Getting a great photo is getting easier and easier.
When traveling during the holidays, taking photos is indispensable. However, most of the photos taken in scenic spots are more or less regretful. Either there is something extra in the background, or there is something missing.
Obtaining a "perfect" image is one of the goals that CV researchers have long strived for. Recently, researchers from Google Research and Cornell University collaborated to propose an “Authentic Image Completion” technology—RealFill, a generative model for image completion.
The advantage of the RealFill model is that it can be personalized with a small number of scene reference images. These reference images do not need to be aligned with the target image, and can even be modified in terms of viewing angle, lighting conditions, camera aperture or image style. Big difference. Once personalization is complete, RealFill can complement the target image with visually engaging content in a way that is true to the original scene.
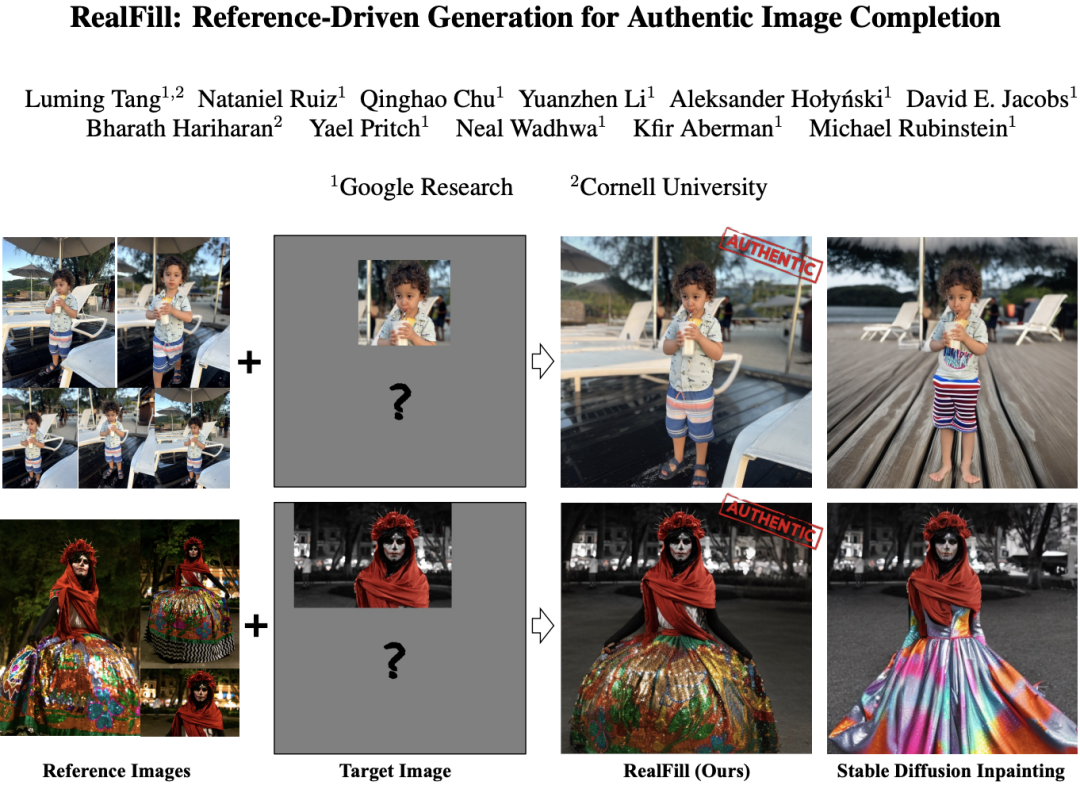
The inpainting and outpainting models are technologies that can generate high-quality and reasonable image content in unknown areas of the image, but the content generated by these models is necessarily unrealistic. , because these models have insufficient contextual information in real scenes. In contrast, RealFill generates content that "should" be there, making the results of image completion more realistic. The authors pointed out in the paper that they defined a new image completion problem - "Authentic Image Completion". Different from traditional generative image restoration (the content that replaces the missing area may be inconsistent with the original scene), the goal of real image completion is to make the completed content as faithful as possible to the original scene, using content that "should appear there". Complete the target image with content that “might be out there.” The authors stated that RealFill is the first method to extend the expressive power of generative image repair models by adding more conditions (i.e. adding reference images) to the process. RealFill significantly outperforms existing methods on a new image completion benchmark covering a diverse and challenging set of scenarios. RealFill’s goal is to maintain as much authenticity as possible , using a small number of reference images to complete the missing parts of a given target image. Specifically, you are given up to 5 reference images, and a target image that roughly captures the same scene (but may have a different layout or appearance). For a given scene, the researchers first create a personalized generative model by fine-tuning a pre-trained inpainting diffusion model on reference and target images. This fine-tuning process is designed so that the fine-tuned model not only maintains good image priors, but also learns the scene content, lighting, and style in the input image. This fine-tuned model is then used to fill in missing regions in the target image through a standard diffusion sampling process.
