


Technical guide for implementing modular development of Python scripts in Linux systems
Technical Guide to Implementing Modular Development of Python Scripts in Linux Systems
Introduction:
Python is a high-level programming language that is easy to learn and powerful. It is widely used in development in different fields. In Linux systems, modular development of Python scripts can effectively improve the maintainability and reusability of code and reduce development and maintenance costs. This article will introduce technical guidelines on how to use Python to implement modular development in Linux systems, and provide specific code examples.
1. Overview of modular development
Modular development is to divide a large program into small, interdependent modules, and communicate and interact through the interfaces between modules. It allows developers to focus on the design and implementation of each module, improving the readability and maintainability of the code. For Python scripts, a module can be a function, a class, or an independent script file.
2. Create a module
- Create an independent module file
In the Linux system, we can define a module by creating an independent Python script file. For example, we create a file named "utils.py", which defines some commonly used tool functions, as shown below:
# utils.py def add(a, b): return a + b def subtract(a, b): return a - b def multiply(a, b): return a * b def divide(a, b): return a / b
- Import module
In other Python scripts , we can use theimport
keyword to import already defined modules for use in the current script. For example, we create a script named "main.py". If we want to use the functions defined in "utils.py", we can import and call them in the following way:
# main.py import utils print(utils.add(1, 2)) # 输出:3 print(utils.subtract(5, 2)) # 输出:3 print(utils.multiply(2, 3)) # 输出:6 print(utils.divide(10, 2)) # 输出:5
- module The data is encapsulated
In the module, in addition to functions, we can also define variables and classes and access them through the module. This helps encapsulate related data and behavior in a module. For example, we define a constant and a class in "utils.py":
# utils.py PI = 3.1415926 class Circle: def __init__(self, radius): self.radius = radius def area(self): return PI * self.radius ** 2 def circumference(self): return 2 * PI * self.radius
It can be used in other scripts as follows:
# main.py import utils print(utils.PI) # 输出:3.1415926 circle = utils.Circle(5) print(circle.area()) # 输出:78.5398185 print(circle.circumference()) # 输出:31.415926
3. Module file organization
For large projects, one module may not be enough. We can organize related modules in the same directory and provide an entry file. For example, we create a directory called "calculator" and place "utils.py" and "main.py" in it.
# calculator/utils.py def add(a, b): return a + b def subtract(a, b): return a - b ... # calculator/main.py import utils ...
In the Linux system, you can run the "main.py" script in the following way:
$ python3 calculator/main.py
4. Module testing
During the development process, we often need to test the module test. Python provides several frameworks and tools for unit testing. For example, we can write test cases using the unittest
library and add a if __name__ == "__main__":
condition in the module to execute the test when the module is run directly.
# utils.py ... def divide(a, b): if b == 0: raise ValueError("除数不能为0") return a / b if __name__ == "__main__": import unittest class UtilsTestCase(unittest.TestCase): def test_add(self): self.assertEqual(add(1, 2), 3) def test_subtract(self): self.assertEqual(subtract(5, 2), 3) def test_multiply(self): self.assertEqual(multiply(2, 3), 6) def test_divide(self): self.assertEqual(divide(10, 2), 5) self.assertRaises(ValueError, divide, 10, 0) unittest.main()
In Linux systems, we can run tests in the following ways:
$ python3 utils.py
Summary:
Using Python scripts for modular development in Linux systems can improve the maintainability of the code and reusability. This article introduces the creation of modules, importing modules, module data encapsulation, module file organization and module testing. Through continuous practice and practice, developers can become more proficient in using Python scripts for modular development, improving development efficiency and code quality.
Reference materials:
- Python official documentation: https://www.python.org/
- Python unittest documentation: https://docs.python. org/3/library/unittest.html
The above is the detailed content of Technical guide for implementing modular development of Python scripts in Linux systems. For more information, please follow other related articles on the PHP Chinese website!

Hot AI Tools

Undresser.AI Undress
AI-powered app for creating realistic nude photos

AI Clothes Remover
Online AI tool for removing clothes from photos.

Undress AI Tool
Undress images for free

Clothoff.io
AI clothes remover

AI Hentai Generator
Generate AI Hentai for free.

Hot Article

Hot Tools

Notepad++7.3.1
Easy-to-use and free code editor

SublimeText3 Chinese version
Chinese version, very easy to use

Zend Studio 13.0.1
Powerful PHP integrated development environment

Dreamweaver CS6
Visual web development tools

SublimeText3 Mac version
God-level code editing software (SublimeText3)

