


With up to 4 million token contexts and 22 times faster inference, StreamingLLM has become popular and has received 2.5K stars on GitHub.
If you’ve ever interacted with any conversational AI bot, you’ll remember some very frustrating moments. For example, the important things you mentioned in the conversation the day before were completely forgotten by the AI...
This is because most current LLMs can only remember limited context. Like students who cram for exams, their flaws will be revealed after a little questioning.
What if the AI assistant could contextually reference conversations from weeks or months ago in a chat, or you could ask the AI assistant to summarize a report that was thousands of pages long, then something like this Is your ability enviable?
In order to make LLM better able to remember and remember more content, researchers have been working hard. Recently, researchers from MIT, Meta AI, and Carnegie Mellon University proposed a method called "StreamingLLM" that enables language models to smoothly process endless text
- ##Paper address: https://arxiv.org/pdf/2309.17453.pdf
- Project address: https://github.com/mit-han-lab/streaming-llm
StreamingLLM The working principle is to identify and save the initial tokens anchored by the model's inherent "attention sinks" for its reasoning. Combined with a rolling cache of recent tokens, StreamingLLM speeds up inference by 22x without sacrificing any accuracy. In just a few days, the project has gained 2.5K stars on the GitHub platform:
# Specifically, StreamingLLM is a A technology that enables language models to accurately remember the score of the last game, the name of a new baby, a lengthy contract, or the content of a debate. Just like upgrading the memory of the AI assistant, it can handle more heavy workloads perfectly
Let’s look at the technical details.
Method Innovation
Generally, LLM is limited by the attention window during pre-training. Although there has been a lot of previous work to expand this window size and improve training and inference efficiency, the acceptable sequence length of LLM is still limited, which is not friendly for persistent deployment.
In this paper, the researcher first introduced the concept of LLM stream application and raised a question: "Can it be used with infinite length without sacrificing efficiency and performance? Input deployment LLM?"
When applying LLM to an infinitely long input stream, you will face two main challenges:
1. When decoding stage, the transformer-based LLM will cache the Key and Value status (KV) of all previous tokens, as shown in Figure 1 (a), which may cause excessive memory usage and increase decoding delay;
2. The length extrapolation ability of existing models is limited, that is, when the sequence length exceeds the attention window size set during pre-training, its performance will decrease.
An intuitive method is called Window Attention (Figure 1 b). This method only focuses on the most recent token. Maintaining a fixed-size sliding window on the KV state, although it can ensure that stable memory usage and decoding speed can be maintained after the cache is filled, once the sequence length exceeds the cache size, even just the KV of the first token is evicted. The model will collapse. Another method is to recalculate the sliding window (shown in Figure 1 c). This method will reconstruct the KV state of the recent token for each generated token. Although the performance is powerful, it requires the calculation of secondary attention within the window. The result is significantly slower, which is not ideal in real streaming applications.
In the process of studying the failure of window attention, the researchers discovered an interesting phenomenon: according to Figure 2, a large number of attention scores are assigned to initial tags, regardless of whether these tags are related to language. Related to modeling tasks
