


Far ahead! BEVHeight++: A new solution for roadside visual 3D target detection!
Regress to the height of the ground to achieve a distance-agnostic formulation, thereby simplifying the optimization process for camera-aware only methods. On the 3D detection benchmark of roadside cameras, the method greatly exceeds all previous vision-centric methods. It yields significant improvements over BEVDepth of 1.9% NDS and 1.1% mAP. On the nuScenes test set, the method has made substantial progress, with NDS and mAP increasing by 2.8% and 1.7% respectively.
Title: BEVHeight: Towards robust vision-centered 3D object detection
Paper link: https://arxiv.org/pdf/2309.16179.pdf
Author Unit: Tsinghua University, Sun Yat-sen University, Cainiao Network, Peking University
From the first domestic autonomous driving community: finally completed the construction of 20 technical direction learning routes (BEV sensing/3D detection/multi-sensor fusion /SLAM and planning, etc.)
Although recent autonomous driving systems have focused on developing perception methods for vehicle sensors, people have often overlooked a method that uses smart roadside cameras to extend perception capabilities beyond the visual range. alternative methods. The authors found that state-of-the-art vision-centric BEV detection methods perform poorly on roadside cameras. This is because these methods mainly focus on recovering the depth about the camera center, where the depth difference between the car and the ground shrinks rapidly with distance. In this article, the author proposes a simple yet effective method, called BEVHeight, to solve this problem. Essentially, the authors regress to the height of the ground to achieve a distance-agnostic formulation, thereby simplifying the optimization process for camera-aware-only methods. By combining height and depth encoding techniques, a more accurate and robust projection from 2D to BEV space is achieved. The method significantly outperforms all previous vision-centric methods on the popular 3D detection benchmark of roadside cameras. For self-vehicle scenes, BEVHeight outperforms depth-only methods
Specifically, it yields 1.9% better NDS and 1.1% better mAP than BEVDepth when evaluated on the nuScenes validation set. To improve. In addition, on the nuScenes test set, the method achieved substantial progress, with NDS and mAP increasing by 2.8% and 1.7% respectively.
Figure 1: (a) To generate 3D bounding boxes from monocular images, state-of-the-art methods first explicitly or implicitly predict per-pixel depth to determine The 3D position of the foreground object versus the background. However, when we plotted the per-pixel depth on the image, we noticed that the difference between points on the roof and the surrounding ground quickly shrinks as the car moves away from the camera, making the optimization sub-optimal, especially for Distant objects. (b) Instead, we plot the per-pixel height to the ground and observe that this difference is agnostic regardless of distance and is visually more suitable for the network to detect objects. However, 3D position cannot be directly regressed by predicting height alone. (c) To this end, we propose a new framework BEVHeight to solve this problem. Empirical results show that our method outperforms the best method by 5.49% on clean settings and 28.2% on noisy settings.
Network structure
Comparison of prediction height and depth. (a) Overview of previous depth-based methods and our proposed height-based pipeline. Please note that this paper proposes a novel 2D to 3D projection module. (b) Plotting histograms of per-pixel depth (top) and ground height (bottom), it can be clearly observed that the depth range is over 200 meters, while the height is within 5 meters, which makes the height easier to learn.
In an image, there is a correlation between the row coordinates of an object and its depth and height. The position of the target in the image can be defined by (u, v), where v represents the row coordinate of the image. In (a) we show a visual example of introducing noise by adding rotational offsets in the roll and pitch directions to a normal distribution. In (b) we show a scatterplot of the depth distribution. In (c) we show the height above the ground. We can observe that the noise setting for height has a greater overlap with its original distribution compared to depth, indicating that height estimation is more robust
The overall framework of BEVHeight contains three sub-networks, namely the depth-based branch (cyan), the height-based branch (green) and the feature fusion process (grey). The depth-based pipeline converts image view features into depth-based BEV features (D-based BEV) using the estimated per-pixel depth. The height-based pipeline generates height-based BEV features (H-based BEV) using ground height predictions of lift features in image views. Feature fusion includes image fusion and bird's-eye view fusion. Image-view fusion obtains fusion features by cascading height distribution and image features, which are used for subsequent upgrading operations. Bird's-eye view fusion obtains fused BEV features from height-based BEV features and depth-based BEV features through deformable cross-attention, and then uses it as the input of the detection head
Experimental results
The above is the detailed content of Far ahead! BEVHeight++: A new solution for roadside visual 3D target detection!. For more information, please follow other related articles on the PHP Chinese website!

