logic of the model.
The above is the detailed content of Analyzing the interpretability of large models: a review reveals the truth and answers doubts. For more information, please follow other related articles on the PHP Chinese website!

Hot AI Tools

Undresser.AI Undress
AI-powered app for creating realistic nude photos

AI Clothes Remover
Online AI tool for removing clothes from photos.

Undress AI Tool
Undress images for free

Clothoff.io
AI clothes remover

Video Face Swap
Swap faces in any video effortlessly with our completely free AI face swap tool!

Hot Article

Hot Tools

Notepad++7.3.1
Easy-to-use and free code editor

SublimeText3 Chinese version
Chinese version, very easy to use

Zend Studio 13.0.1
Powerful PHP integrated development environment

Dreamweaver CS6
Visual web development tools

SublimeText3 Mac version
God-level code editing software (SublimeText3)

Hot Topics


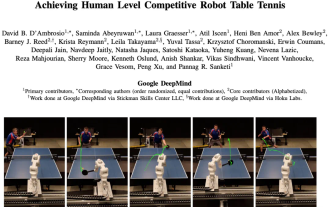
But maybe he can’t defeat the old man in the park? The Paris Olympic Games are in full swing, and table tennis has attracted much attention. At the same time, robots have also made new breakthroughs in playing table tennis. Just now, DeepMind proposed the first learning robot agent that can reach the level of human amateur players in competitive table tennis. Paper address: https://arxiv.org/pdf/2408.03906 How good is the DeepMind robot at playing table tennis? Probably on par with human amateur players: both forehand and backhand: the opponent uses a variety of playing styles, and the robot can also withstand: receiving serves with different spins: However, the intensity of the game does not seem to be as intense as the old man in the park. For robots, table tennis
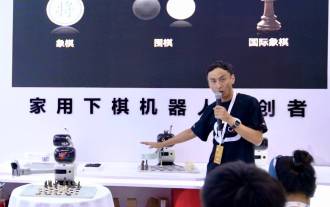
On August 21, the 2024 World Robot Conference was grandly held in Beijing. SenseTime's home robot brand "Yuanluobot SenseRobot" has unveiled its entire family of products, and recently released the Yuanluobot AI chess-playing robot - Chess Professional Edition (hereinafter referred to as "Yuanluobot SenseRobot"), becoming the world's first A chess robot for the home. As the third chess-playing robot product of Yuanluobo, the new Guoxiang robot has undergone a large number of special technical upgrades and innovations in AI and engineering machinery. For the first time, it has realized the ability to pick up three-dimensional chess pieces through mechanical claws on a home robot, and perform human-machine Functions such as chess playing, everyone playing chess, notation review, etc.
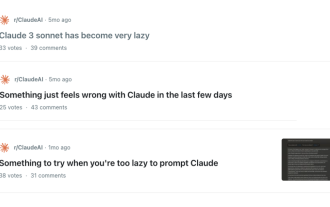
The start of school is about to begin, and it’s not just the students who are about to start the new semester who should take care of themselves, but also the large AI models. Some time ago, Reddit was filled with netizens complaining that Claude was getting lazy. "Its level has dropped a lot, it often pauses, and even the output becomes very short. In the first week of release, it could translate a full 4-page document at once, but now it can't even output half a page!" https:// www.reddit.com/r/ClaudeAI/comments/1by8rw8/something_just_feels_wrong_with_claude_in_the/ in a post titled "Totally disappointed with Claude", full of
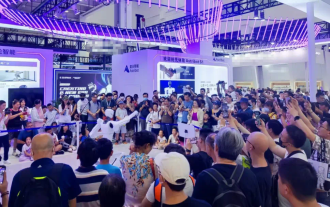
At the World Robot Conference being held in Beijing, the display of humanoid robots has become the absolute focus of the scene. At the Stardust Intelligent booth, the AI robot assistant S1 performed three major performances of dulcimer, martial arts, and calligraphy in one exhibition area, capable of both literary and martial arts. , attracted a large number of professional audiences and media. The elegant playing on the elastic strings allows the S1 to demonstrate fine operation and absolute control with speed, strength and precision. CCTV News conducted a special report on the imitation learning and intelligent control behind "Calligraphy". Company founder Lai Jie explained that behind the silky movements, the hardware side pursues the best force control and the most human-like body indicators (speed, load) etc.), but on the AI side, the real movement data of people is collected, allowing the robot to become stronger when it encounters a strong situation and learn to evolve quickly. And agile
