Natural language understanding problems in dialogue systems
Natural language understanding problems in dialogue systems require specific code examples
With the continuous development of artificial intelligence technology, dialogue systems have become an increasingly important part of people’s daily lives. important part. However, building an efficient and accurate dialogue system is not easy. One of the key issues is how to achieve natural language understanding.
Natural Language Understanding (NLU) refers to the process of computer analysis and understanding of human language. In a dialogue system, the main task of NLU is to transform the user's input into a form that the computer can understand and process, so that the dialogue system can correctly understand the user's intentions and needs and make the correct response.
In the process of realizing natural language understanding, Natural Language Processing (NLP) technology is often used. NLP technology identifies the structure, grammar, semantics and other information of sentences through the analysis and processing of text, thereby realizing the understanding and processing of text. In dialogue systems, NLP technology can help the system understand the commands, questions, intentions, etc. entered by users.
The following is a simple code example that shows how to use the nltk library in Python to implement word segmentation and part-of-speech tagging for user input:
import nltk from nltk.tokenize import word_tokenize from nltk.tag import pos_tag def nlu(text): # 分词 tokens = word_tokenize(text) # 词性标注 tags = pos_tag(tokens) return tags # 用户输入的文本 input_text = "请帮我订一张明天早上九点的机票。" # 调用NLU函数进行处理 result = nlu(input_text) print(result)
In the above code, first import Use the nltk library, and then use the word_tokenize function to segment the text entered by the user into words to obtain a word list. Then, use the pos_tag function to perform part-of-speech tagging on the word segmentation results to obtain the part-of-speech of each word. Finally, print the results.
For example, for the input text "Please book a ticket for me at nine o'clock tomorrow morning.", the output result is as follows:
[('请', 'NN'), ('帮', 'VV'), ('我', 'PN'), ('订', 'VV'), ('一', 'CD'), ('张', 'M'), ('明天', 'NT'), ('早上', 'NT'), ('九点', 'NT'), ('的', 'DEC'), ('机票', 'NN'), ('。', 'PU')]
As can be seen from the output result, each word is marked with a part of speech. For example: "please" is marked as a noun (NN), "help" is marked as a verb (VV), and so on.
This simple code example shows how to use the nltk library to implement word segmentation and part-of-speech tagging of user input, which is an important part of achieving natural language understanding. Of course, for a complete dialogue system, more NLP technologies and algorithms are needed, such as named entity recognition, syntactic analysis, semantic analysis, etc., to achieve more complex and accurate natural language understanding capabilities.
To sum up, the problem of natural language understanding in dialogue systems is a critical and complex task. By making full use of natural language processing technology, combined with appropriate algorithms and models, we can achieve accurate understanding of user input and provide better intelligent interaction capabilities for dialogue systems.
The above is the detailed content of Natural language understanding problems in dialogue systems. For more information, please follow other related articles on the PHP Chinese website!

Hot AI Tools

Undresser.AI Undress
AI-powered app for creating realistic nude photos

AI Clothes Remover
Online AI tool for removing clothes from photos.

Undress AI Tool
Undress images for free

Clothoff.io
AI clothes remover

Video Face Swap
Swap faces in any video effortlessly with our completely free AI face swap tool!

Hot Article

Hot Tools

Notepad++7.3.1
Easy-to-use and free code editor

SublimeText3 Chinese version
Chinese version, very easy to use

Zend Studio 13.0.1
Powerful PHP integrated development environment

Dreamweaver CS6
Visual web development tools

SublimeText3 Mac version
God-level code editing software (SublimeText3)

Hot Topics


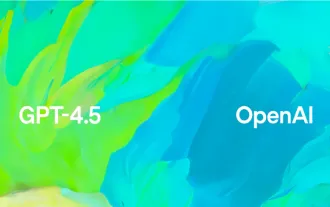
February 2025 has been yet another game-changing month for generative AI, bringing us some of the most anticipated model upgrades and groundbreaking new features. From xAI’s Grok 3 and Anthropic’s Claude 3.7 Sonnet, to OpenAI’s G
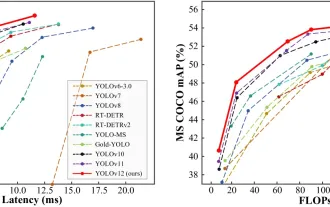
YOLO (You Only Look Once) has been a leading real-time object detection framework, with each iteration improving upon the previous versions. The latest version YOLO v12 introduces advancements that significantly enhance accuracy
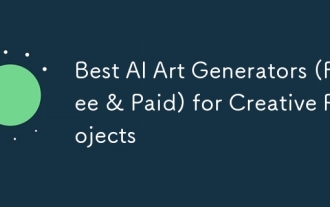
The article reviews top AI art generators, discussing their features, suitability for creative projects, and value. It highlights Midjourney as the best value for professionals and recommends DALL-E 2 for high-quality, customizable art.
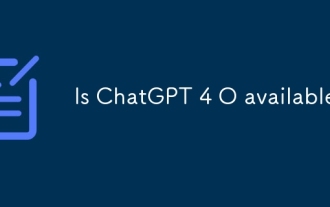
ChatGPT 4 is currently available and widely used, demonstrating significant improvements in understanding context and generating coherent responses compared to its predecessors like ChatGPT 3.5. Future developments may include more personalized interactions and real-time data processing capabilities, further enhancing its potential for various applications.
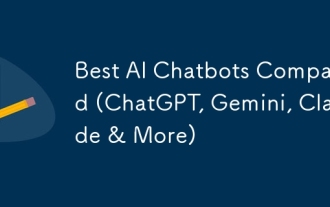
The article compares top AI chatbots like ChatGPT, Gemini, and Claude, focusing on their unique features, customization options, and performance in natural language processing and reliability.
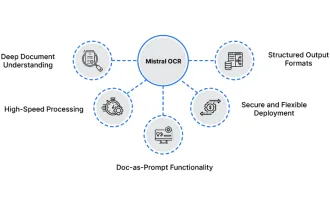
Mistral OCR: Revolutionizing Retrieval-Augmented Generation with Multimodal Document Understanding Retrieval-Augmented Generation (RAG) systems have significantly advanced AI capabilities, enabling access to vast data stores for more informed respons
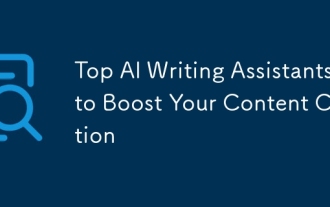
The article discusses top AI writing assistants like Grammarly, Jasper, Copy.ai, Writesonic, and Rytr, focusing on their unique features for content creation. It argues that Jasper excels in SEO optimization, while AI tools help maintain tone consist
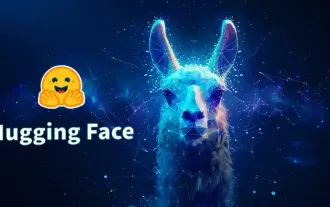
Meta's Llama 3.2: A Leap Forward in Multimodal and Mobile AI Meta recently unveiled Llama 3.2, a significant advancement in AI featuring powerful vision capabilities and lightweight text models optimized for mobile devices. Building on the success o
