


ICCV'23 paper award 'Fighting of Gods'! Meta Divide Everything and ControlNet were jointly selected, and there was another article that surprised the judges
The ICCV 2023, the top computer vision conference held in Paris, France, has just ended!
This year’s Best Paper Award is simply a “fight between gods”.
For example, the two papers that won the Best Paper Award include the work that subverted the field of Vincentian graph AI-ControlNet.
Since being open source, ControlNet has received 24k stars on GitHub. Whether it is for the diffusion model or the entire field of computer vision, this paper's award is well-deserved
And the honorable mention for the best paper award is awarded to Gave another equally famous paper, Meta's "Split Everything" model SAM.
Since its launch, "Segment Everything" has become the "benchmark" for various image segmentation AI models, including many latecomers such as FastSAM, LISA, and SegGPT, all of which use it as a reference benchmark for effectiveness testing. .
The paper nominations are all so heavyweight. How fierce is the competition in this ICCV 2023?
A total of 8068 papers were submitted for ICCV 2023, but only about a quarter, that is, 2160 papers, were accepted
Nearly 10% of the papers were from China, and there were many besides universities Industrial institutions such as SenseTime and Joint Laboratories have 49 papers selected for ICCV 2023, and Megvii has 14 papers selected.
Let’s take a look at which papers won the ICCV 2023 awards
ControlNet won the best paper at ICCV
Let us first take a look at the best paper awards this year (Mar Two papers of the
ICCV best paper, also known as Marr Prize (Marr Prize),are selected every two years and are known as the best papers in the field of computer vision One of the highest honors.
This award is named after David Marr, a pioneer in the field of computer vision and the founder of computational neuroscience
The first best paper award winner is "Modeling Text to Image Diffusion" from Stanford Adding conditional control》
#This paper proposes a model called ControlNet, which only needs to add an additional input to the pre-trained diffusion model , you can control the details of its generation.
The input here can be of various types, including sketches, edge images, semantic segmentation images, human body key point features, Hough transform detection straight lines, depth maps, human bones, etc. The so-called "AI can draw hands" ”, the core technology comes from this article.
Its ideas and architecture are as follows:
The control network first copies the weights of the diffusion model to obtain a "trainable copy"
In contrast, the original diffusion model has been pre-trained on billions of images, so the parameters are "locked". And this "trainable copy" only needs to be trained on a small data set of a specific task to learn conditional control.
Even if the amount of data is very small (no more than 50,000 images), the conditional control generated by the model after training is very good.
Connected by a 1×1 convolution layer, the "locked model" and the "trainable copy" form a structure called "0 convolution layer". The weights and biases of this 0 convolutional layer are initialized to 0, so that a very fast speed can be obtained during the training process, close to the speed of fine-tuning the diffusion model, and can even be trained on personal devices
For example, if you use 200,000 image data to train an Nvidia RTX 3090TI, it will only take less than a week.
Zhang Lumin is the first author of the ControlNet paper and is currently the PhD student at Stanford University. In addition to ControlNet, he also created famous works such as Style2Paints and Fooocus
Paper address: https://arxiv.org/abs/2302.05543
Second paper「 Passive Ultra-Wideband Single-Photon Imaging" from the University of Toronto.
This paper was called "the most surprising paper on the topic" by the selection committee, so much so that one of the judges said "it was almost impossible for him to think of trying such a thing."
The abstract of the paper is as follows:
This paper discusses how to image dynamic scenes at extreme time scales (from seconds to picoseconds) simultaneously Imaging is required to be passive (without actively sending large amounts of light signals) and to take place in very sparse light situations, and does not rely on any timing signals from the light source.
Since existing optical flow estimation techniques for single-photon cameras fail in this range, this paper develops an optical flow detection theory that draws on the idea of stochastic calculus to Reconstruct the time-varying optical flow of pixels in the stream of photon detection timestamps.
Based on this theory, the paper mainly does three things:
(1) Shows that under low optical flow conditions, a passive free-running single-photon wavelength detector camera has an achievable frequency bandwidth, Spanning the entire spectrum from DC to 31 GHz;
(2) Derive a novel Fourier domain optical flow reconstruction algorithm for scanning timestamp data for frequencies with statistically significant support;
( 3) Ensure that the algorithm's noise model remains valid even at very low photon counts or non-negligible dead times.