It is worth noting that for practical application value, this model pays special attention to more challenging, In the unconstrained case, the target image and the reference image may have very different viewpoints, environmental conditions, camera apertures, image styles, and even moving objects. According to the reference image on the left, RealFill can The target image is expanded (uncrop) or repaired (inpaint), and the generated result is not only visually appealing, but also consistent with the reference image, even if the reference image and the target image differ in aspects such as viewpoint, aperture, lighting, image style, and object motion. There are big differences. 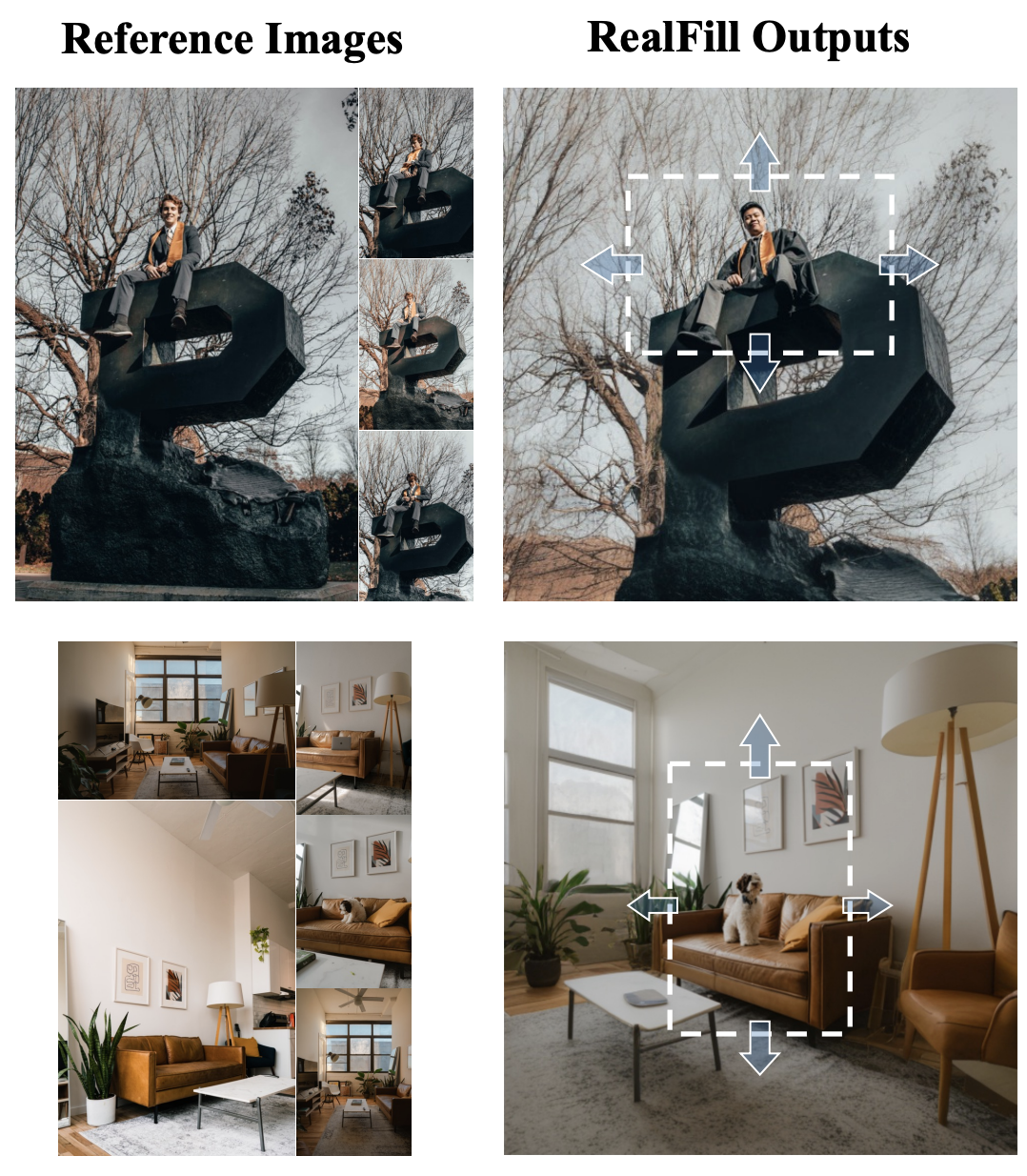
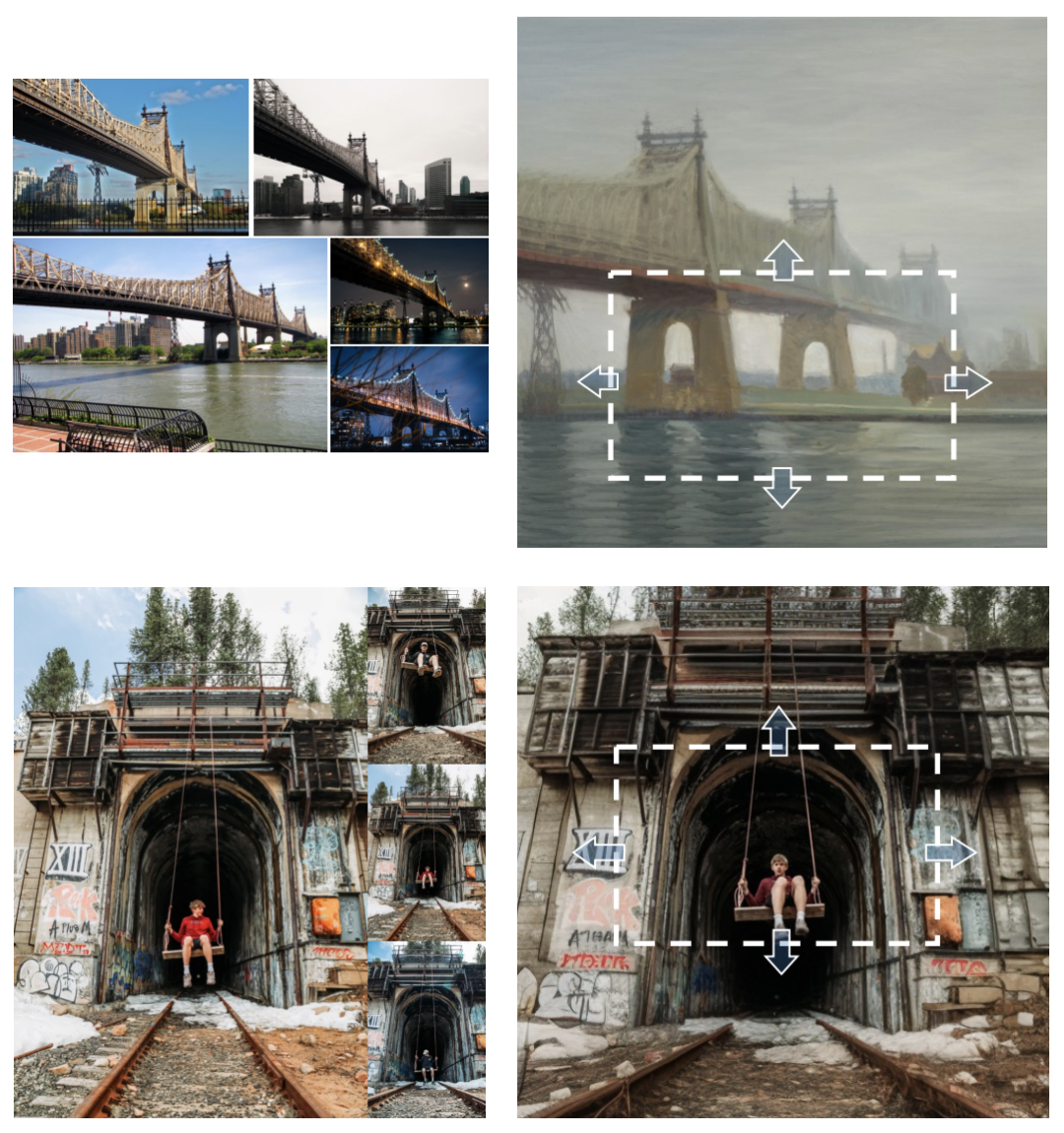
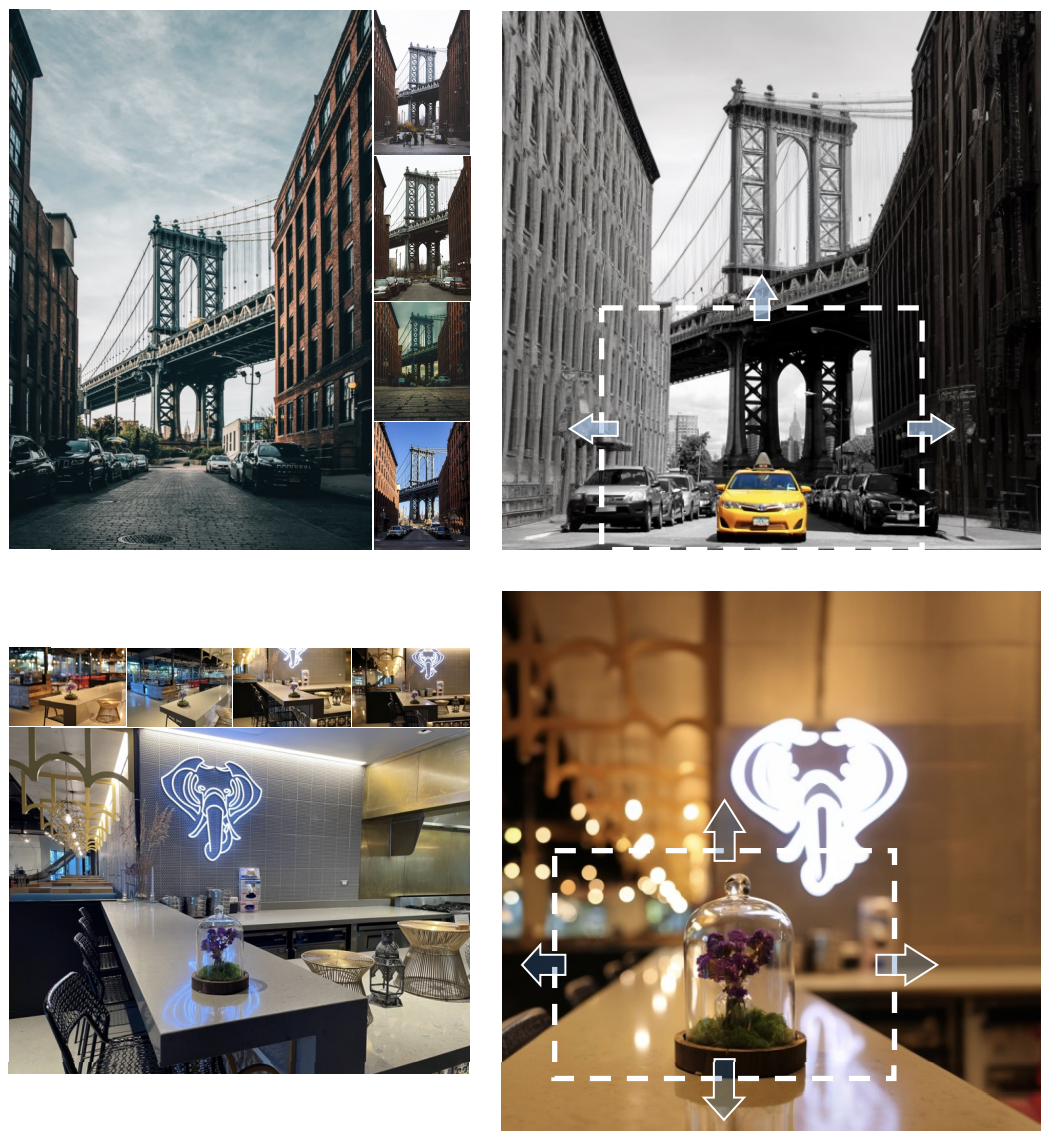
The output effect of RealFill model. Given a reference image on the left, RealFill can expand the corresponding target image on the right. The areas inside the white box are provided to the network as known pixels, while the areas outside the white box are generated. The results show that RealFill can generate high-quality images that are faithful to the reference image even if there are huge differences between the reference image and the