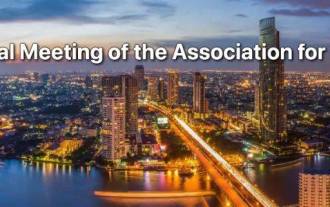
At this ACL conference, contributors have gained a lot. The six-day ACL2024 is being held in Bangkok, Thailand. ACL is the top international conference in the field of computational linguistics and natural language processing. It is organized by the International Association for Computational Linguistics and is held annually. ACL has always ranked first in academic influence in the field of NLP, and it is also a CCF-A recommended conference. This year's ACL conference is the 62nd and has received more than 400 cutting-edge works in the field of NLP. Yesterday afternoon, the conference announced the best paper and other awards. This time, there are 7 Best Paper Awards (two unpublished), 1 Best Theme Paper Award, and 35 Outstanding Paper Awards. The conference also awarded 3 Resource Paper Awards (ResourceAward) and Social Impact Award (
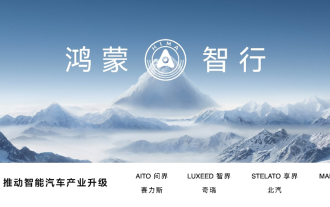
This afternoon, Hongmeng Zhixing officially welcomed new brands and new cars. On August 6, Huawei held the Hongmeng Smart Xingxing S9 and Huawei full-scenario new product launch conference, bringing the panoramic smart flagship sedan Xiangjie S9, the new M7Pro and Huawei novaFlip, MatePad Pro 12.2 inches, the new MatePad Air, Huawei Bisheng With many new all-scenario smart products including the laser printer X1 series, FreeBuds6i, WATCHFIT3 and smart screen S5Pro, from smart travel, smart office to smart wear, Huawei continues to build a full-scenario smart ecosystem to bring consumers a smart experience of the Internet of Everything. Hongmeng Zhixing: In-depth empowerment to promote the upgrading of the smart car industry Huawei joins hands with Chinese automotive industry partners to provide
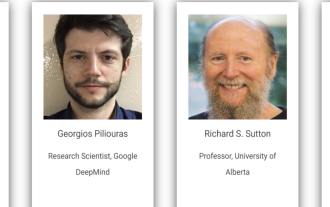
Conference Introduction With the rapid development of science and technology, artificial intelligence has become an important force in promoting social progress. In this era, we are fortunate to witness and participate in the innovation and application of Distributed Artificial Intelligence (DAI). Distributed artificial intelligence is an important branch of the field of artificial intelligence, which has attracted more and more attention in recent years. Agents based on large language models (LLM) have suddenly emerged. By combining the powerful language understanding and generation capabilities of large models, they have shown great potential in natural language interaction, knowledge reasoning, task planning, etc. AIAgent is taking over the big language model and has become a hot topic in the current AI circle. Au

Deep integration of vision and robot learning. When two robot hands work together smoothly to fold clothes, pour tea, and pack shoes, coupled with the 1X humanoid robot NEO that has been making headlines recently, you may have a feeling: we seem to be entering the age of robots. In fact, these silky movements are the product of advanced robotic technology + exquisite frame design + multi-modal large models. We know that useful robots often require complex and exquisite interactions with the environment, and the environment can be represented as constraints in the spatial and temporal domains. For example, if you want a robot to pour tea, the robot first needs to grasp the handle of the teapot and keep it upright without spilling the tea, then move it smoothly until the mouth of the pot is aligned with the mouth of the cup, and then tilt the teapot at a certain angle. . this