The authors experimentally demonstrated the potential of this asynchronous imaging method, including some unprecedented capabilities:
(1) In the absence of synchronization (such as light bulbs, projectors, multi-pulse lasers) Under the hood, image scenes illuminated simultaneously by light sources running at different speeds;
(2) Passive non-line-of-sight video collection;
(3) Record ultra-wideband video, It can be played back at 30 Hz to show everyday movement, but it can also be played back at one billionth of a second to show how light travels.
The first author of the paper, Mian Wei, is a doctoral student at the University of Toronto. His research direction is computational photography. His current research interest lies in improving computer vision algorithms based on active illumination imaging technology.
Please click the following link to view the paper: https://openaccess.thecvf.com/content/ICCV2023/papers/Wei_Passive_Ultra-Wideband_Single-Photon_Imaging_ICCV_2023_paper.pdf
「Segmentation Everything" received an honorable mention
At this conference, in addition to ControNet, which attracted much attention, Meta's "Split Everything" model also received an honorable mention for the Best Paper Award, becoming a highly anticipated topic at the time. The topic
#This paper not only proposes a currently largest image segmentation data set with more than 1 billion masks on 11M images, but also A SAM model was trained that can quickly segment unseen images.
Compared with the previous fragmented image segmentation models, SAM can be said to have "unified" the functions of this series of models, and is effective in various tasks. Showed good performance.
This open source model has currently received 38.8k stars on GitHub, which can be said to be the "benchmark" in the field of semantic segmentation
Paper address: https://arxiv.org/abs/2304.02643
Project homepage: https://segment-anything.com/
In student works, Google's "Track Everything" model stands out
Just like the title of the article, this model can perform pixel-level tracking of any (multiple) objects in the image at any location at the same time.
The first author of this project is Qianqian Wang, a Chinese Ph.D. from Cornell University, who is currently conducting postdoctoral research at UCB.
Paper address: https://arxiv.org/abs/2306.05422
Project homepage: https://omnimotion. github.io/
At the opening ceremony, special awards donated by members of the PAMITC committee were also announced. The committee also donated awards for two computer vision field conferences, CVPR and WACV.
The following four awards are included in Inside:
- Helmholtz Prize: ICCV papers that had a major impact on computer vision research ten years ago
- Everingham Prize: Progress in the field of computer vision
- Outstanding Researcher: Researchers who have made significant contributions to the advancement of computer vision
- Rosenfeld Lifetime Achievement Award: Researchers who have made significant contributions to the field of computer vision over a long career
The scientists who won the Helmholtz Prize are Chinese scientists Heng Wang and Google's Cordelia Schmid, who are members of Meta AI
They relied on an article about action published in 2013 The papers identified received this award.
At that time, both of them were working in the Lear laboratory under the French National Institute of Computing and Automation (French abbreviation: INRIA), and Schmid was the leader of the laboratory at the time.
Please click the following link to view the paper: https://ieeexplore.ieee.org/document/6751553
The Everingham Award was awarded Awarded to two teams
The winners of the first group are Samer Agarwal, Keir Mierle and their teams from Google
The two winners graduated from the University of Washington and the University of Toronto respectively. The achievement is to develop an open source C library Ceres Solver
which is widely used in the field of computer vision. Project homepage link: http://ceres-solver.org /
Another award-winning result is the COCO data set, which contains a large number of images and annotations, has rich content and tasks, and is an important data set for testing computer vision models.
This data set was proposed by Microsoft. The first author of the relevant paper is Chinese scientist Tsung-Yi Lin. He graduated from Cornell University with a Ph.D. and now works as a researcher at NVIDIA Labs.
Paper address: https://arxiv.org/abs/1405.0312
Project homepage: https://cocodataset.org/
The recipients of the Outstanding Researcher honor are two professors, Michael Black from the Max Planck Institute in Germany and Rama Chellappa from Johns Hopkins University.
Professor Ted Adelson from MIT won the Lifetime Achievement Award
Is your paper accepted by ICCV 2023? Yet? What do you think of this year’s awards selection?
The above is the detailed content of ICCV'23 paper award 'Fighting of Gods'! Meta Divide Everything and ControlNet were jointly selected, and there was another article that surprised the judges. For more information, please follow other related articles on the PHP Chinese website!