target image, including viewpoint, aperture, lighting, image style, and object motion. Source: Paper
The researchers compared the RealFill model with other baseline methods. In comparison, RealFill produces high-quality results and performs better in terms of scene fidelity and consistency with reference images. Paint-by-Example cannot achieve a high degree of scene fidelity because it relies on CLIP embedding, which can only capture high-level semantic information. Stable Diffusion Inpainting can produce seemingly reasonable results, but due to the limited expressive power of prompt, the final generated results are not consistent with the reference image. 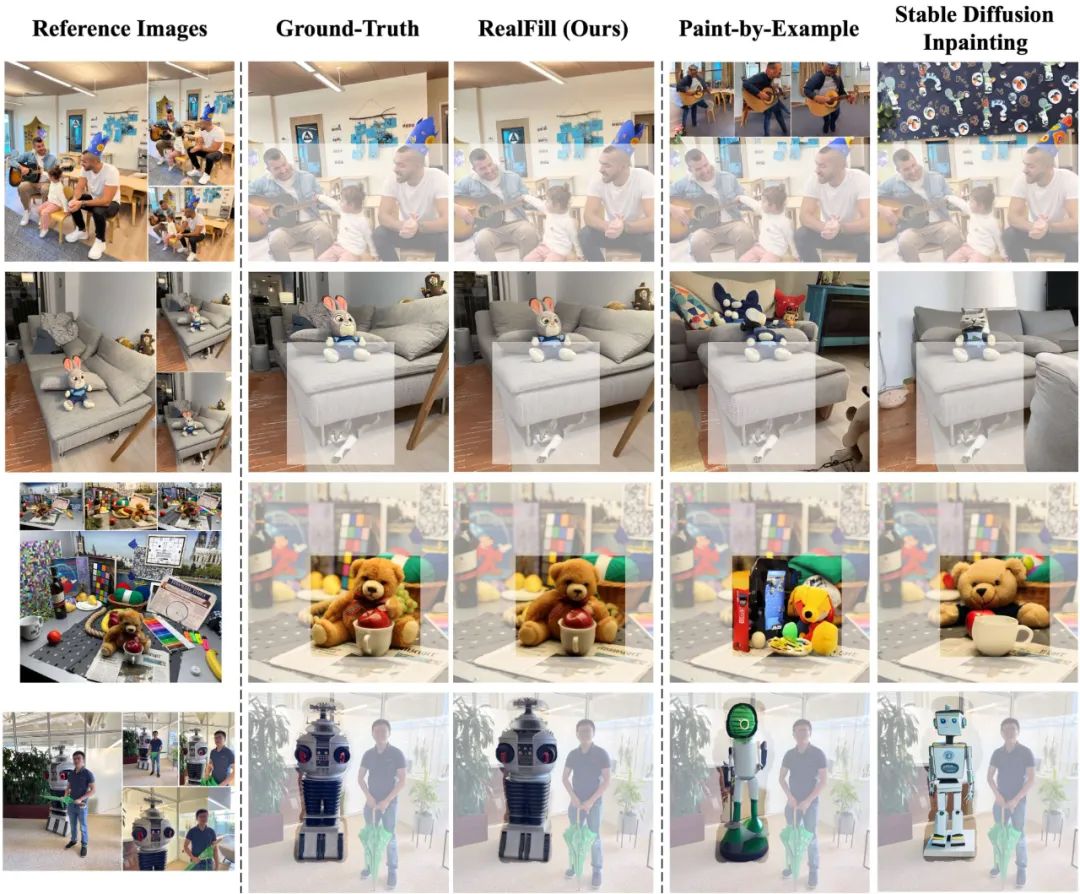
Comparison of RealFill with two other baseline methods. The area covered by a transparent white mask is the unmodified portion of the target image. Source: realfill.github.ioResearchers also Some potential issues and limitations of the RealFill model are discussed, including processing speed, the ability to handle viewpoint changes, and the ability to handle situations that are challenging for the underlying model. Specifically:
RealFill requires a gradient-based fine-tuning process on the input image, which makes it relatively slow to run.