Researchers call these tokens "attention pools": although they lack semantic meaning, but takes up a lot of attention points. The researchers attribute this phenomenon to Softmax (which requires the sum of the attention scores of all context tokens to be 1). Even if the current query does not have a strong match among many previous tokens, the model still needs to transfer these unnecessary attentions. Values are assigned somewhere so that they sum to 1. The reason why the initial token becomes a "pool" is intuitive: due to the characteristics of autoregressive language modeling, the initial token is visible to almost all subsequent tokens, which makes them easier to train as an attention pool.
Based on the above insights, the researcher proposed StreamingLLM. This is a simple yet efficient framework that allows attention models trained with limited attention windows to handle infinitely long text without fine-tuning
StreamingLLM leverages attention The fact that pools have high attention values, retaining these attention pools can make the attention score distribution close to a normal distribution. Therefore, StreamingLLM only needs to retain the KV value of the attention pool token (only 4 initial tokens are enough) and the KV value of the sliding window to anchor the attention calculation and stabilize the performance of the model.
Use StreamingLLM, including Llama-2-[7,13,70] B, MPT-[7,30] B, Falcon-[7,40] B and Pythia [2.9 ,6.9,12] Models including B can reliably simulate 4 million tokens or even more.
Compared to recalculating the sliding window, StreamingLLM is 22.2 times faster without any performance penalty
Evaluation
In experiments, as shown in Figure 3, for text spanning 20K tokens, StreamingLLM’s perplexity is comparable to the Oracle baseline of recomputing the sliding window. At the same time, when the input length exceeds the pre-training window, dense attention fails, and when the input length exceeds the cache size, window attention gets stuck, causing the initial tags to be eliminated
Figure 5 further confirms the reliability of StreamingLLM, which can handle unconventional scale text, including more than 4 million tokens, covering various model families and sizes. These models include Llama-2-[7,13,70] B, Falcon-[7,40] B, Pythia-[2.8,6.9,12] B and MPT-[7,30] B
Subsequently, the researchers confirmed the hypothesis of "attention pool" and proved that the language model can be pre-trained and only requires one attention pool token during streaming deployment. Specifically, they suggest adding an additional learnable token at the beginning of all training samples as a designated attention pool. By pre-training a language model with 160 million parameters from scratch, the researchers demonstrated that our method can maintain the performance of the model. This is in sharp contrast to current language models, which require the reintroduction of multiple initial tokens as attention pools to achieve the same level of performance.
Finally, the researchers compared the decoding latency and memory usage of StreamingLLM with the recalculated sliding window, using Llama-2-7B and Llama- on a single NVIDIA A6000 GPU. 2-13B model was tested. According to the results in Figure 10, as the cache size increases, the decoding speed of StreamingLLM increases linearly, while the decoding delay increases quadratically. Experiments have proven that StreamingLLM achieves impressive speed-up, with the speed of each token increased by up to 22.2 times
More For more research details, please refer to the original paper.
The above is the detailed content of With up to 4 million token contexts and 22 times faster inference, StreamingLLM has become popular and has received 2.5K stars on GitHub.. For more information, please follow other related articles on the PHP Chinese website!