Hot AI Tools

Undresser.AI Undress
AI-powered app for creating realistic nude photos

AI Clothes Remover
Online AI tool for removing clothes from photos.

Undress AI Tool
Undress images for free

Clothoff.io
AI clothes remover

AI Hentai Generator
Generate AI Hentai for free.

Hot Article

Hot Tools

Notepad++7.3.1
Easy-to-use and free code editor

SublimeText3 Chinese version
Chinese version, very easy to use

Zend Studio 13.0.1
Powerful PHP integrated development environment

Dreamweaver CS6
Visual web development tools

SublimeText3 Mac version
God-level code editing software (SublimeText3)

Hot Topics


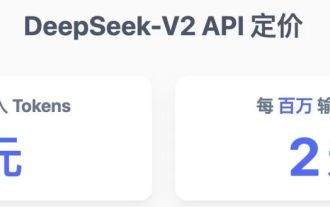
Imagine an artificial intelligence model that not only has the ability to surpass traditional computing, but also achieves more efficient performance at a lower cost. This is not science fiction, DeepSeek-V2[1], the world’s most powerful open source MoE model is here. DeepSeek-V2 is a powerful mixture of experts (MoE) language model with the characteristics of economical training and efficient inference. It consists of 236B parameters, 21B of which are used to activate each marker. Compared with DeepSeek67B, DeepSeek-V2 has stronger performance, while saving 42.5% of training costs, reducing KV cache by 93.3%, and increasing the maximum generation throughput to 5.76 times. DeepSeek is a company exploring general artificial intelligence
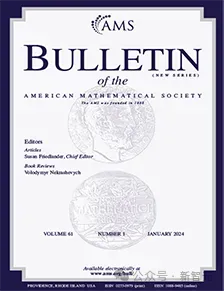
AI is indeed changing mathematics. Recently, Tao Zhexuan, who has been paying close attention to this issue, forwarded the latest issue of "Bulletin of the American Mathematical Society" (Bulletin of the American Mathematical Society). Focusing on the topic "Will machines change mathematics?", many mathematicians expressed their opinions. The whole process was full of sparks, hardcore and exciting. The author has a strong lineup, including Fields Medal winner Akshay Venkatesh, Chinese mathematician Zheng Lejun, NYU computer scientist Ernest Davis and many other well-known scholars in the industry. The world of AI has changed dramatically. You know, many of these articles were submitted a year ago.
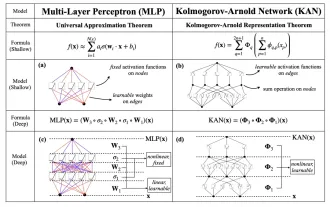
Earlier this month, researchers from MIT and other institutions proposed a very promising alternative to MLP - KAN. KAN outperforms MLP in terms of accuracy and interpretability. And it can outperform MLP running with a larger number of parameters with a very small number of parameters. For example, the authors stated that they used KAN to reproduce DeepMind's results with a smaller network and a higher degree of automation. Specifically, DeepMind's MLP has about 300,000 parameters, while KAN only has about 200 parameters. KAN has a strong mathematical foundation like MLP. MLP is based on the universal approximation theorem, while KAN is based on the Kolmogorov-Arnold representation theorem. As shown in the figure below, KAN has
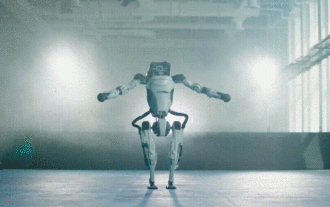
Boston Dynamics Atlas officially enters the era of electric robots! Yesterday, the hydraulic Atlas just "tearfully" withdrew from the stage of history. Today, Boston Dynamics announced that the electric Atlas is on the job. It seems that in the field of commercial humanoid robots, Boston Dynamics is determined to compete with Tesla. After the new video was released, it had already been viewed by more than one million people in just ten hours. The old people leave and new roles appear. This is a historical necessity. There is no doubt that this year is the explosive year of humanoid robots. Netizens commented: The advancement of robots has made this year's opening ceremony look like a human, and the degree of freedom is far greater than that of humans. But is this really not a horror movie? At the beginning of the video, Atlas is lying calmly on the ground, seemingly on his back. What follows is jaw-dropping