When the viewpoint change between the reference image and the target image is very large, RealFill is often unable to restore the 3D scene, especially when there is only one reference image.
Since RealFill mainly relies on image priors inherited from the base pre-trained model, it cannot handle situations that are challenging for the base model, such as stable The diffusion model does not handle text well.
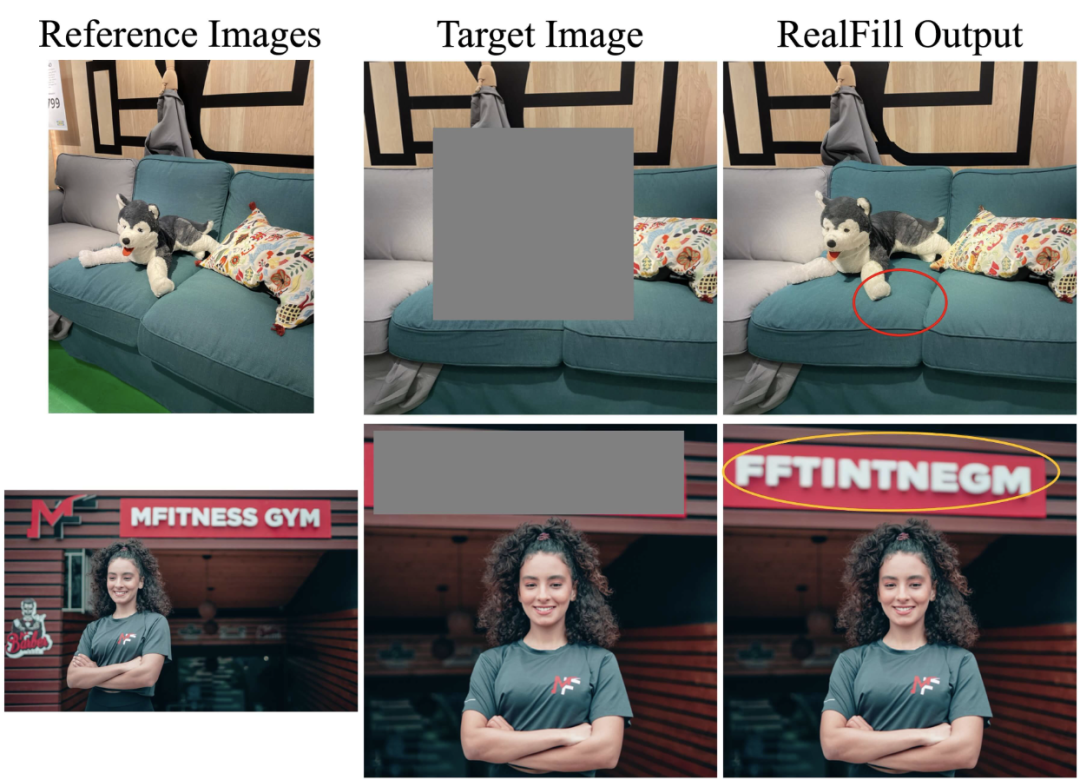
Finally, the author expresses his gratitude to the collaborators:
We would like to thank Rundi Wu, Qianqian Wang, Viraj Shah, Ethan Weber, Zhengqi Li , Kyle Genova, Boyang Deng, Maya Goldenberg, Noah Snavely, Ben Poole, Ben Mildenhall, Alex Rav-Acha, Pratul Srinivasan, Dor Verbin, and Jon Barron for valuable discussions and feedback, and also thanks to Zeya Peng, Rundi Wu, Shan Nan for Evaluate the contribution of the dataset. We are especially grateful to Jason Baldridge, Kihyuk Sohn, Kathy Meier-Hellstern, and Nicole Brichtova for their feedback and support on the project.
Please read the original paper and visit the project homepage for more information
The above is the detailed content of The authenticity is shocking! Google and Cornell University launch real-life image completion technology RealFill. For more information, please follow other related articles on the PHP Chinese website!