Hot AI Tools

Undresser.AI Undress
AI-powered app for creating realistic nude photos

AI Clothes Remover
Online AI tool for removing clothes from photos.

Undress AI Tool
Undress images for free

Clothoff.io
AI clothes remover

AI Hentai Generator
Generate AI Hentai for free.

Hot Article

Hot Tools

Notepad++7.3.1
Easy-to-use and free code editor

SublimeText3 Chinese version
Chinese version, very easy to use

Zend Studio 13.0.1
Powerful PHP integrated development environment

Dreamweaver CS6
Visual web development tools

SublimeText3 Mac version
God-level code editing software (SublimeText3)

Hot Topics


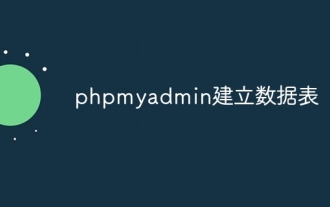
To create a data table using phpMyAdmin, the following steps are essential: Connect to the database and click the New tab. Name the table and select the storage engine (InnoDB recommended). Add column details by clicking the Add Column button, including column name, data type, whether to allow null values, and other properties. Select one or more columns as primary keys. Click the Save button to create tables and columns.
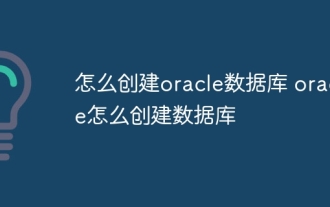
Creating an Oracle database is not easy, you need to understand the underlying mechanism. 1. You need to understand the concepts of database and Oracle DBMS; 2. Master the core concepts such as SID, CDB (container database), PDB (pluggable database); 3. Use SQL*Plus to create CDB, and then create PDB, you need to specify parameters such as size, number of data files, and paths; 4. Advanced applications need to adjust the character set, memory and other parameters, and perform performance tuning; 5. Pay attention to disk space, permissions and parameter settings, and continuously monitor and optimize database performance. Only by mastering it skillfully requires continuous practice can you truly understand the creation and management of Oracle databases.
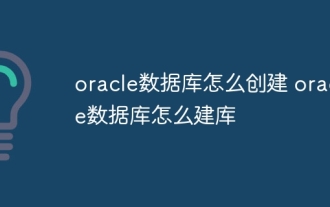
To create an Oracle database, the common method is to use the dbca graphical tool. The steps are as follows: 1. Use the dbca tool to set the dbName to specify the database name; 2. Set sysPassword and systemPassword to strong passwords; 3. Set characterSet and nationalCharacterSet to AL32UTF8; 4. Set memorySize and tablespaceSize to adjust according to actual needs; 5. Specify the logFile path. Advanced methods are created manually using SQL commands, but are more complex and prone to errors. Pay attention to password strength, character set selection, tablespace size and memory
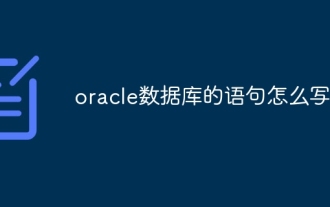
The core of Oracle SQL statements is SELECT, INSERT, UPDATE and DELETE, as well as the flexible application of various clauses. It is crucial to understand the execution mechanism behind the statement, such as index optimization. Advanced usages include subqueries, connection queries, analysis functions, and PL/SQL. Common errors include syntax errors, performance issues, and data consistency issues. Performance optimization best practices involve using appropriate indexes, avoiding SELECT *, optimizing WHERE clauses, and using bound variables. Mastering Oracle SQL requires practice, including code writing, debugging, thinking and understanding the underlying mechanisms.
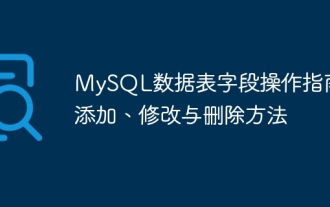
Field operation guide in MySQL: Add, modify, and delete fields. Add field: ALTER TABLE table_name ADD column_name data_type [NOT NULL] [DEFAULT default_value] [PRIMARY KEY] [AUTO_INCREMENT] Modify field: ALTER TABLE table_name MODIFY column_name data_type [NOT NULL] [DEFAULT default_value] [PRIMARY KEY]
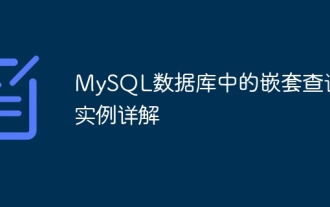
Nested queries are a way to include another query in one query. They are mainly used to retrieve data that meets complex conditions, associate multiple tables, and calculate summary values or statistical information. Examples include finding employees above average wages, finding orders for a specific category, and calculating the total order volume for each product. When writing nested queries, you need to follow: write subqueries, write their results to outer queries (referenced with alias or AS clauses), and optimize query performance (using indexes).
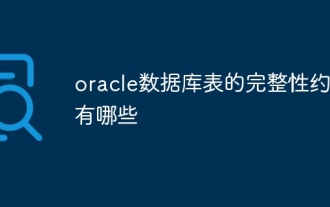
The integrity constraints of Oracle databases can ensure data accuracy, including: NOT NULL: null values are prohibited; UNIQUE: guarantee uniqueness, allowing a single NULL value; PRIMARY KEY: primary key constraint, strengthen UNIQUE, and prohibit NULL values; FOREIGN KEY: maintain relationships between tables, foreign keys refer to primary table primary keys; CHECK: limit column values according to conditions.
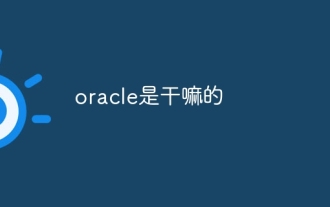
Oracle is the world's largest database management system (DBMS) software company. Its main products include the following functions: relational database management system (Oracle database) development tools (Oracle APEX, Oracle Visual Builder) middleware (Oracle WebLogic Server, Oracle SOA Suite) cloud service (Oracle Cloud Infrastructure) analysis and business intelligence (Oracle Analytics Cloud, Oracle Essbase) blockchain (Oracle Blockchain Pla