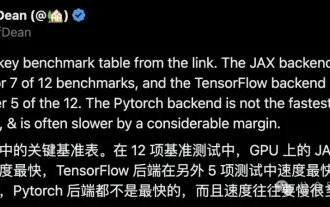
The performance of JAX, promoted by Google, has surpassed that of Pytorch and TensorFlow in recent benchmark tests, ranking first in 7 indicators. And the test was not done on the TPU with the best JAX performance. Although among developers, Pytorch is still more popular than Tensorflow. But in the future, perhaps more large models will be trained and run based on the JAX platform. Models Recently, the Keras team benchmarked three backends (TensorFlow, JAX, PyTorch) with the native PyTorch implementation and Keras2 with TensorFlow. First, they select a set of mainstream
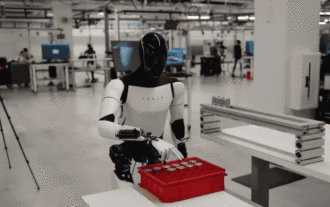
The latest video of Tesla's robot Optimus is released, and it can already work in the factory. At normal speed, it sorts batteries (Tesla's 4680 batteries) like this: The official also released what it looks like at 20x speed - on a small "workstation", picking and picking and picking: This time it is released One of the highlights of the video is that Optimus completes this work in the factory, completely autonomously, without human intervention throughout the process. And from the perspective of Optimus, it can also pick up and place the crooked battery, focusing on automatic error correction: Regarding Optimus's hand, NVIDIA scientist Jim Fan gave a high evaluation: Optimus's hand is the world's five-fingered robot. One of the most dexterous. Its hands are not only tactile
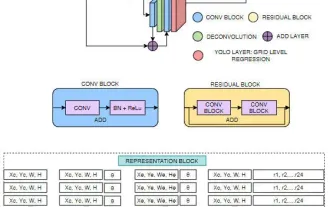
Target detection is a relatively mature problem in autonomous driving systems, among which pedestrian detection is one of the earliest algorithms to be deployed. Very comprehensive research has been carried out in most papers. However, distance perception using fisheye cameras for surround view is relatively less studied. Due to large radial distortion, standard bounding box representation is difficult to implement in fisheye cameras. To alleviate the above description, we explore extended bounding box, ellipse, and general polygon designs into polar/angular representations and define an instance segmentation mIOU metric to analyze these representations. The proposed model fisheyeDetNet with polygonal shape outperforms other models and simultaneously achieves 49.5% mAP on the Valeo fisheye camera dataset for autonomous driving
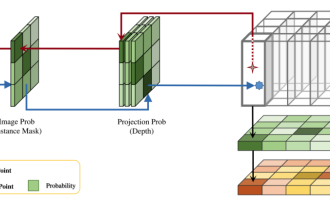
This paper explores the problem of accurately detecting objects from different viewing angles (such as perspective and bird's-eye view) in autonomous driving, especially how to effectively transform features from perspective (PV) to bird's-eye view (BEV) space. Transformation is implemented via the Visual Transformation (VT) module. Existing methods are broadly divided into two strategies: 2D to 3D and 3D to 2D conversion. 2D-to-3D methods improve dense 2D features by predicting depth probabilities, but the inherent uncertainty of depth predictions, especially in distant regions, may introduce inaccuracies. While 3D to 2D methods usually use 3D queries to sample 2D features and learn the attention weights of the correspondence between 3D and 2D features through a Transformer, which increases the computational and deployment time.