Hot AI Tools

Undresser.AI Undress
AI-powered app for creating realistic nude photos

AI Clothes Remover
Online AI tool for removing clothes from photos.

Undress AI Tool
Undress images for free

Clothoff.io
AI clothes remover

AI Hentai Generator
Generate AI Hentai for free.

Hot Article

Hot Tools

Notepad++7.3.1
Easy-to-use and free code editor

SublimeText3 Chinese version
Chinese version, very easy to use

Zend Studio 13.0.1
Powerful PHP integrated development environment

Dreamweaver CS6
Visual web development tools

SublimeText3 Mac version
God-level code editing software (SublimeText3)

Hot Topics
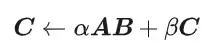
General Matrix Multiplication (GEMM) is a vital part of many applications and algorithms, and is also one of the important indicators for evaluating computer hardware performance. In-depth research and optimization of the implementation of GEMM can help us better understand high-performance computing and the relationship between software and hardware systems. In computer science, effective optimization of GEMM can increase computing speed and save resources, which is crucial to improving the overall performance of a computer system. An in-depth understanding of the working principle and optimization method of GEMM will help us better utilize the potential of modern computing hardware and provide more efficient solutions for various complex computing tasks. By optimizing the performance of GEMM
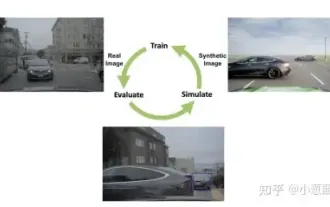
Yesterday during the interview, I was asked whether I had done any long-tail related questions, so I thought I would give a brief summary. The long-tail problem of autonomous driving refers to edge cases in autonomous vehicles, that is, possible scenarios with a low probability of occurrence. The perceived long-tail problem is one of the main reasons currently limiting the operational design domain of single-vehicle intelligent autonomous vehicles. The underlying architecture and most technical issues of autonomous driving have been solved, and the remaining 5% of long-tail problems have gradually become the key to restricting the development of autonomous driving. These problems include a variety of fragmented scenarios, extreme situations, and unpredictable human behavior. The "long tail" of edge scenarios in autonomous driving refers to edge cases in autonomous vehicles (AVs). Edge cases are possible scenarios with a low probability of occurrence. these rare events
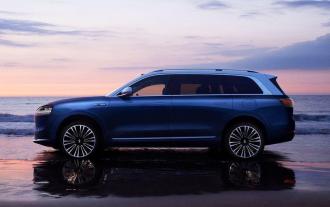
On July 29, at the roll-off ceremony of AITO Wenjie's 400,000th new car, Yu Chengdong, Huawei's Managing Director, Chairman of Terminal BG, and Chairman of Smart Car Solutions BU, attended and delivered a speech and announced that Wenjie series models will be launched this year In August, Huawei Qiankun ADS 3.0 version was launched, and it is planned to successively push upgrades from August to September. The Xiangjie S9, which will be released on August 6, will debut Huawei’s ADS3.0 intelligent driving system. With the assistance of lidar, Huawei Qiankun ADS3.0 version will greatly improve its intelligent driving capabilities, have end-to-end integrated capabilities, and adopt a new end-to-end architecture of GOD (general obstacle identification)/PDP (predictive decision-making and control) , providing the NCA function of smart driving from parking space to parking space, and upgrading CAS3.0
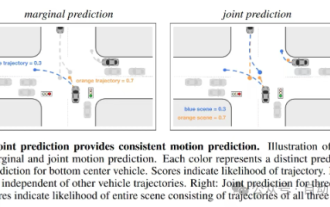
Trajectory prediction plays an important role in autonomous driving. Autonomous driving trajectory prediction refers to predicting the future driving trajectory of the vehicle by analyzing various data during the vehicle's driving process. As the core module of autonomous driving, the quality of trajectory prediction is crucial to downstream planning control. The trajectory prediction task has a rich technology stack and requires familiarity with autonomous driving dynamic/static perception, high-precision maps, lane lines, neural network architecture (CNN&GNN&Transformer) skills, etc. It is very difficult to get started! Many fans hope to get started with trajectory prediction as soon as possible and avoid pitfalls. Today I will take stock of some common problems and introductory learning methods for trajectory prediction! Introductory related knowledge 1. Are the preview papers in order? A: Look at the survey first, p