Hot Topics


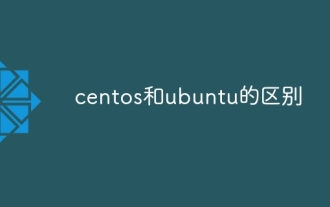
The key differences between CentOS and Ubuntu are: origin (CentOS originates from Red Hat, for enterprises; Ubuntu originates from Debian, for individuals), package management (CentOS uses yum, focusing on stability; Ubuntu uses apt, for high update frequency), support cycle (CentOS provides 10 years of support, Ubuntu provides 5 years of LTS support), community support (CentOS focuses on stability, Ubuntu provides a wide range of tutorials and documents), uses (CentOS is biased towards servers, Ubuntu is suitable for servers and desktops), other differences include installation simplicity (CentOS is thin)
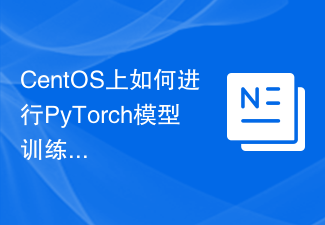
Efficient training of PyTorch models on CentOS systems requires steps, and this article will provide detailed guides. 1. Environment preparation: Python and dependency installation: CentOS system usually preinstalls Python, but the version may be older. It is recommended to use yum or dnf to install Python 3 and upgrade pip: sudoyumupdatepython3 (or sudodnfupdatepython3), pip3install--upgradepip. CUDA and cuDNN (GPU acceleration): If you use NVIDIAGPU, you need to install CUDATool
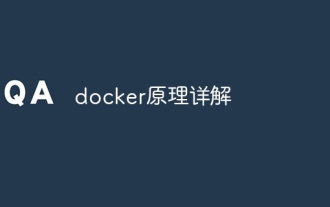
Docker uses Linux kernel features to provide an efficient and isolated application running environment. Its working principle is as follows: 1. The mirror is used as a read-only template, which contains everything you need to run the application; 2. The Union File System (UnionFS) stacks multiple file systems, only storing the differences, saving space and speeding up; 3. The daemon manages the mirrors and containers, and the client uses them for interaction; 4. Namespaces and cgroups implement container isolation and resource limitations; 5. Multiple network modes support container interconnection. Only by understanding these core concepts can you better utilize Docker.
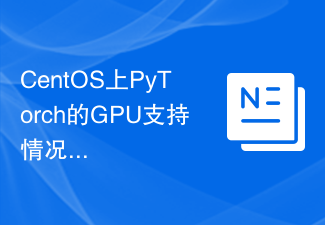
Enable PyTorch GPU acceleration on CentOS system requires the installation of CUDA, cuDNN and GPU versions of PyTorch. The following steps will guide you through the process: CUDA and cuDNN installation determine CUDA version compatibility: Use the nvidia-smi command to view the CUDA version supported by your NVIDIA graphics card. For example, your MX450 graphics card may support CUDA11.1 or higher. Download and install CUDAToolkit: Visit the official website of NVIDIACUDAToolkit and download and install the corresponding version according to the highest CUDA version supported by your graphics card. Install cuDNN library:
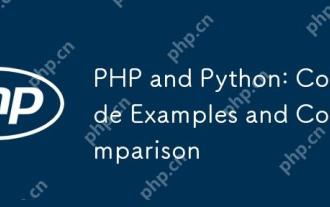
PHP and Python have their own advantages and disadvantages, and the choice depends on project needs and personal preferences. 1.PHP is suitable for rapid development and maintenance of large-scale web applications. 2. Python dominates the field of data science and machine learning.
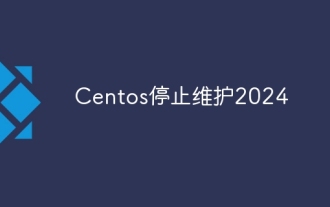
CentOS will be shut down in 2024 because its upstream distribution, RHEL 8, has been shut down. This shutdown will affect the CentOS 8 system, preventing it from continuing to receive updates. Users should plan for migration, and recommended options include CentOS Stream, AlmaLinux, and Rocky Linux to keep the system safe and stable.
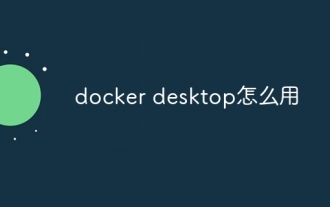
How to use Docker Desktop? Docker Desktop is a tool for running Docker containers on local machines. The steps to use include: 1. Install Docker Desktop; 2. Start Docker Desktop; 3. Create Docker image (using Dockerfile); 4. Build Docker image (using docker build); 5. Run Docker container (using docker run).
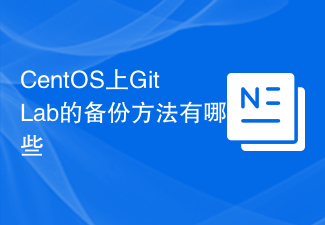
Backup and Recovery Policy of GitLab under CentOS System In order to ensure data security and recoverability, GitLab on CentOS provides a variety of backup methods. This article will introduce several common backup methods, configuration parameters and recovery processes in detail to help you establish a complete GitLab backup and recovery strategy. 1. Manual backup Use the gitlab-rakegitlab:backup:create command to execute manual backup. This command backs up key information such as GitLab repository, database, users, user groups, keys, and permissions. The default backup file is stored in the /var/opt/gitlab/backups directory. You can modify /etc/gitlab