Original title: SIMPL: ASimpleandEfficientMulti-agentMotionPredictionBaselineforAutonomousDriving Paper link: https://arxiv.org/pdf/2402.02519.pdf Code link: https://github.com/HKUST-Aerial-Robotics/SIMPL Author unit: Hong Kong University of Science and Technology DJI Paper idea: This paper proposes a simple and efficient motion prediction baseline (SIMPL) for autonomous vehicles. Compared with traditional agent-cent
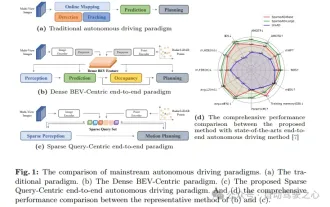
Written in front & starting point The end-to-end paradigm uses a unified framework to achieve multi-tasking in autonomous driving systems. Despite the simplicity and clarity of this paradigm, the performance of end-to-end autonomous driving methods on subtasks still lags far behind single-task methods. At the same time, the dense bird's-eye view (BEV) features widely used in previous end-to-end methods make it difficult to scale to more modalities or tasks. A sparse search-centric end-to-end autonomous driving paradigm (SparseAD) is proposed here, in which sparse search fully represents the entire driving scenario, including space, time, and tasks, without any dense BEV representation. Specifically, a unified sparse architecture is designed for task awareness including detection, tracking, and online mapping. In addition, heavy
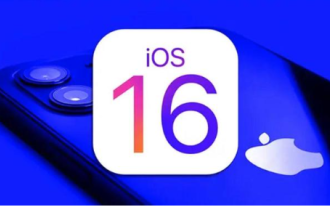
The best version of the Apple 16 system is iOS16.1.4. The best version of the iOS16 system may vary from person to person. The additions and improvements in daily use experience have also been praised by many users. Which version of the Apple 16 system is the best? Answer: iOS16.1.4 The best version of the iOS 16 system may vary from person to person. According to public information, iOS16, launched in 2022, is considered a very stable and performant version, and users are quite satisfied with its overall experience. In addition, the addition of new features and improvements in daily use experience in iOS16 have also been well received by many users. Especially in terms of updated battery life, signal performance and heating control, user feedback has been relatively positive. However, considering iPhone14
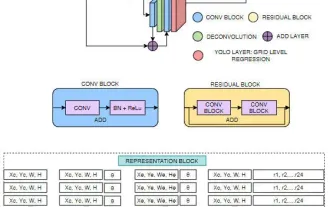
Target detection is a relatively mature problem in autonomous driving systems, among which pedestrian detection is one of the earliest algorithms to be deployed. Very comprehensive research has been carried out in most papers. However, distance perception using fisheye cameras for surround view is relatively less studied. Due to large radial distortion, standard bounding box representation is difficult to implement in fisheye cameras. To alleviate the above description, we explore extended bounding box, ellipse, and general polygon designs into polar/angular representations and define an instance segmentation mIOU metric to analyze these representations. The proposed model fisheyeDetNet with polygonal shape outperforms other models and simultaneously achieves 49.5% mAP on the Valeo fisheye camera dataset for autonomous driving
